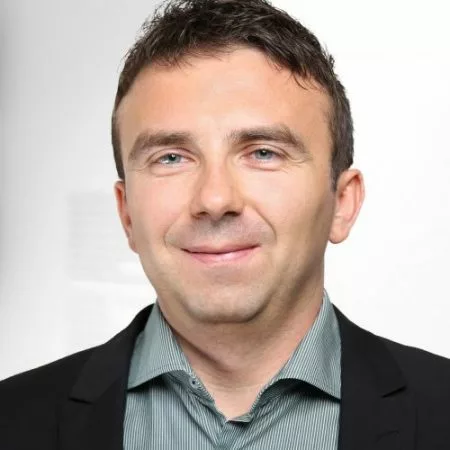
Prof. Dr. Wojciech Samek
Fellow
Fellow | BIFOLD
Professor | Technische Universität Berlin
Head of AI Department | Fraunhofer HHI
Wojciech Samek is a professor in the department of Electrical Engineering and Computer Science at the Technical University of Berlin and is jointly heading the department of Artificial Intelligence and the Explainable AI Group at Fraunhofer Heinrich Hertz Institute (HHI), Berlin, Germany. He studied computer science at Humboldt University of Berlin from 2004 to 2010, was visiting researcher at NASA Ames Research Center, CA, USA, and received the Ph.D. degree in machine learning from the Technische Universität Berlin in 2014. He is associated faculty at the ELLIS Unit Berlin and the DFG Graduate School BIOQIC, and member of the scientific advisory board of IDEAS NCBR. Furthermore, he is a senior editor of IEEE TNNLS, an editorial board member of Pattern Recognition, and an elected member of the IEEE MLSP Technical Committee. He is recipient of multiple best paper awards, including the 2020 Pattern Recognition Best Paper Award, and part of the expert group developing the ISO/IEC MPEG-17 NNC standard. He is the leading editor of the Springer book “Explainable AI: Interpreting, Explaining and Visualizing Deep Learning” and organizer of various special sessions, workshops and tutorials on topics such as explainable AI, neural network compression, and federated learning. He has co-authored more than 150 peer-reviewed journal and conference papers; some of them listed by Thomson Reuters as “Highly Cited Papers” (i.e., top 1%) in the field of Engineering.
2021 | 2020 Pattern Recognition Best Paper Award |
2019 | Best Paper Award at ICML Workshop on On-Device Machine Learning & Compact Deep Neural Network Representations |
2019 | Honorable Mention Award at IEEE AIVR |
2016 | Best Paper Award at ICML Workshop on Visualization for Deep Learning |
2010 | Scholarship of the DFG Research Training Group GRK 1589/1 |
2006 | Scholarship of the German National Merit Foundation (“Studienstiftung des deutschen Volkes”) |
- Deep Learning
- Interpretable Machine Learning
- Model Compression
- Computer Vision
- Distributed Learning
Jonas R. Naujoks, Aleksander Krasowski, Moritz Weckbecker, Galip Ümit Yolcu, Thomas Wiegand, Sebastian Lapuschkin, Wojciech Samek, René P. Klausen
Leveraging Influence Functions for Resampling Data in Physics-Informed Neural Networks
Sayed Mohammad Vakilzadeh Hatefi, Maximilian Dreyer, Reduan Achtibat, Patrick Kahardipraja, Thomas Wiegand, Wojciech Samek, Sebastian Lapuschkin
Attribution-guided Pruning for Compression, Circuit Discovery, and Targeted Correction in LLMs
Emílio Dolgener Cantú, Rolf Klemens Wittmann, Oliver Abdeen, Patrick Wagner, Wojciech Samek, Moritz Baier, Sebastian Lapuschkin
Deep Learning-based Multi Project InP Wafer Simulation for Unsupervised Surface Defect Detection
Shreyas Gururaj, Lars Grüne, Wojciech Samek, Sebastian Lapuschkin, Leander Weber
Relevance-driven Input Dropout: an Explanation-guided Regularization Technique
Maximilian Dreyer, Lorenz Hufe, Jim Berend, Thomas Wiegand, Sebastian Lapuschkin, Wojciech Samek
From What to How: Attributing CLIP's Latent Components Reveals Unexpected Semantic Reliance
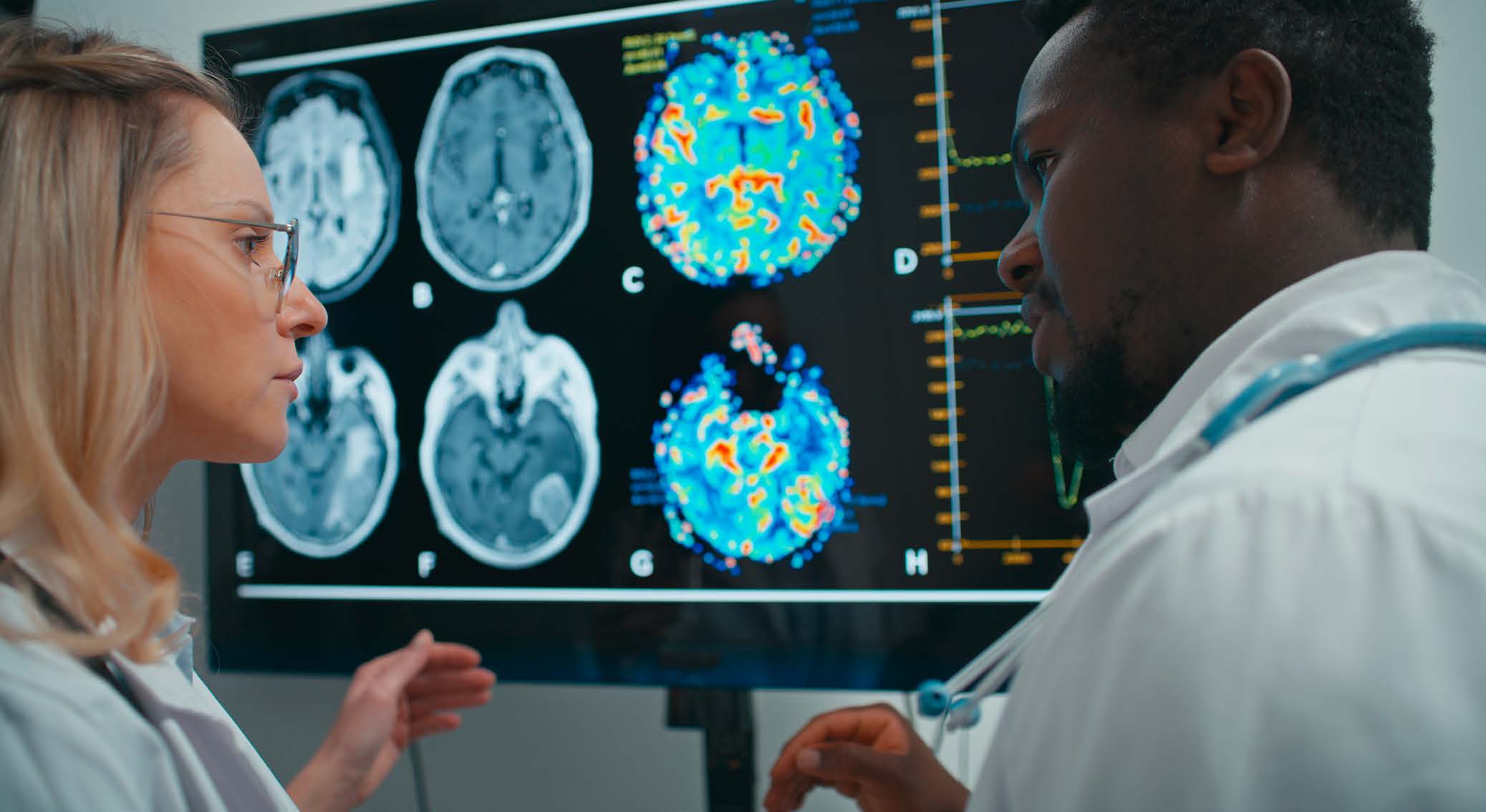
New Whitepaper on XAI
Prof. Dr. Wojciech Samek is co-author of the new white paper “Explainable AI”, published today by the Plattform Lernende Systeme. Together with colleagues from the “Technological Enablers and Data Science” working group of the Platform Lernende Systeme, they explored different application areas, guided by the question: Who should be told what, how and for what purpose?
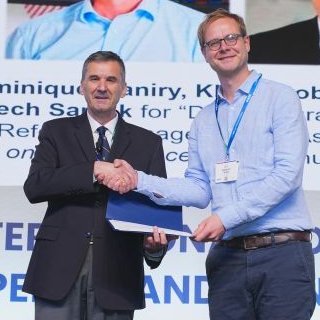
Best Paper Award for groundbreaking work on image quality assessment
Researchers from Fraunhofer Heinrich Hertz Institute and BIFOLD have received the prestigious IEEE Signal Processing Society Best Paper Award for their groundbreaking work on image quality assessment using deep neural networks.
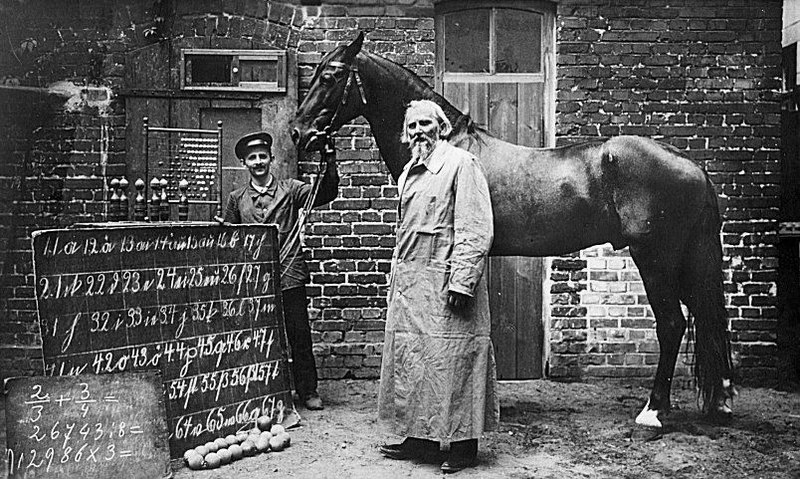
The Hidden Domino Effect
A team of BIFOLD researchers discovered that foundation models such as GPT, Llama, CLIP etc., trained by unsupervised learning methods, often produce compromised representations from which instances can be correctly predicted, although only supported by data artefacts. In a Nature Machine Intelligence publication the researchers propose an explainable AI technique that is able to detect the false prediction strategies.
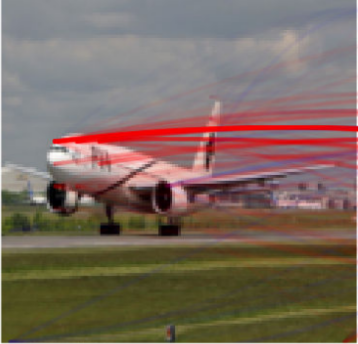
Insight into Deep Generative Models with Explainable AI
The just completed agility project "Understanding Deep Generative Models" focused on making ML models more transparent, in order to uncover hidden flaws in them, a research area known as Explainable AI.
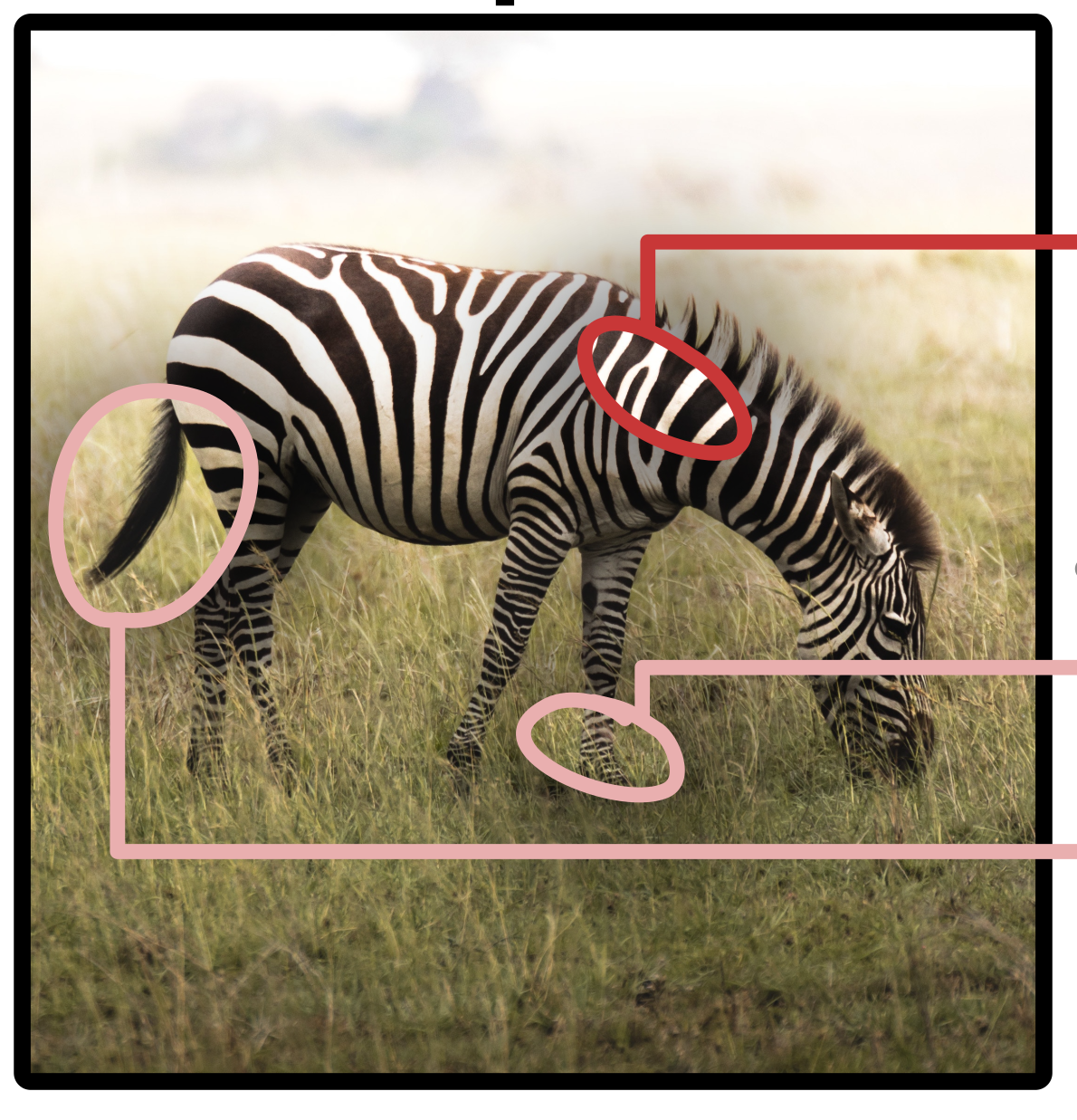
AI - finally explainable to humans
As of today it remains difficult to understand how an AI system reaches its decisions. Scientists at the Fraunhofer Heinrich-Hertz-Institut (HHI) and the Berlin Institute for the Foundations of Learning and Data (BIFOLD) at TU Berlin have collaborated for many years to make AI explainable. In their new paper the researchers present Concept Relevance Propagation (CRP), a new method for explainable AI that can explain individual AI decisions as concepts understandable to humans.
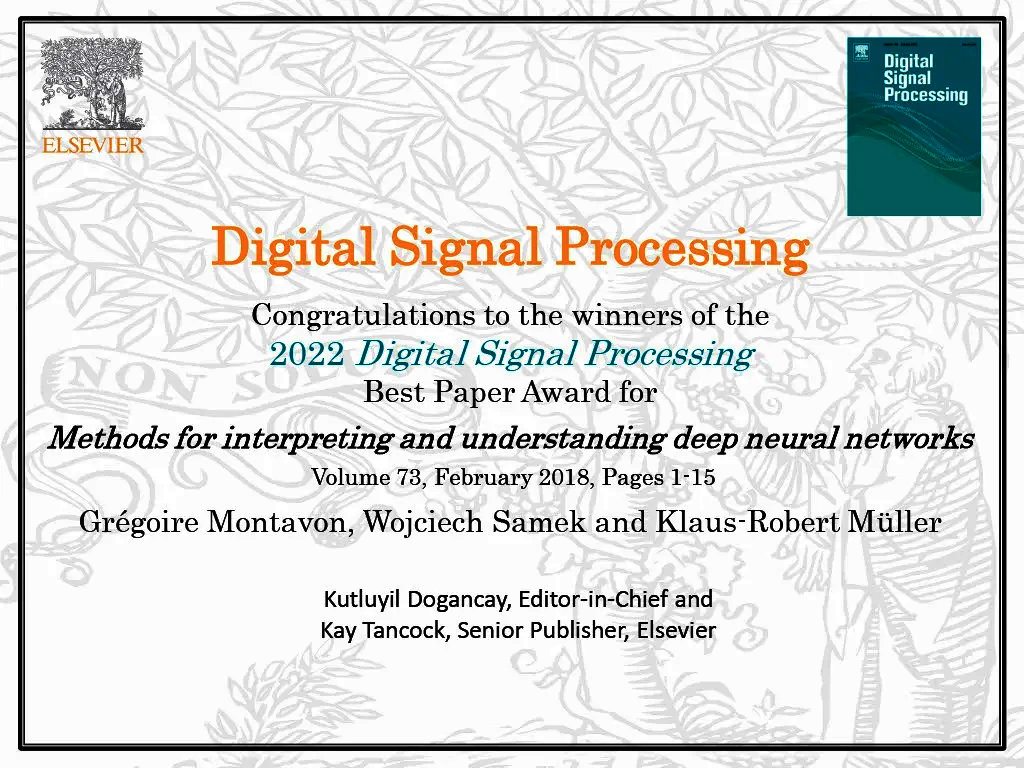
DSP Best Paper Prize
BIFOLD researchers Prof. Klaus-Robert Müller, Prof. Wojciech Samek and Prof. Grégoire Montavon were honored by the journal Digital Signal Processing (DSP) with the 2022 Best Paper Prize. The DSP mention of excellence highlights important research findings published within the last five years.
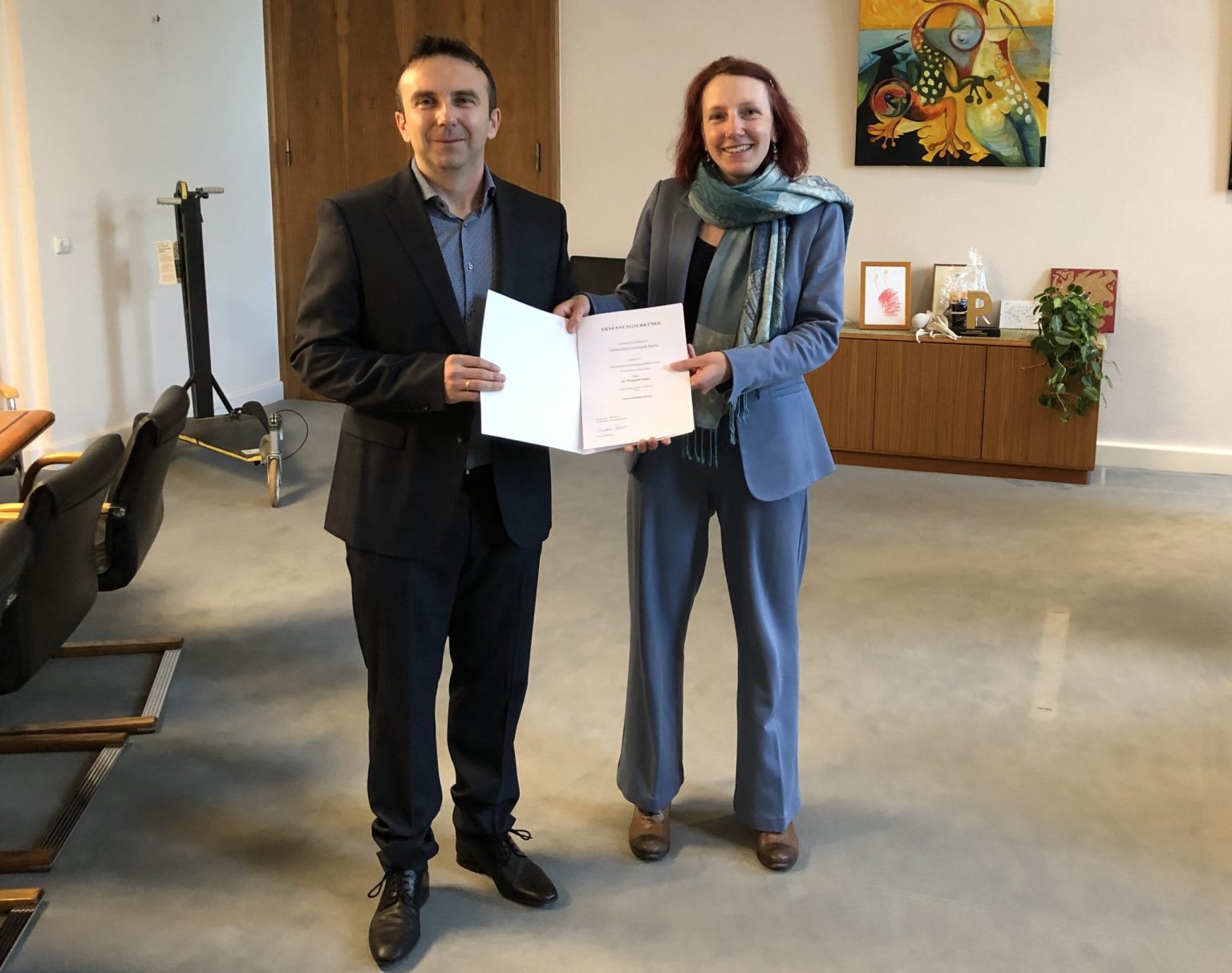
“I want to move beyond purely ‘Explaining’ AI”
BIFOLD researcher Dr. Wojciech Samek has been appointed Professor of Machine Learning and Communications at TU Berlin with effect from 1 May 2022. Professor Samek heads the Department of Artificial Intelligence at the Fraunhofer Heinrich-Hertz-Institute. His goal is to further develop three areas: explainability and trustworthiness of artificial intelligence, the compression of neural networks, and so-called federated leaning. He aims to focus on the practical, methodological, and theoretical aspects of machine learning at the interface to other areas of application.
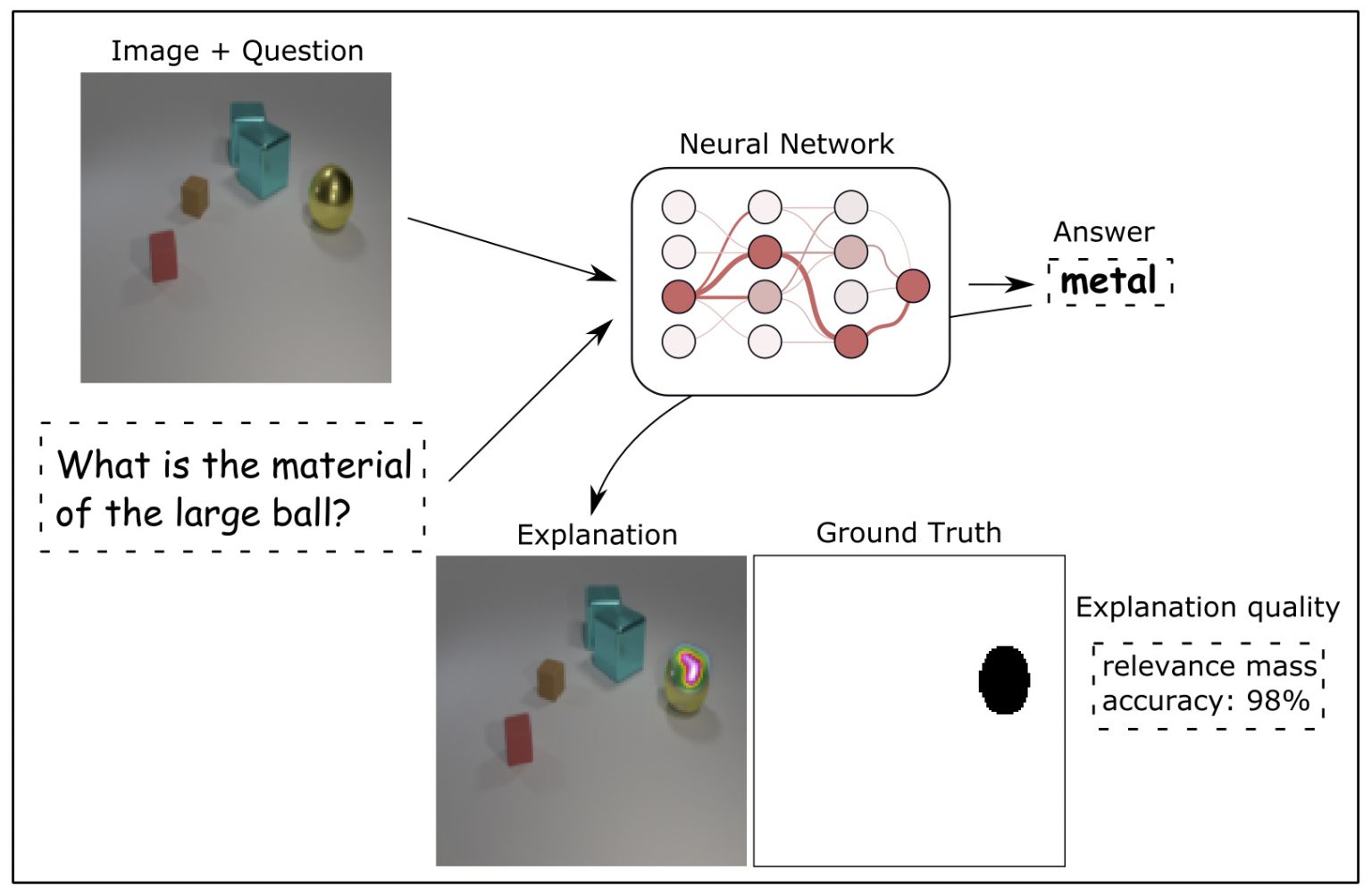
Benchmarking Neural Network Explanations
Neural networks have found their way into many every day applications. During the past years they reached excellent performances on various largescale prediction tasks, ranging from computer vision, language processing or medical diagnosis. Even if in recent years AI research developed various techniques that uncover the decision-making process and detect so called “Clever Hans” predictors – there exists no ground truth-based evaluation framework for such explanation methods. BIFOLD researcher Dr. Wojciech Samek and his colleagues now established an Open Source ground truth framework, that provides a selective, controlled and realistic testbed for the evaluation of neural network explanations. The work will be published in Information Fusion.
New workshop series “Trustworthy AI”
The AI for Good global summit is an all year digital event, featuring a weekly program of keynotes, workshops, interviews or Q&As. BIFOLD Fellow Dr. Wojciech Samek, head of department of Artificial Intelligence at Fraunhofer Heinrich Hertz Institute (HHI), is implementing a new online workshop series “Trustworthy AI” for this platform.
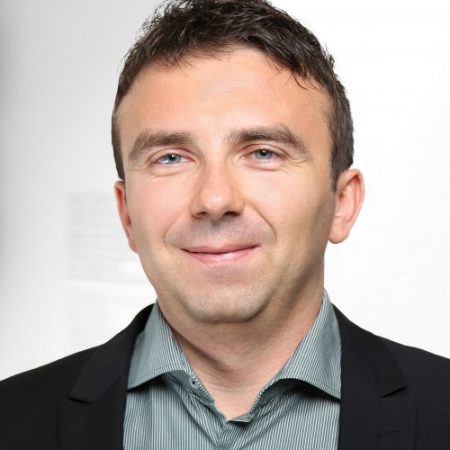
Making the use of AI systems safe
BIFOLD Fellow Dr. Wojciech Samek and Luis Oala (Fraunhofer Heinrich Hertz Institute) together with Jan Macdonald and Maximilian März (TU Berlin) were honored with the award for “best scientific contribution” at this year’s medical imaging conference BVM. Their paper “Interval Neural Networks as Instability Detectors for Image Reconstructions” demonstrates how uncertainty quantification can be used to detect errors in deep learning models.
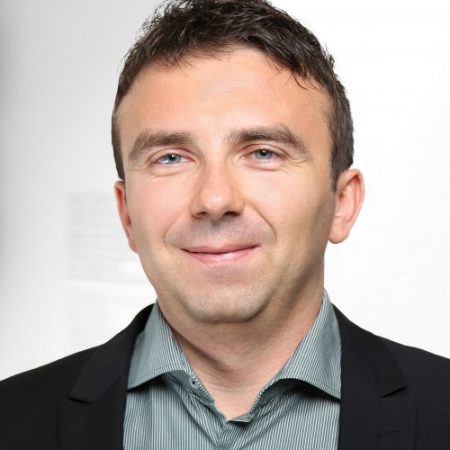
BIFOLD PI Dr. Samek talks about explainable AI at NeurIPS 2020 social event
BIFOLD Principal Investigator Dr. Wojciech Samek (Fraunhofer HHI) talked about explainable and trustworthy AI at the “Decemberfest on Trustworthy AI Research” as part of the annual Conference on Neural Information Processing Systems (NeurIPS 2020). NeurIPS is a leading international conference on neural information processing systems, Machine Learning (ML) and their biological, technological, mathematical, and theoretical aspects.
An overview of the current state of research in BIFOLD
Since the official announcement of the Berlin Institute for the Foundations of Learning and Data in January 2020, BIFOLD researchers achieved a wide array of advancements in the domains of Machine Learning and Big Data Management as well as in a variety of application areas by developing new Systems and creating impactfull publications. The following summary provides an overview of recent research activities and successes.