Global optimization is a crucial endeavor in the development of new materials, demanding significant computational resources. In order to tackle this challenge, we efficiently select promising candidate structures for optimization, minimizing the required steps in the process. This is achieved through the utilization of neural network force fields equipped with uncertainty estimation, which iteratively guide a pool of randomly generated candidates towards their respective local minima. By employing this approach, we are able to effectively identify the most promising candidates for further evaluation using density functional theory (DFT), while simultaneously reducing the number of relaxation steps necessary.
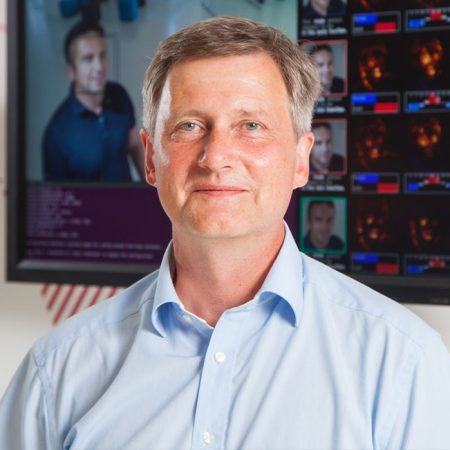
Director
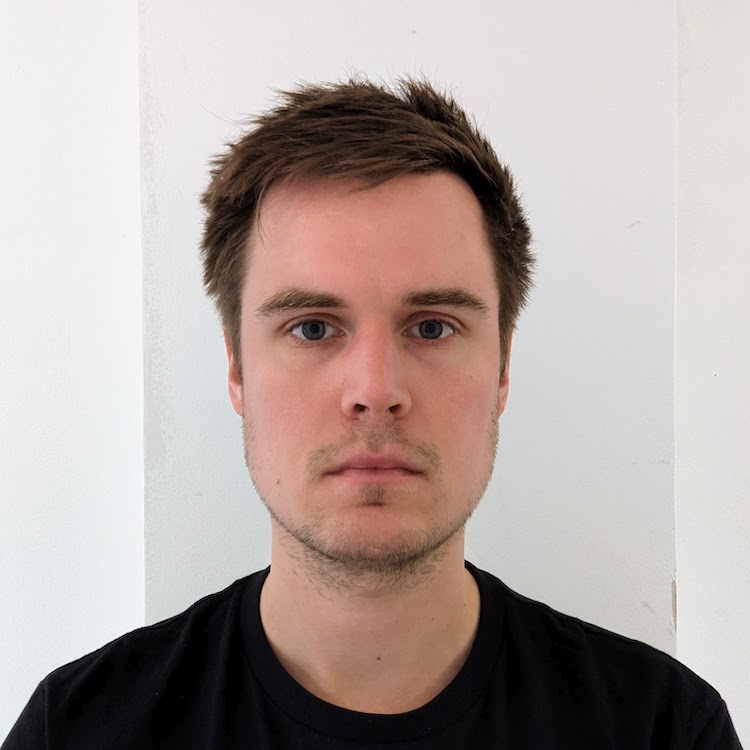
Doctoral Researcher