A Deep Reinforcement Learning framework, developed by one of BIFOLD’s directors, Prof. Dr. Klaus-Robert Müller, and his colleagues at the Department of Brain and Cognitive Engineering of the Korea University in Seoul, enabled the robot “Curly” to beat top-level athletes in the Olympic sport of curling. The work was recently featured in Nature Research Highlights.
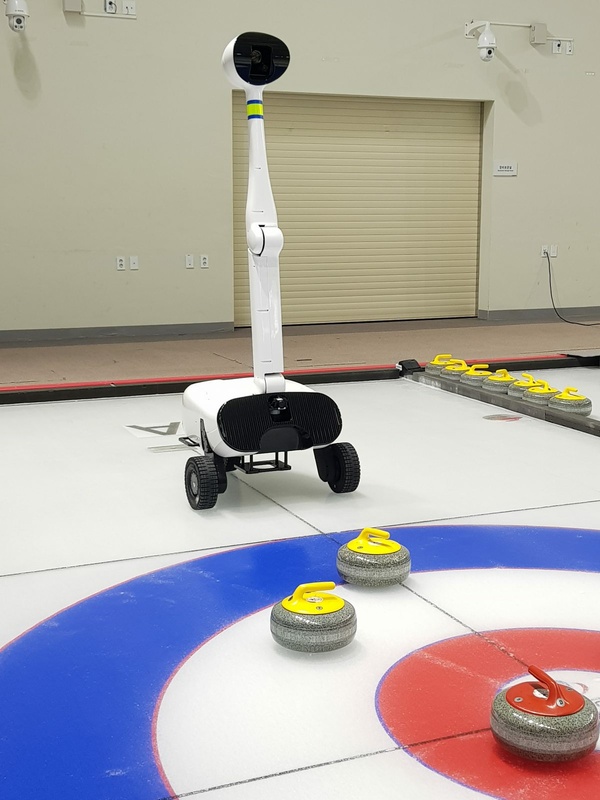
The sport of curling is a good testbed for the performance of AI in the real-world. On the one hand, the ice field on which the curling is performed is not overly complex and on the other hand, the conditions change constantly during the game. Also, the game’s timing rules do not allow for relearning while playing. Prof. Dr. Klaus-Robert Müller (Full Professor and Chair of the Machine Learning group at TU Berlin and Distinguished Professor at Korea University Seoul), Prof. Dr. Dong-Ok Won and Prof. Dr. Seong-Whan Lee (both Korea University of Seoul) met the challenge by designing an adaptive Reinforcement Learning framework which uses temporal features to deal with the uncertainties of the game.
All strategic decisions, planning, estimations in the synchronization between AI agents and robot control must be carried out not only in real time, but also under high uncertainties. At the same time, the data available to train the deep learning network is very limited. All in all, a huge challenge for modern AI.
Prof. Dr. Klaus-Robert Müller
Based on this framework, the robot “Curly” was able to beat top-level human curling players in three out of four games, after a short calibration phase. This human-like sports performance is an early but important step in physics-based real-world application of AI robots. The work was published in Robotics Science Vol. 5, Issue 46 and recently featured in Natur Research Highlights.
More information is available in the official press release of TU Berlin.
THE PAPER IN DETAIL:
>
Authors: Dong-Ok Won, Klaus-Robert Müller, Seong-Whan Lee
Abstract:
The game of curling can be considered a good test bed for studying the interaction between artificial intelligence systems and the real world. In curling, the environmental characteristics change at every moment, and every throw has an impact on the outcome of the match. Furthermore, there is no time for relearning during a curling match due to the timing rules of the game. Here, we report a curling robot that can achieve human-level performance in the game of curling using an adaptive deep reinforcement learning framework. Our proposed adaptation framework extends standard deep reinforcement learning using temporal features, which learn to compensate for the uncertainties and nonstationarities that are an unavoidable part of curling. Our curling robot, Curly, was able to win three of four official matches against expert human teams [top-ranked women’s curling teams and Korea national wheelchair curling team (reserve team)]. These results indicate that the gap between physics-based simulators and the real world can be narrowed.
Published in: Science Robotics 23 Sep 2020: Vol. 5, Issue 46, eabb9764
Authors: Dong-Ok Won, Klaus-Robert Müller, Seong-Whan Lee
Abstract:
The game of curling can be considered a good test bed for studying the interaction between artificial intelligence systems and the real world. In curling, the environmental characteristics change at every moment, and every throw has an impact on the outcome of the match. Furthermore, there is no time for relearning during a curling match due to the timing rules of the game. Here, we report a curling robot that can achieve human-level performance in the game of curling using an adaptive deep reinforcement learning framework. Our proposed adaptation framework extends standard deep reinforcement learning using temporal features, which learn to compensate for the uncertainties and nonstationarities that are an unavoidable part of curling. Our curling robot, Curly, was able to win three of four official matches against expert human teams [top-ranked women’s curling teams and Korea national wheelchair curling team (reserve team)]. These results indicate that the gap between physics-based simulators and the real world can be narrowed.
Published in: Science Robotics 23 Sep 2020: Vol. 5, Issue 46, eabb9764