Ensuring Safety and Interpretability in Machine Learning Pipelines
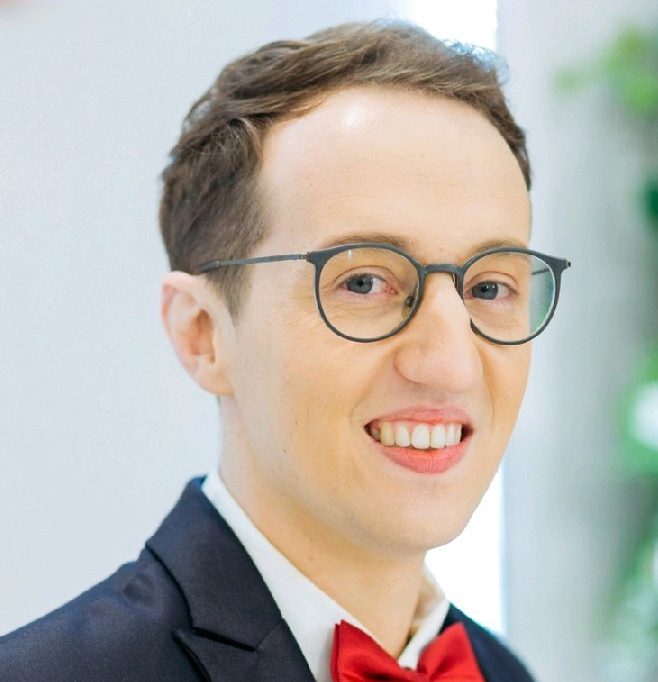
Until April 2024 Felix Neutatz was a PhD candidate at BIFOLD and part of the "Machine Learning and Data Quality" agility project, led by Professor Dr. Ziawasch Abedjan. His research focuses on the intersection of data management and declarative machine learning and he plans to defend his thesis in summer 2024. In this interview Felix describes the challenges and results of the project.
Please describe and explain your research focus?
Felix: Currently, most Machine Learning researchers focus on improving model predictive performance, but there are many more dimensions in Machine Learning applications, such as efficiency, fairness, privacy, safety, and interpretability. My research focuses on providing data scientists a way to automatically train Machine Learning pipelines that satisfy constraints across all these dimensions.
For instance, in an autonomous driving use case, one must train a model to detect pedestrians. The model has to fit into the car's compute environment, predict quickly, and work well across the whole population of pedestrians.
What personally motivated you to enter this specific research-field?
Felix: I enjoy solving challenging problems. Since high school, I have been constantly working on computer science problems that excite me.
What was your greatest success/failure as a scientist?
Felix: My greatest success was to build an Auto Machine Learning system that outperformed Amazon’s Auto Machine Learning system.
Failure is part of being a scientist. Important is that you fail fast to make progress quickly. So, my biggest failure was to fail too slow in some cases.
Agility ProjectsThe BIFOLD Agility Program enables researchers to swiftly exploit scientific opportunities by funding interdisciplinary, collaborative projects among Research Groups, fellows, and external partners. The "Machine Learning and Data Quality" Agility Project built a system that automatically generates Machine Learning pipelines that fulfill the user-defined constraints; the systems scale to 100s of hyperparameters and dozens of continuous constraints. Additionally, these systems adapt the search and validation strategy and the Machine Learning hyperparameter space based on the given data set and the defined constraints. All current Agility Projects can be found here |
Which major innovation do you expect in your research field in the next ten years?
Felix: Generative Machine Learning will be used in more and more cases and will improve many research areas.
Which living or historical scientist has fascinated you and why?
Felix: I am a big fan of Andrew Ng because he was the first to offer a free online course on machine learning. I learned a lot from him.
AI is considered a disruptive technology - in which areas of life do you expect the greatest upheaval in the next ten years?
Felix: Education, workplace, and entertainment: For language models, there are no “stupid” questions, which makes learning much faster. In the workplace, many simple processes and documentation can be automated. For entertainment, we can dive into worlds and talk to characters in a way that would have never been possible.
Where would one find you, if you are not sitting in front of the computer?
Felix: In my winter garden to get some sun or go for a walk or run along the Spree.
Paper HighlightThe paper “AutoML in Heavily Constrained Applications” received the L3S Best Publication Award. The paper proposes an efficient solution for constraint-driven AutoML by leveraging meta-learning. This solution is called Caml. It uses meta-learning to automatically adapt its own AutoML parameters, such as the search strategy, the validation strategy, and the search space, for a task at hand. The dynamic AutoML strategy of Caml takes user-defined constraints into account and obtains constraint-satisfying pipelines with high predictive performance. Neutatz, F., Lindauer, M., & Abedjan, Z. (2023). AutoML in Heavily Constrained Applications. VLDB Journal. https://doi.org/10.1007/s00778-023-00820-1 |