With advances in satellite technology, Earth observation (EO) data archives are continuously growing with high speed, delivering an unprecedented amount of data on the state of our planet over time. For example, via the Copernicus Programme (the European flagship satellite initiative in EO), Sentinel satellites acquire roughly 12TB of satellite images per day, and the total size of the Copernicus data archives is more than 30PB. The “big EO data” is a great source of information that is relevant for several varying EO applications (e.g., climate change analysis, urban area studies, forestry applications, risk and damage assessment, water quality assessment, crop monitoring). In this project, we develop the data engineering techniques to prepare, process, and store EO data. To this end, we identify suitable storage and data processing systems, considering the high spectral and temporal resolution of EO data with different data modalities (e.g., multispectral, hyperspectral, SAR).
The repeat-pass nature of satellites orbiting the Earth generate data with great speed (i.e., high temporal resolution). Accordingly, the need for the continual learning of new tasks (e.g., new land-cover land-use classes) from a stream of recently acquired EO data, without forgetting previously learned knowledge (a.k.a. catastrophic forgetting) has become increasingly important. To address this issue, in this project we also research continual learning (a.k.a. lifelong learning). In detail, we analyze which tasks associated with the EO data analysis suffer from catastrophic forgetting over lifelong learning. Then, to achieve efficient and effective continual learning for these tasks, we develop methods that: (i) prevent catastrophic forgetting effectively, (ii) are highly scalable to a large number of tasks, (iii) are computation and memory efficient, and (iv) are highly generalizable to previously unseen EO data and the related land-use and land-cover classes.
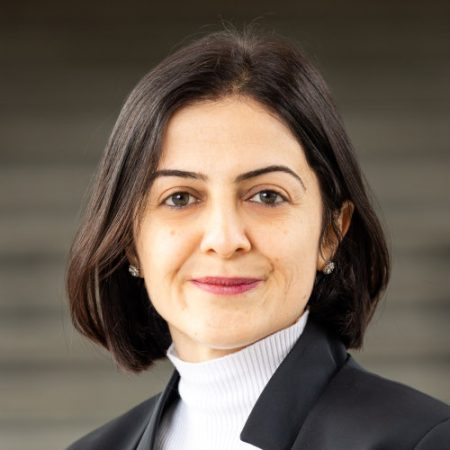
Research Group Lead
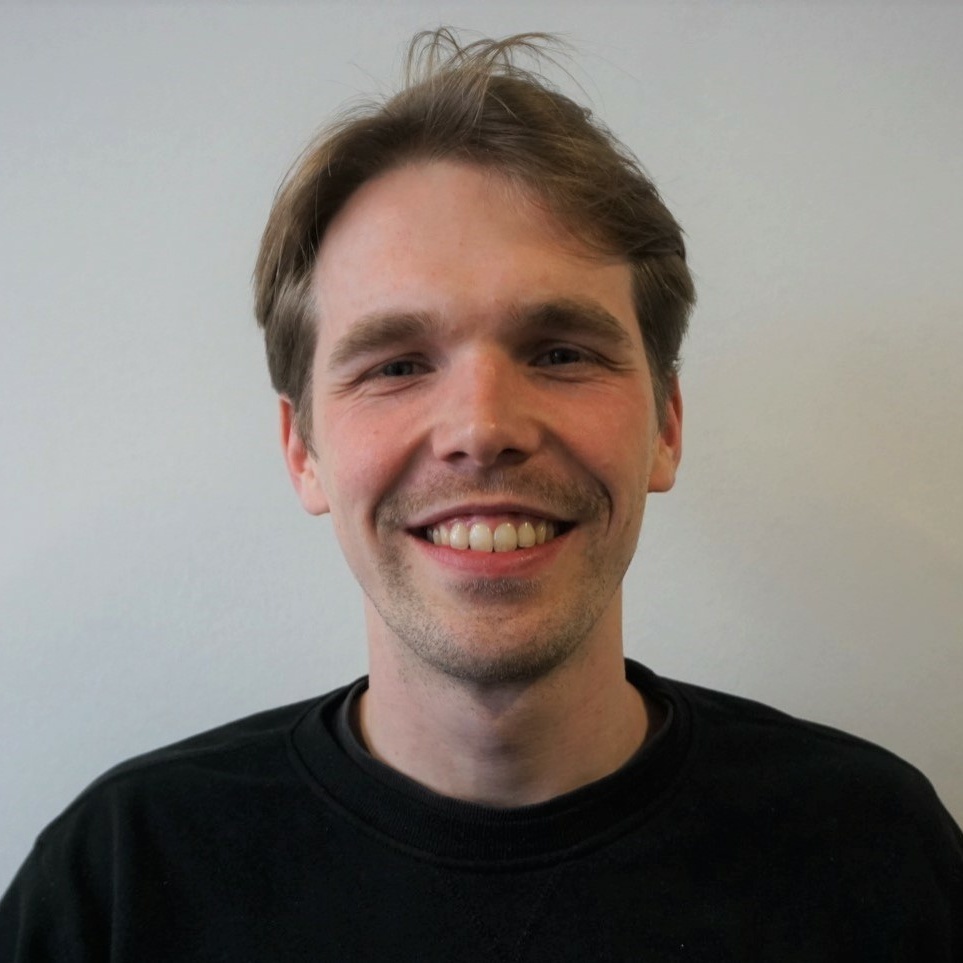
Doctoral Researcher