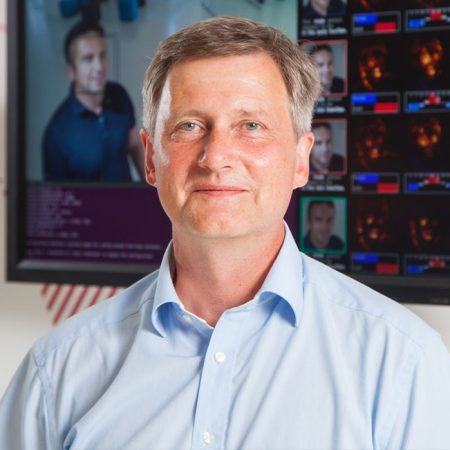
Klaus-Robert Müller, professor of Machine Learning at TU Berlin and Co-Director of the Berlin Institute for the Foundations of Learning and Data (BIFOLD), discusses computation time as a climate killer and his predictions for science in 80 years.
Professor Müller, in our conversation prior to this interview about your vision for the future of the computer on the 80th anniversary of the invention of the Z3, you mentioned energy conservation as one of the major challenges we face. Why is this?
The world’s computer centers are major emitters of CO2. Huge amounts of fossil energy are still being used to power them. More and more calculations are performed, and the computation time required for these is increasing. It is not enough for us to go on Fridays for Future marches. We all have to try to do something in the areas where we have direct influence.
So, the work of your research group focuses directly on this topic?
Yes, but even more so our research at the Berlin Institute for the Foundations of Learning and Data, or BIFOLD for short, which was set up in 2020 as a part of the federal government’s AI strategy.
Where do you see possible solutions to significantly reduce the energy consumption of computer centers?
Solving a known image recognition problem uses about as much energy as a four-person household over a period of three months. One approach is to save computation time by using a different mathematical method. This could reduce energy consumption to the level of a four-person household for two months while achieving the same result. A greater saving would of course be better. We need to develop energy-saving methods of computing for AI. Data traffic for distributed learning requires a great deal of energy, so we are also looking to minimize this. My team has been able to demonstrate how smart mathematical solutions can reduce the requirement for data transfer from 40 terabytes to 5 or 6 gigabytes. Getting a good result is no longer the only issue; how you achieve that result is becoming increasingly important.
What for you were the most important milestones in the development of the computer over the past 80 years?
For me, it all began with Konrad Zuse and the Z3. I am fascinated how this computer with its three arithmetic calculations and a memory of just 64 words was able to give rise to the supercomputer. In the 1950s and 60s, some people were still able to perform calculations faster than computers. At the beginning of the 90s, around the time I received my doctorate, the first workstations became available. These marked the end of the time that you had to to log on to a mainframe computer. In 1994 while working as a postdoc in the USA, I had the opportunity to perform calculations using such a supercomputer, the Connection Machine CM5. The most recent major step are graphics processing units, or GPUs for short. These graphic processors not only allow you to have a mini supercomputer at your daily disposal for a small cost, their architecture also makes them ideal for machine learning and training large neural network models. This has led to many new scientific developments, which today form part of our lives. It really fascinates me how we have progressed in such a short time from a situation where people could perform calculations faster than a computer to today where I have a supercomputer under my desk. Although supercomputers aren’t everything.
How do you mean?
Three decades ago, I published a paper with another student on the properties of a neural network. There was another researcher working on the same topic who, unlike us, had access to the Cray supercomputer. We had to do perform our calculations using a work station. Well, we adapted our algorithm to this hardware and were able to achieve the same results using a simple computer as our colleague with access to the Cray XMP. This was greeted with amazement in our field. What I am getting at is that you can sometimes achieve good results with simpler equipment if you use a little more creativity.
This year marks the 80th anniversary of the invention of the computer. Are you able to predict what may be possible in the area of machine learning, in other words your area of research, within the next 80 years?
What I would say is that machine learning will become a standard tool in industry and science, for the humanities as well as natural sciences and medicine. To make this happen, we have to now train a generation of researchers to not only use these tools but also understand their underlying principles so as to prevent improper use of machine learning and thus false scientific findings. This includes an understanding of big data, as the data volumes required in science are becoming ever larger. These two areas – machine learning and big data – will become more and more closely connected with each other as well as with their areas of application. And this brings me back to BIFOLD: We see both areas as a single entity linked to its applications and it is precisely on this basis that we have now started to train a new generation of researchers.
Interview: Sybille Nitsche