Call for XAI-Papers!
AI systems are becoming more and more complex. This complexity makes it difficult even for researchers to know why artificial intelligence (AI) makes specific decisions. At the World Conference on Explainable Artificial Intelligence experts from different fields discuss state of the art methods to make AI systems explainable. The venue will take place from July 17th to 19th, 2024, in Malta. BIFOLD researchers take part in the organization of the 2nd World Conference on Explainable Artificial Intelligence. They organize two special tracks and published a Call for Papers. The call is open until March 15th, 2024. All application details can be found here.
Special Track 1: Actionable Explainable AI
Following the success of Explainable AI at generating faithful and understandable explanations of complex ML models, researchers have started to ask how the outcomes of Explainable AI can be used systematically to enable meaningful actions. This includes (1) what type of explanations are most helpful to enable human experts to achieve more efficient and more accurate decision-making, (2) how, based on human feedback on explanations, can one systematically improve the robustness and generalization ability of ML models or make them comply with specific human norms, and (3) how to enable meaningful actioning of real-world systems via interpretable ML-based digital twins. This special track will treat at the same time the technical aspects of how to build highly informative explanations which form the basis for actionability, the question of how to evaluate and improve the quality of actions derived from XAI, and finally, explore real-world use cases where those actions lead to improved outcomes.
Organization: Grégoire Montavon (BIFOLD Research Group Lead / Freie Universität Berlin), and Lorenz Linhardt (BIFOLD researcher).
More information
Special Track 2: Concept-based global explainability
Deep Neural Networks have demonstrated remarkable success across various disciplines, primarily due to their ability to learn intricate data representations. However, the inherent semantic nature of these representations remains elusive, posing challenges for the responsible application of Deep Learning methods, particularly in safety-critical domains. In response to this challenge, this special track delves into the critical aspects of global explainability, a subfield of Explainable AI. Generally, the global explainability methods aim to interpret what abstractions have been learned by the network. This can be achieved by analyzing the network’s reliance on specific concepts in general or by examining individual neurons and their functional roles within models. This approach facilitates the elucidation of abstractions learned by the networks. It extends to identifying and interpreting circuits—computational subgraphs within the models that elucidate information flow within complex architectures. Furthermore, global explainability could be employed to explain the local decision-making of machines, termed glocal explainability.
Organization: Marina Marie-Claire Höhne (BIFOLD Fellow), Kiril Bykov (BIFOLD researcher) together with our colleagues from Fraunhofer Heinrich Hertz Institute HHI, Sebastian Lapuschkin, Maximilian Dreyer, Johanna Vielhaben
More Information
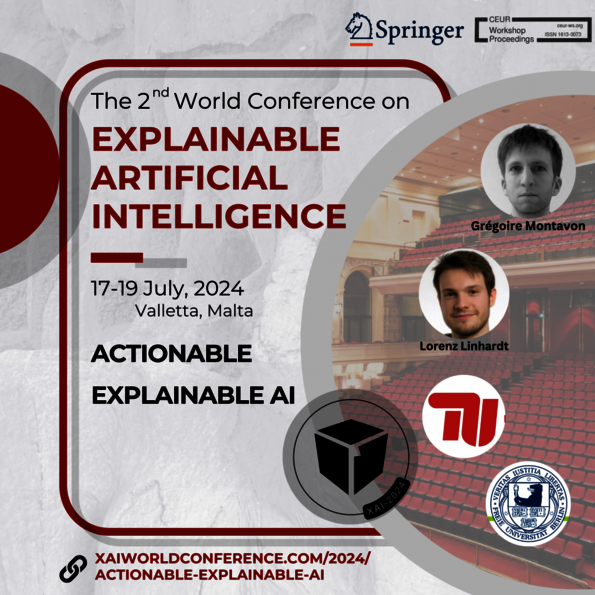
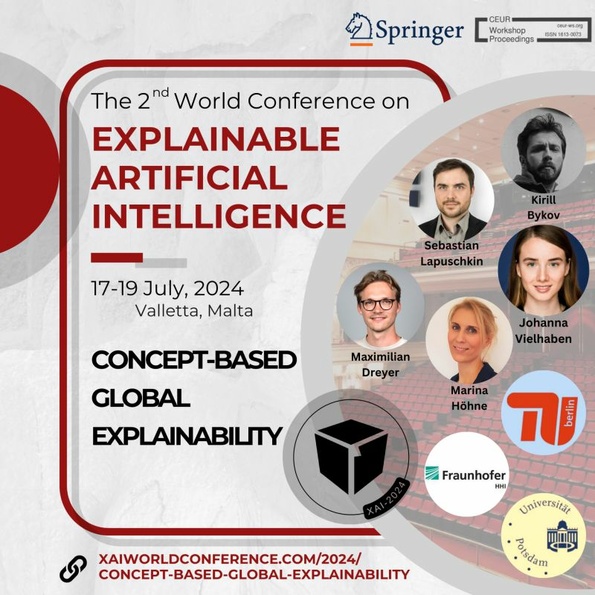