This project focuses on the development of new methods to bring Explainable AI to time series data and time series predictions. It investigates in particular the specificities of real-world time series, such as uncertainty or missing values, and aims to develop Explainable AI methods that produce more informative explanations in such contexts. It also investigates how time series predictions are best explained to a human user, for example, using methods that enable an interpretation beyond the temporal domain, e.g. in terms of frequencies.
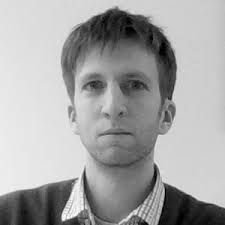
Research Group Lead
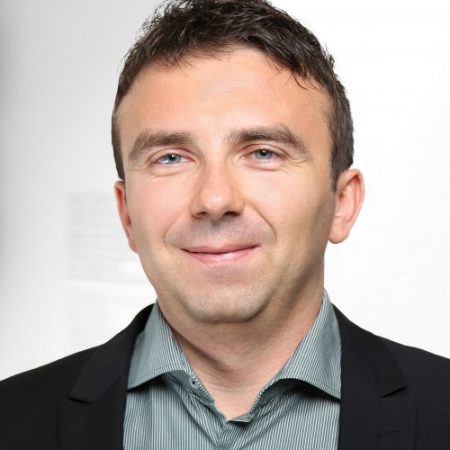
Fellow
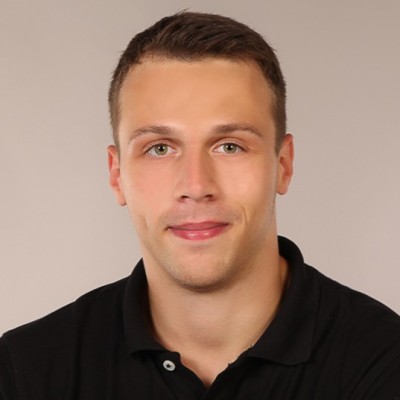
Doctoral Researcher