Available PhD research topics
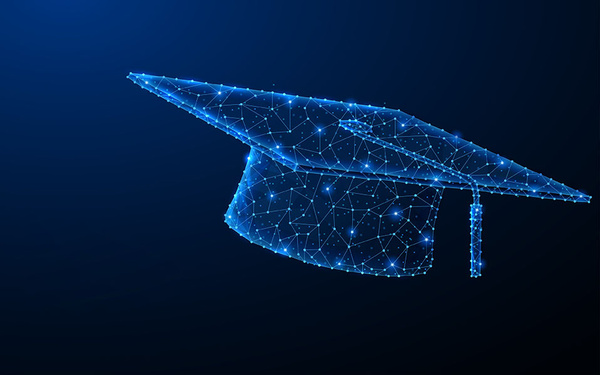
Based on the overarching research foci of BIFOLD, the BIFOLD Graduate School is offering new PhD projects in the areas of current challenges in artificial intelligence (AI) and data science (DS), with focus on data management, machine learning, and their intersection.
Below is a brief description of the current research pursued by the BIFOLD research groups, including short lists of their main topics and foci. For more details, we recommend that you look at the respective webpages of the group leads.
Contact:
Please feel free to reach out to the group leads directly or to: gsapplication@bifold.tu-berlin.de – depending of the nature of your query.
BIFOLD Research Groups and Their Topics
The Distinguished Research Group of Volker Markl works on a wide range of topics and challenges in Database Systems and Information Management, with the overarching goal to address both the human and technical latencies prevalent in the data analysis process. The group investigates:
- Large-Scale Data Stream Processing
- Distributed Query Processing
- Adaptive Query Processing
- Query Compilation, Optimization and Hardware-tailored Code Generation
- Data Processing on Modern Hardware
- Data Storage and Transaction Processing
- Declarative Query Languages
The Distinguished Research Group led by Klaus-Robert Müller tackles problems within the bigger fields of Machine Learning and Intelligent Data Analysis, with the overarching goals to develop robust and interpretable ML methods for learning from complex structured and non-stationary data, and the fusion of heterogeneous multi-modal data sources. The group works on:
- Learning from Structured, Non-stationary and Multi-modal Data
- Incorporating Domain Knowledge and Symmetries in ML Models
- Robust Explainable AI for Structured, Heterogeneous Data
- Structured Anomaly Detection
- Robust Reinforcement Learning in Complex, Partially Observed State Spaces
- ML Applications in the Sciences
- Deep Learning and GANs
The Senior Research Group led by Begüm Demir works on Big Data Analytics for Earth Observation (EO) at the intersection of remote sensing, DM and ML. The group investigates and creates theoretical and methodological foundations of DM and ML for EO, with the goal to process and analyze a large amount of decentralized EO data in a scalable and privacy-aware manner and focuses on the following topics:
- Privacy-preserving Analysis of EO Data
- Continual Learning for Large-Scale EO Data Analysis
- Heterogeneous Multi-Source EO Data Analysis
- Uncertainty-Aware Analysis of Large-Scale EO Data
The Senior Research Group led by Matthias Boehm focuses on system-oriented research for simplifying the end-to-end data science lifecycle via high-level, data-science-centric abstractions as well as systems and tools to execute these tasks in an efficient and scalable manner:
- Data-centric ML Pipelines (data integration, cleaning, augmentation, alignment of multi-modal data)
- Compilation Techniques for Efficient and Scalable Model Training and Scoring
- Automated Data Reorganization, Sparsity and Redundancy Exploitation
- Data Platforms, Federated Learning, and Cloud Infrastructure
- Data and Model Debugging, Fairness and Robustness
The Senior Research Group led by Konrad Rieck conducts fundamental research at the intersection of computer security and machine learning. On the one end, the group develops intelligent systems that can learn to protect computers from attacks and identify security problems automatically. On the other end, it explores the security and privacy of machine learning and develops novel attacks and defenses.
- Intelligent detection and analysis of computer attacks
- Automatic discovery of security vulnerabilities
- Novel attacks and defenses for learning algorithms
- Trustworthy and privacy-friendly machine learning
The Junior Research Group led by Grégoire Montavon advances the foundations and algorithms of explainable AI (XAI) with a focus on deep neural networks. It develops novel XAI methods that can identify features that are relevant for prediction. Another focus of the group is on closing the gap between existing XAI methods and practical desiderata.
- Uncovering the neural network structure of ML models to improve their explainability
- Leveraging latent human-interpretable concepts in Explainable AI
- Explainable AI to build more trustworthy machine learning models
- Explainable AI to extract actionable insights from complex datasets
The Independent Research Group of Shinichi Nakajima focuses on probabilistic modelling and inference methods for multimodal, heterogeneous, and complex structured data analysis, providing ML tools that can incorporate multiple aspects of data samples observed under different circumstances, in efficient and theoretically grounded ways.
- Generative Models and Inference Methods
- Applications of Generative Models and Bayesian Inference Methods
- Practical Uncertainty Estimation Methods
The Independent Research Group “Intelligent Biomedical Sensing” led by Alexander von Lühmann develops miniaturized wearable neurotechnology, body-worn sensors and multimodal machine learning methods for continuous and unobtrusive sensing of the embodied brain in the everyday world. The group focuses on multimodal analysis of physiological signals from diffuse optics (e.g., Diffuse Optical Tomography - DOT / functional Near-Infrared Spectroscopy - fNIRS) and from bio-potentials (e.g., Electroencephalography - EEG).
- Multimodal wearable instruments for neuro-physiological imaging
- Machine learning for multimodal multivariate time series analysis, bio signal processing and physiological modelling
- Naturalistic experiments and monitoring, context sensitivity and automated labelling
The Research Training Group led by Steffen Zeuch works on developing a data management system for the processing of heterogeneous data streams in distributed fog and edge environments. The aim is to design a data management system that unifies cloud, fog, and sensor environments at an unprecedented scale. In particular, a system that can host these environments on a unified platform, and leverages the opportunities of the unified architecture for cross-paradigm data processing optimizations, to support emerging IoT applications. The NebulaStream (www.nebula.stream) project builds a novel open-source stream data management system, which combines the cloud, the fog, and the sensors in a single unified platform and provides a holistic view for processing distributed fast data.
- Data Processing on Modern Hardware
- Data Processing in a Fog/Cloud Environment
The Research Training Group led by Stefan Chmiela focuses on Machine Learning for many-body problems, with particular focus on quantum chemistry. The group develops methods that combine fundamental physical principles with statistical modeling approaches to overcome the combinatorial challenges that manifest themselves when large numbers of particles interact. Research is centered around topics such as
- graph neural networks
- large-scale kernel methods an
- the challenge of invariant/equivariant modelling