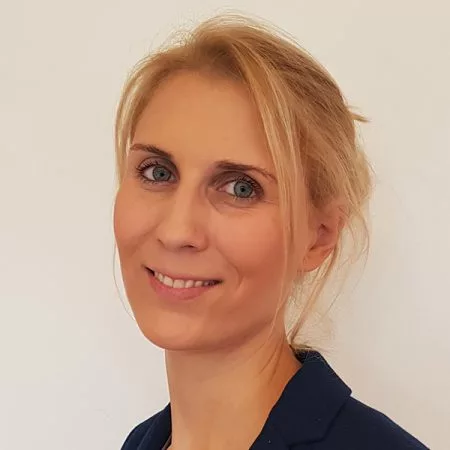
Prof. Dr. Marina Marie-Claire Höhne (Née Vidovic)
Fellow
Junior Fellow | BIFOLD
Head of Department: Data Science in Bioeconomy, Leibniz-Institut für Agrartechnik und Bioökonomie
Marina M.-C. Höhne (née Vidovic) received the Master’s degree in Technomathematics in 2012. From 2012 to 2014 she worked as a researcher at Ottobock in Vienna, Austria, on time series data and domain adaptation for controlling prosthetic devices. In 2014 she started her PhD on explainable AI and received the Dr. rer. nat. degree with summa cum laude from TU Berlin in 2017. Afterwards from 2017 to 2018 she took one year maternity leave, and continued working at the machine learning chair at TU Berlin as a postdoctoral researcher in 2018. In this time, from 2018-2020, she has been lecturing seminars and lectures in machine learning, supervised bachelor, master and PhD students and continued her studies in explainable AI and domain adaptation. In 2020 she started her own junior research group – Understandable Machine Intelligence (UMI) lab – in the area of explainable AI at TU Berlin, funded by the german federal ministry of education and research.
Furthermore, she received the best paper prize at the workshop for explainable AI for complex systems in 2016. She is a reviewer for NeurIPS and ICML and she serves as a reviewer for the german federal ministry of education and research (BMBF). In 2021 she joined the Berlin Institute for the Foundations of Learning and Data (BIFOLD) as a junior fellow.
2017 | Dr. rer. nat.: summa cum laude |
2016 | Best paper award at NeurIPS Workshop on AI explainability for complex system |
- Explainable Artificial Intelligence (XAI) – local & global
- Robustness of Neural Networks and XAI Methods
- Domain Adaptation
- Representation Learning
- Applications: EMG, EEG, Brain Computer Interfaces, Computer Vision, Bioinformatics, Digital Pathology, Climate Data Analysis
- ProFil
Carlos Eiras-Franco, Anna Hedström, Marina M.-C. Höhne
Evaluate with the Inverse: Efficient Approximation of Latent Explanation Quality Distribution
Anna Hedström, Philine Lou Bommer, Thomas F Burns, Sebastian Lapuschkin, Wojciech Samek, Marina MC Höhne
Evaluating Interpretable Methods via Geometric Alignment of Functional Distortions
Kristoffer Wickstrøm, Marina M.-C.Höhne, Anna Hedström
From Flexibility to Manipulation: The Slippery Slope of XAI Evaluation
Laura Kopf, Philine Lou Bommer, Anna Hedström, Sebastian Lapuschkin, Marina M.-C. Höhne, Kirill Bykov
CoSy: Evaluating Textual Explanations of Neurons
Anna Hedström, Leander Weber, Sebastian Lapuschkin, Marina Höhne
A Fresh Look at Sanity Checks for Saliency Maps
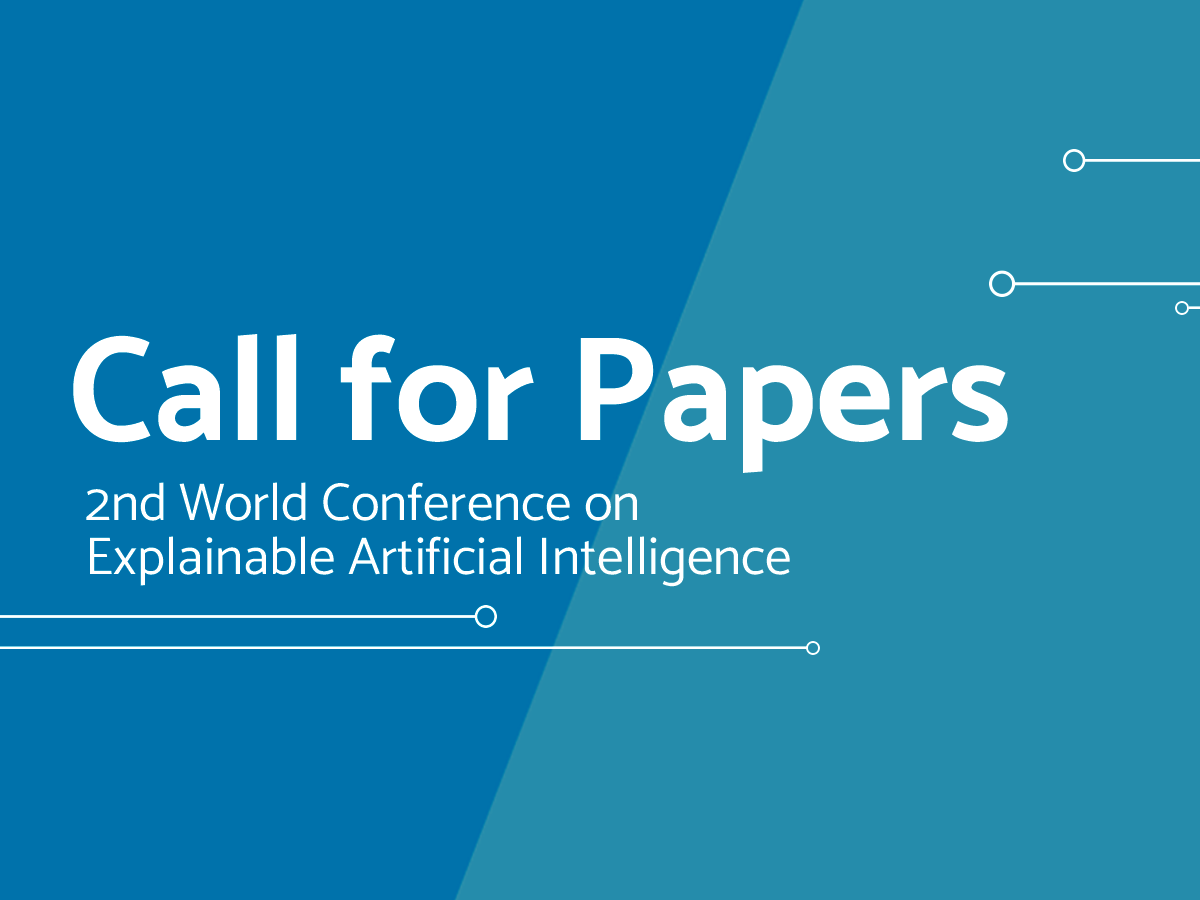
Call for XAI-Papers!
Two research groups associated with BIFOLD take part in the organization of the 2nd World Conference on Explainable Artificial Intelligence. Each group is hosting a special track and has already published a Call for Papers. Researchers are encouraged to submit their papers by March 5th, 2024.
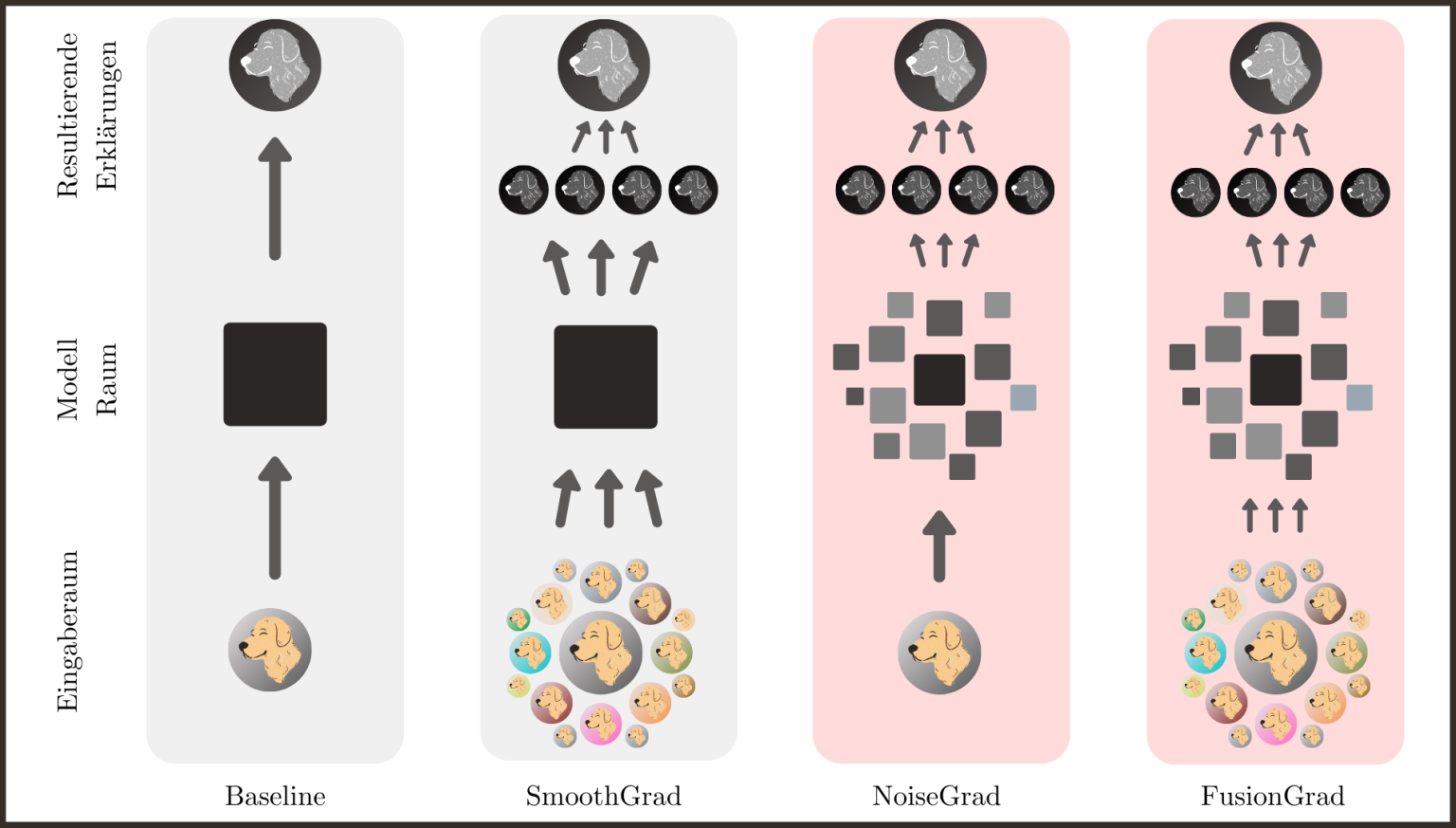
Shining a light into the Black Box of AI Systems
In the paper “NoiseGrad — Enhancing Explanations by Introducing Stochasticity to Model Weights,” to be presented at the 36th AAAI-22 Conference on Artificial Intelligence, a team of researchers, among them BIFOLD researchers Dr. Marina Höhne, Shinichi Nakajima, PhD, and Kirill Bykov, propose new methods to reduce visual diffusion of the different explanation methods, which have shown to make existing explanation methods more robust and reliable.
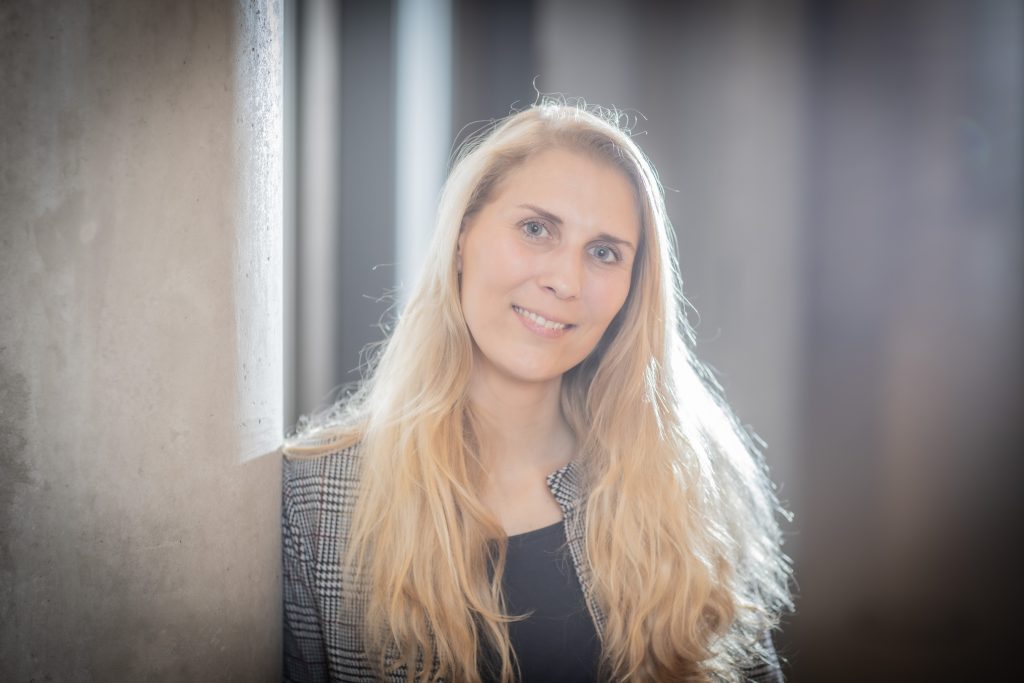
Intelligent machines also need control
Dr. Marina Höhne, BIFOLD Junior Fellow, was awarded two million euros funding by the German Federal Ministry of Education and Research to establish a research group working on explainable artificial intelligence.