BIFOLD AI researchers receive DSP Best Paper Prize
The Berlin Institute for the Foundations of Learning and Data (BIFOLD) is well-distinguished for its expertise in Artificial Intelligence (AI). As a result, the BIFOLD leading researchers Prof. Wojciech Samek, Prof. Klaus-Robert Müller and Prof. Grégoire Montavon were now honored by the journal Digital Signal Processing (DSP) with the 2022 Best Paper Prize. Importantly, the DSP mention of excellence highlights research findings published within the last five years. Awarding the article entitled “Methods for interpreting and understanding deep neural networks” (2018), DSP accentuates the scientists’ outstanding work on the interpretability of AI models.
DSP represents one of the most established journals in the field of signal processing. The journal curates multi-disciplinary research insights, providing a platform for the publication of cutting-edge theory with academic and industrial appeal. As exemplified in honoring the paper by BIFOLD researchers Prof. Wojciech Samek, Prof. Klaus-Robert Müller and Prof. Grégoire Montavon, articles on emerging applications of signal processing and machine learning (ML) form a vital part of DSP’s archive. Moreover, the best paper prize underlines the productive institution-spanning cooperation between the Fraunhofer Heinrich Hertz Institute and the Technical University Berlin under the umbrella of BIFOLD.
Contextually, Deep Neural Networks (DNN) have recently powered myriads of applications such as image classification, speech recognition, or natural language processing. In practice, its advantage conveys extremely high predictive accuracy – often on par with human performance. By consequence, a robust validation procedure is essential to implementing desired, however contested technology (e.g. self-driving cars). Methods for interpreting what the model has learned are thus of high societal relevance to ensure sustainable and safe use cases.
In this realm, BIFOLD researchers Prof. Wojciech Samek, Prof. Grégoire Montavon and Prof. Klaus-Robert Müller focus on post-hoc interpretability. The concept tethers to a trained model to understand the model’s representation as well as its decision-making strategies. With the award-winning paper, the researchers provide an entry point to the problem of interpreting a deep neural network model and explaining its predictions. Moreover, they also provide theoretical insights, recommendations and tricks, and discuss a number of technical challenges, and possible applications.
In a nutshell, the AI experts wrote a rigorously clear account of ML’s embeddedness in real-world decision processes, thereby stressing the necessity for transparent DNNs. Concisely, their tutorial paper covered two approaches for improving ML transparency: 1) interpreting the concepts learned by a model via building prototypes and (2) explaining the model's decisions by identifying the relevant input variables. Bearing comparison with DSP’s rigorous shortlisting process, BIFOLD’s expertise in the interpretability of AI models proves its excellent standing in the research community.
Find the awarded paper here.
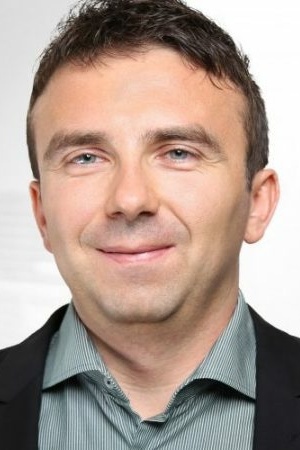
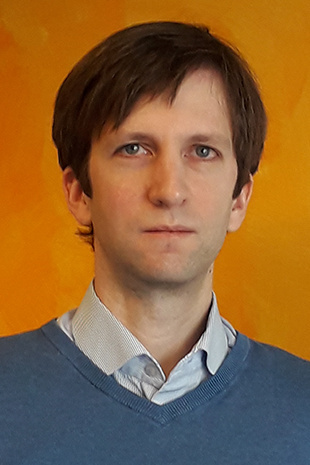