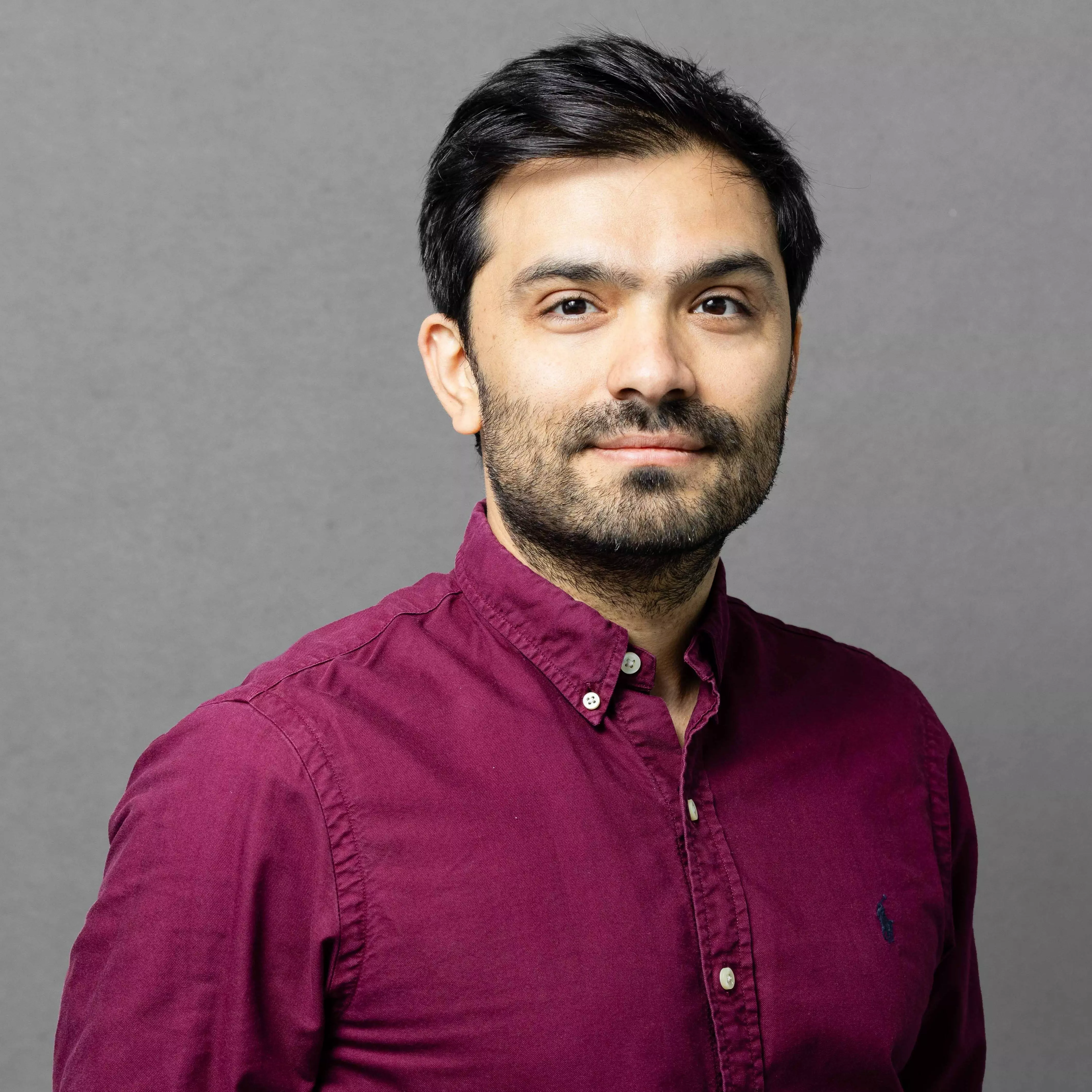
Prof. Dr. Ziawasch Abedjan
Research Group Lead
Ziawasch Abedjan is Full Professor and Chair of the Data Integration and Data Preparation group at BIFOLD and TU Berlin.
Ziawasch Abedjan is Full Professor and Chair of the Data Integration and Data Preparation group at BIFOLD and TU Berlin.
Ziawasch received his PhD from the Hasso Plattner Institute in Potsdam, Germany and spent two years as a postdoctoral associate at MIT. From 2016 - 2020 he was Junior Professor at the TU Berlin and senior researcher at DFKI. 2020 he was appointed Full Professor and Chair of the „Datenbanken und Informationssysteme“ group at Leibniz Universität Hannover.
His research is supported by additional funding from the DFG, the Federal Ministry for Research and Education, and the Federal Ministry of Transport, Building and Urban Development.
2019 | SIGMOD Most Reproducible Paper Award |
2019 | GI Junior Fellow |
2019 | BTW 2019 Winner of Data Science Challenge |
2015 | SIGMOD Best Demonstration Award |
2014 | CIKM Best Student Paper Award |
2014 | ESWC Best Workshop Paper Award |
2013 | Best Dissertation Award of the University of Potsdam |
2010-2014 | Scholarship of the HPI Research School |
- Data Science
- Databases
- Data Integration
- Data Mining
- Machine Learning
- ACM
- GI
Binger Chen, Jacek Golebiowski, Ziawasch Abedjan
Data Augmentation for Supervised Code Translation Learning
Yupei Du, Jacek Golebiowski, Philipp Schmidt, Ziawasch Abedjan
Guiding Catalogue Enrichment with User Queries
Binger Chen, Jacek Golebiowski, Ziawasch Abedjan
Towards Data Augmentation for Supervised Code Translation
Felix Neutatz, Marius Lindauer, Ziawasch Abedjan
AutoML in heavily constrained applications
Felix Neutatz, Ziawasch Abedjan
What is “Good” Training Data? - Data Quality Dimensions that Matter for Machine Learning
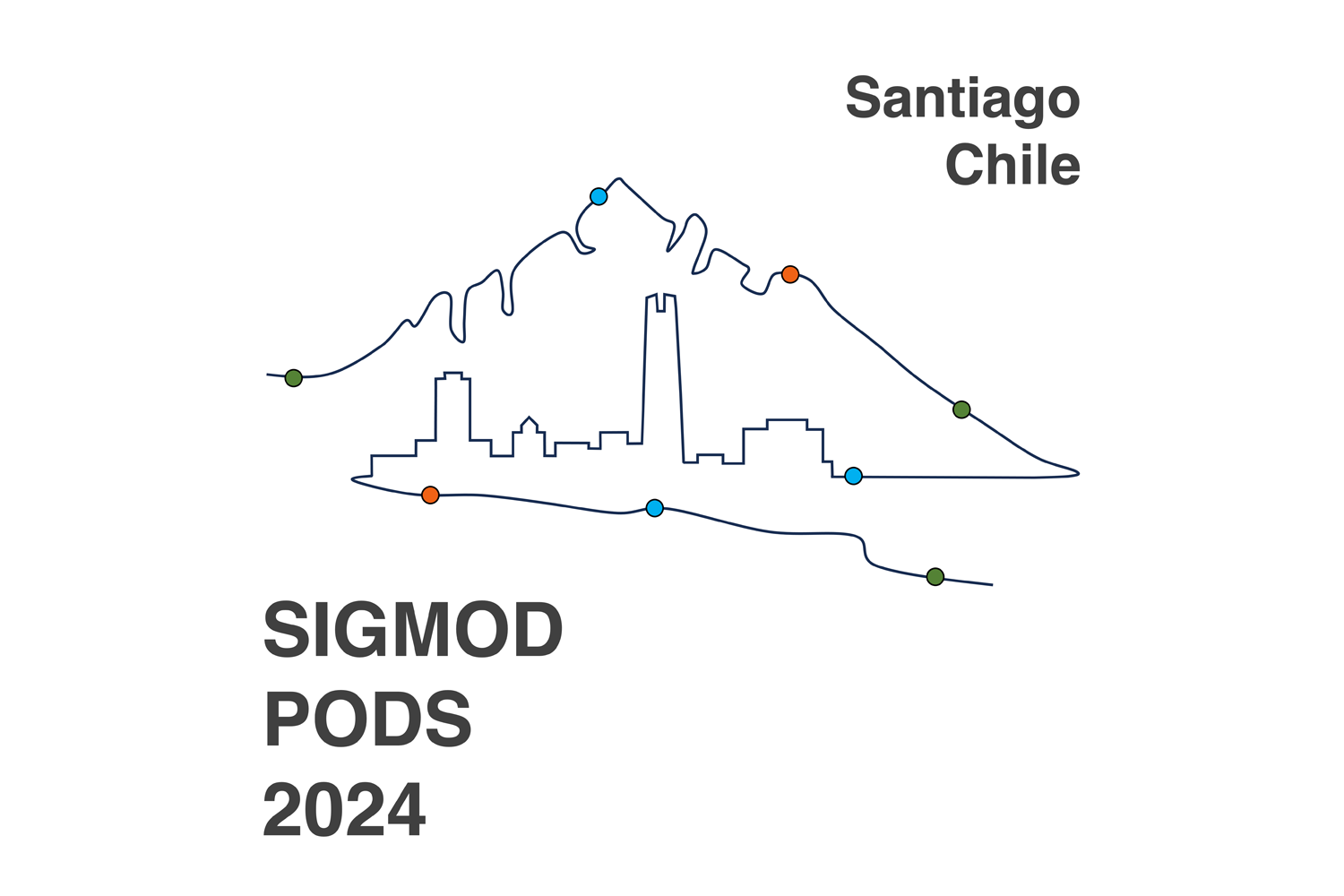
BIFOLD at the 2024 ACM SIGMOD/PODS Conference
BIFOLD researchers presented four research papers, two demos, one workshop paper and were of a panel at the 2024 ACM SIGMOD/ PODS Conference in Santiago, Chile.
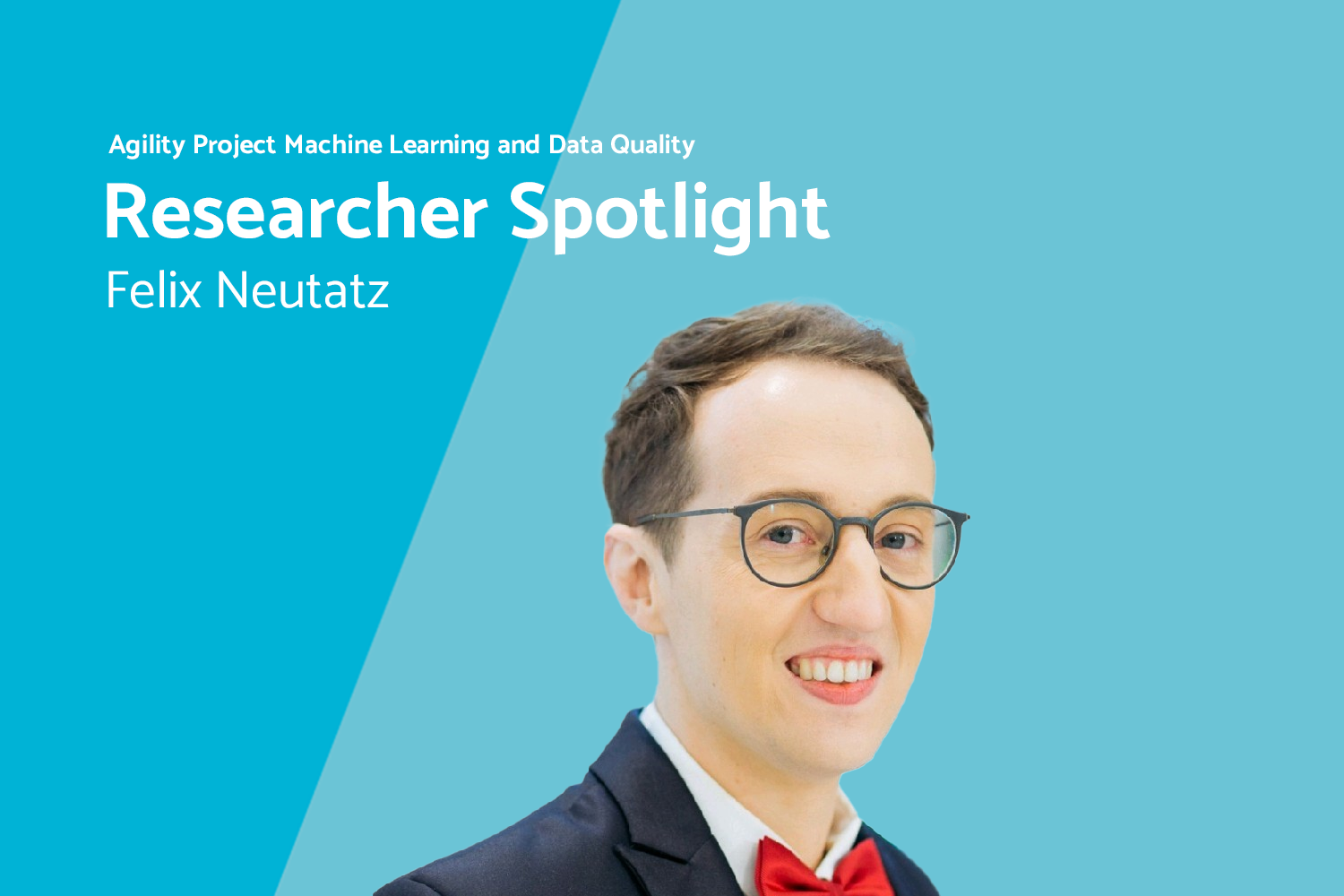
Researcher Spotlight: Felix Neutatz
"Machine Learning and Data Quality" is the first concluded BIFOLD Agility Project. Get to know BIFOLD researcher Felix Neutatz and gain valuable insights into the project, his work and the Agility Program.
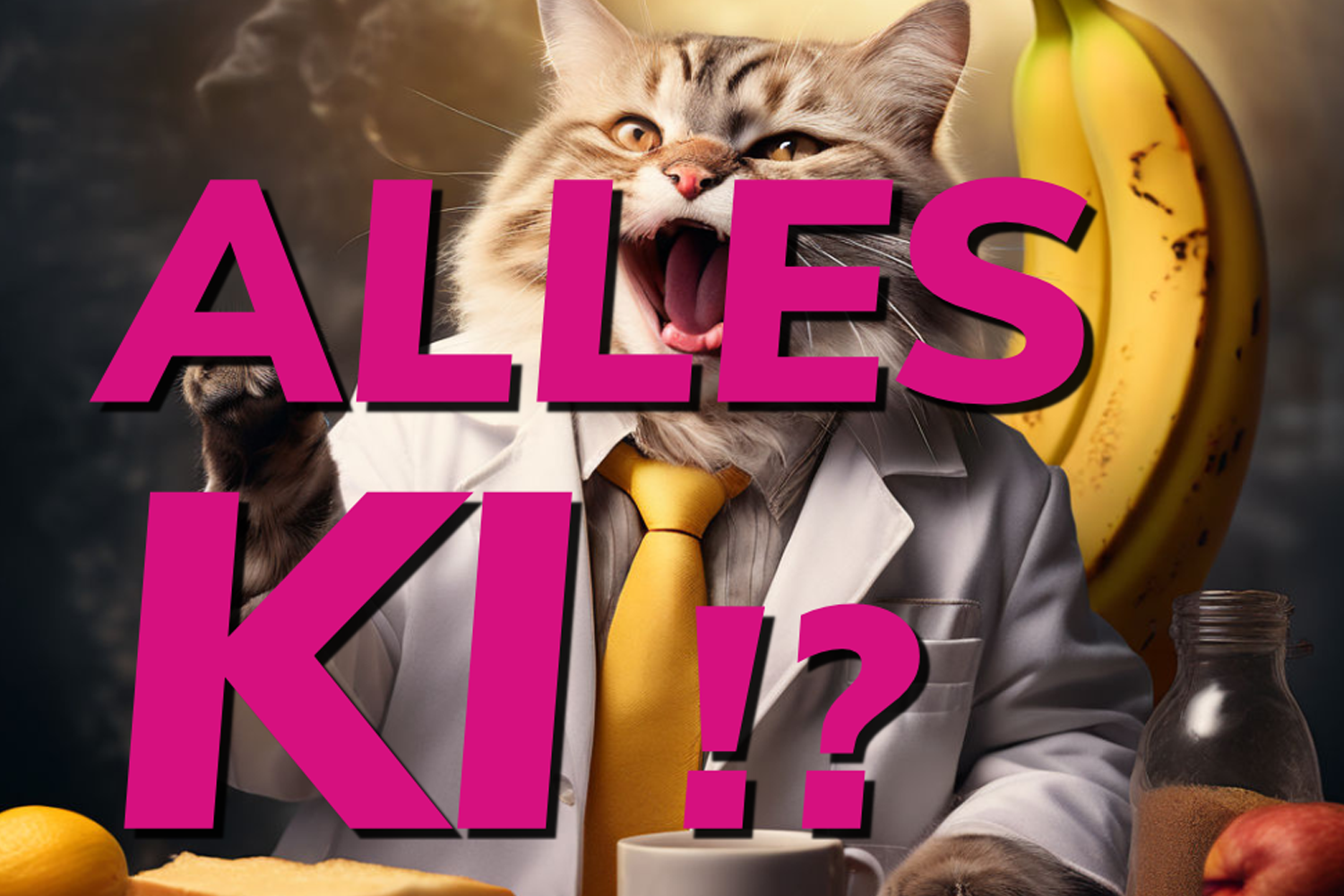
“KI ist was es isst”: Prof. Dr. Ziawasch Abedjan on Data Quality at TU Berlin
At the “Tag der Lehre” (Teaching Day) at the TU Berlin on May 27, 2024, BIFOLD Research Group Lead Prof. Dr. Ziawasch Abedjan welcomed the attendees with a 20-minute talk on the quality of training data.
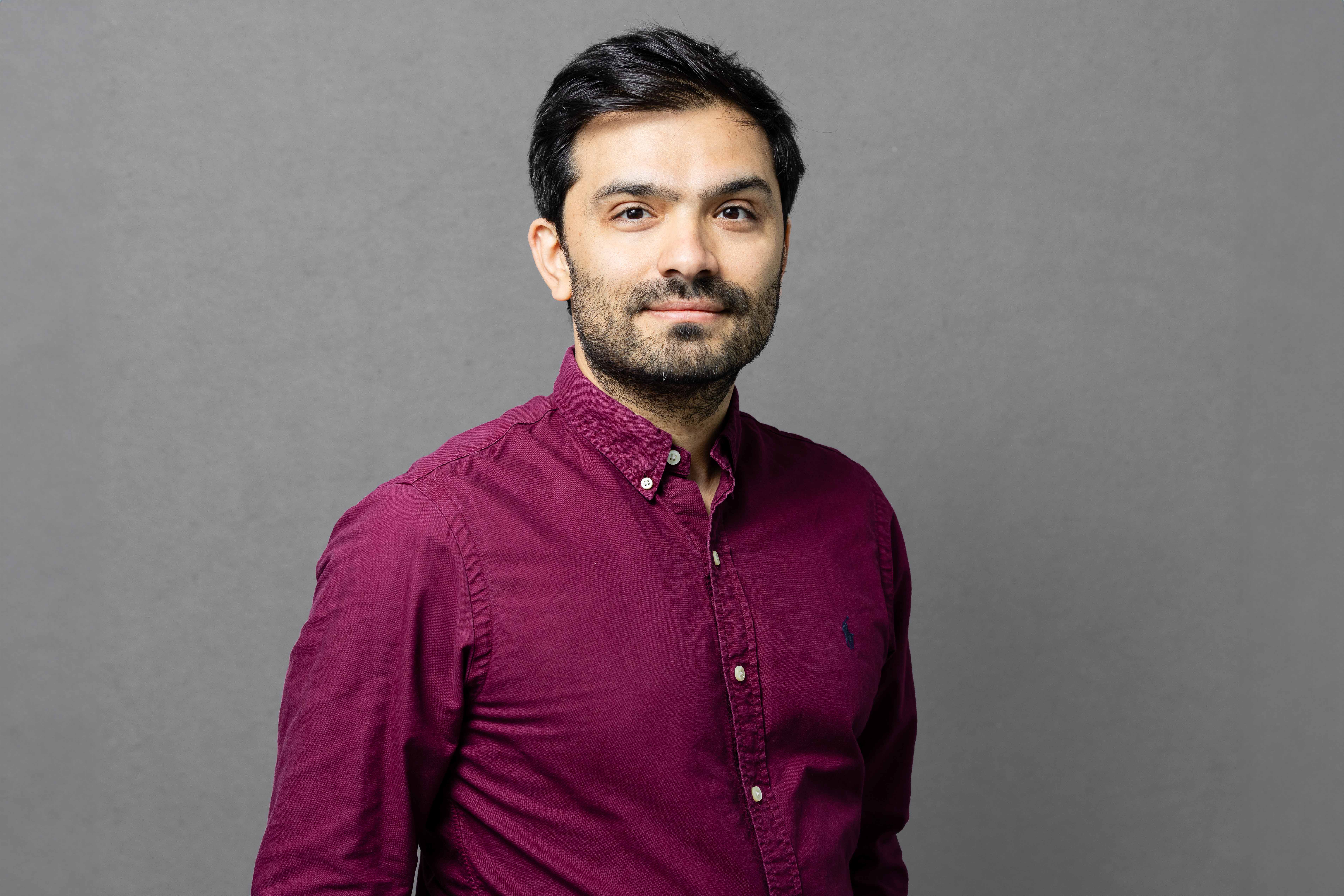
Welcome Prof. Dr. Ziawasch Abedjan
As of March 1, 2024, BIFOLD welcomes a new Research Group Lead in Berlin: Prof. Dr. Ziawasch Abedjan heads the new chair for Data Integration and Data Preparation. In particular, he develops new algorithms for the automatic preparation, extraction, and cleaning of datasets for data science workflows.
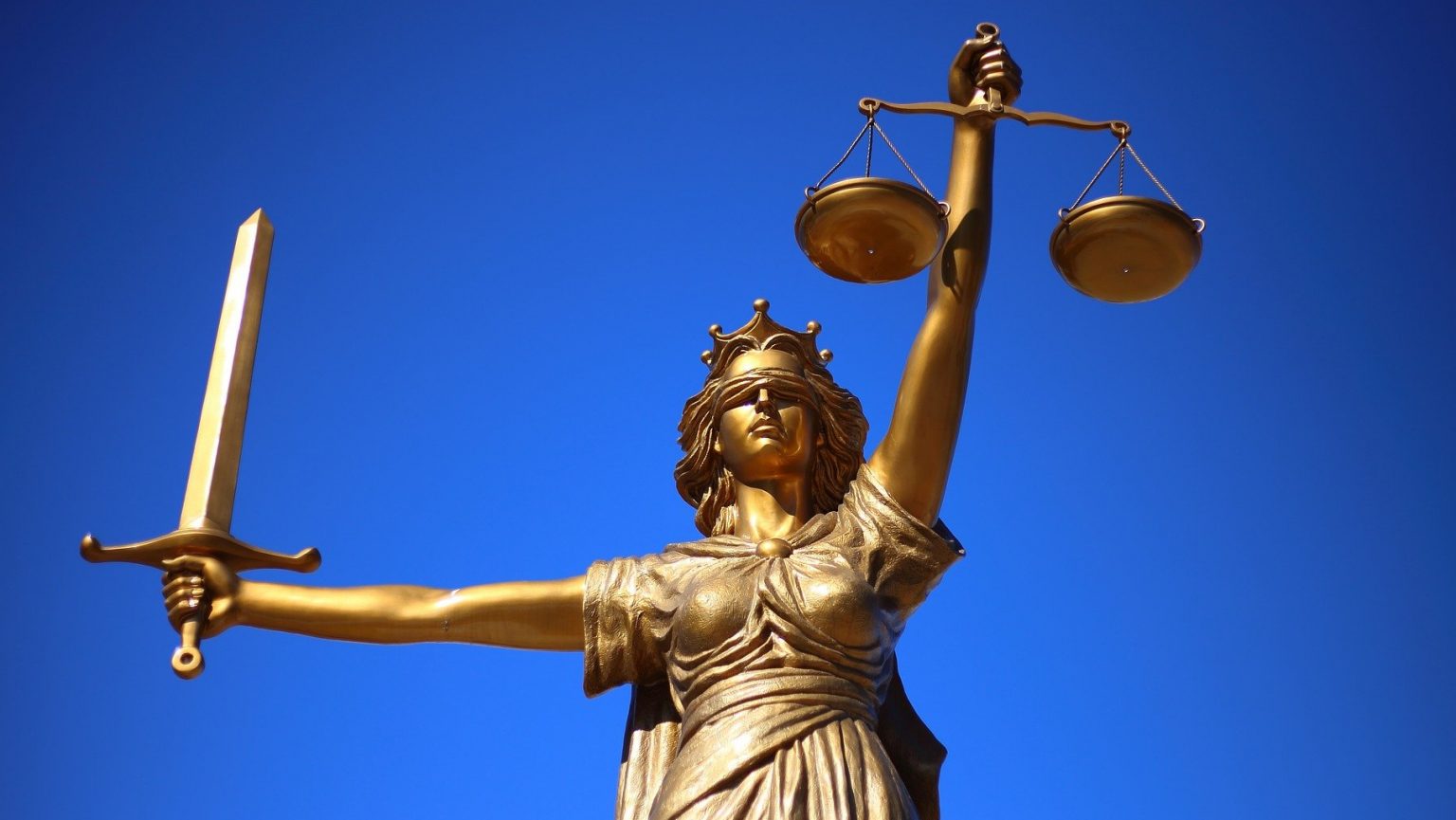
In search for algorithmic fairness
Artificial intelligence (AI) has found its way into many work routines – be it the development of hiring procedures, the granting of loans, or even law enforcement. However, the machine learning (ML) systems behind these procedures repeatedly attract attention by distorting results or even discriminating against people on the basis of gender or race. “Accuracy is one essential factor of machine learning models, but fairness and robustness are at least as important,” knows Felix Neutatz, a BIFOLD doctoral student in the group of Prof. Dr. Ziawasch Abedjan, BIFOLD researcher and former professor at TU Berlin who recently moved to Leibniz Universität Hannover. Together with Ricardo Salazar Diaz they published “Automated Feature Engineering for Algorithmic Fairness“, a paper on fairness of machine learning models in Proceedings of the VLDB Endowment.
An overview of the current state of research in BIFOLD
Since the official announcement of the Berlin Institute for the Foundations of Learning and Data in January 2020, BIFOLD researchers achieved a wide array of advancements in the domains of Machine Learning and Big Data Management as well as in a variety of application areas by developing new Systems and creating impactfull publications. The following summary provides an overview of recent research activities and successes.
Researchers in Prof. Abedjan’s group win SIGMOD reproducibility award
The paper “Raha: A Configuration-Free Error Detection System” by Mohammad Mahdavi, Ziawasch Abedjan, Raul Castro Fernandez, Samuel Madden, Mourad Ouzzani, Michael Stonebraker, and Nan Tang won the ACM SIGMOD Most Reproducible Paper Award.
Four papers authored by TU Berlin and DFKI researchers have been accepted at SIGMOD 2020
Data management systems researchers in the Database Systems and Information Management (DIMA) Group at TU Berlin and the Intelligent Analytics for Massive Data (IAM) Group at DFKI (the German Research Institute for Artificial Intelligence) were informed that their papers have been accepted at the 2020 ACM SIGMOD/PODS International Conference on the Management of Data.