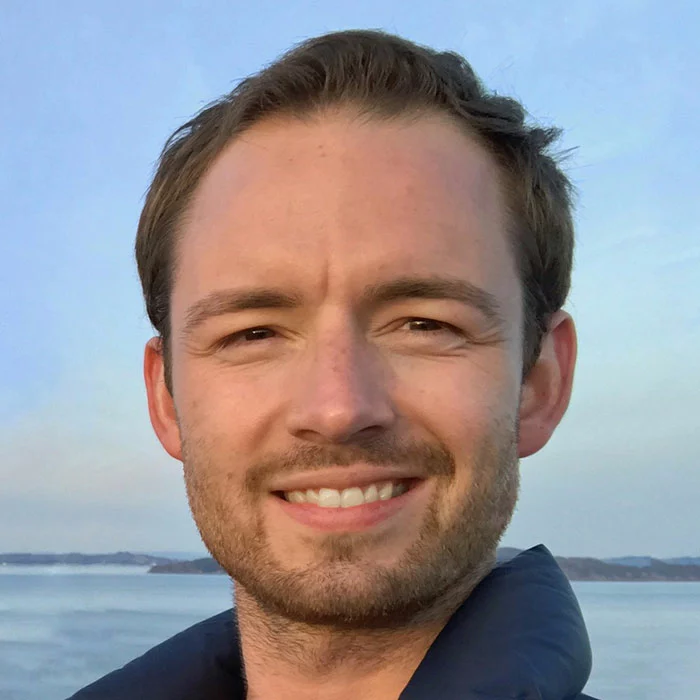
Dr. Stefan Chmiela
Research Junior Group Lead
Dr. Stefan Chmiela leads the Research Training Group "Machine learning for molecular simulations in quantum chemistry".
2019 | Chorafas-Award |
- Hilbert space learning methods
- Learning from structured data
- Data efficient learning with explicit prior knowledge constraints
J. Thorben Frank, Winfried Ripken, Gregor Lied, Klaus-Robert Müller, Oliver T. Unke, Stefan Chmiela
Sampling 3D Molecular Conformers with Diffusion Transformers
Anton Charkin-Gorbulin, Artem Kokorin, Huziel E. Sauceda, Stefan Chmiela ,Claudio Quarti ,David Beljonne ,Alexandre Tkatchenko ,Igor Poltavsky
Atomic Orbits in Molecules and Materials for Improving Machine Learning Force Fields
Oz Y. Mendelsohn, Michal Hartstein, Stefan Chmiela, Alexandre Tkatchenko, Leeor Kronik
Stable molecular dynamics simulations of halide perovskites from a temperature-ensemble gradient-domain machine learning approach
J. Thorben Frank, Stefan Chmiela, Klaus-Robert Müller, Oliver T. Unke
Euclidean Fast Attention: Machine Learning Global Atomic Representations at Linear Cost
Adil Kabylda, J. Thorben Frank, Sergio Suarez Dou, Almaz Khabibrakhmanov, Leonardo Medrano Sandonas, Oliver T. Unke, Stefan Chmiela, Klaus-Robert Müller, Alexandre Tkatchenko
Molecular Simulations with a Pretrained Neural Network and Universal Pairwise Force Fields

Simulation of quantum systems
Researchers from the Berlin Institute for the Foundations of Learning and Data (BIFOLD) at TU Berlin and Google DeepMind have now developed a novel machine learning algorithm which enables highly accurate simulations of the dynamics of a single or multiple molecule on long time-scales.
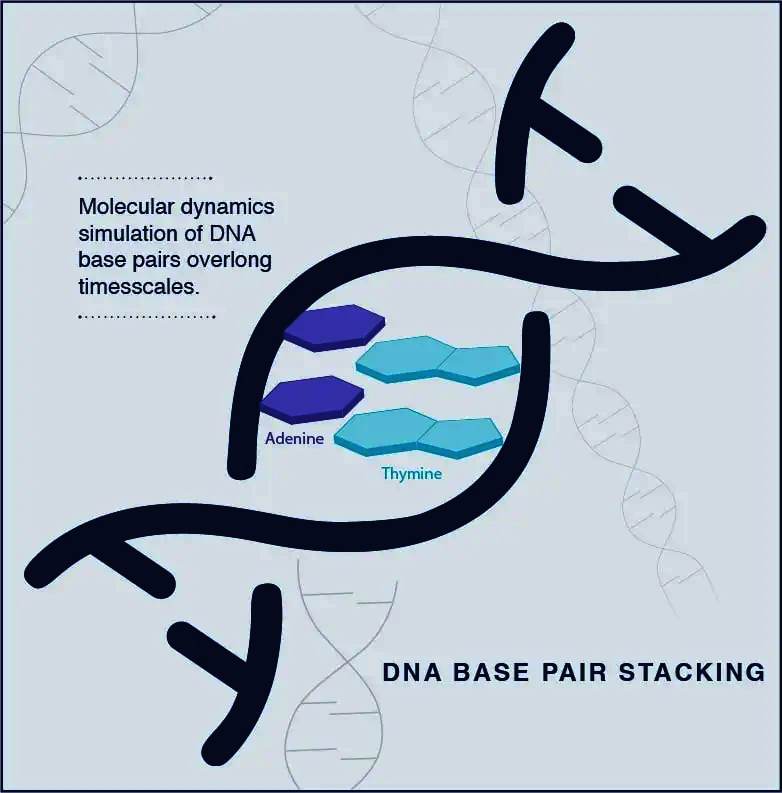
Simulation of complex quantum systems
An international team of BIFOLD scientists together with scientists from the Université du Luxembourg and Google has now successfully developed a machine learning algorithm to simulate complex quantum system.
Machine Learning meets Quantum Physics
BIFOLD researchers contributed to an in-depth referenced work on the physics-based machine learning techniques that model electronic and atomistic properties of matter.