Modern algorithms open up new possibilities for historians
In the past, scholars used to pore over dusty tomes. Today Dr. Matteo Valleriani, group leader at the Max Planck Institute for the History of Science as well as honorary professor at TU Berlin and fellow at the Berlin Institute for the Foundations of Learning and Data (BIFOLD), uses algorithms to group and analyze digitized data from historical works. The term used to describe this process is computational history. One of the goals of Valleriani’s research is to unlock the mechanisms involved in the homogenization of cosmological knowledge in the context of studies in the history of science.
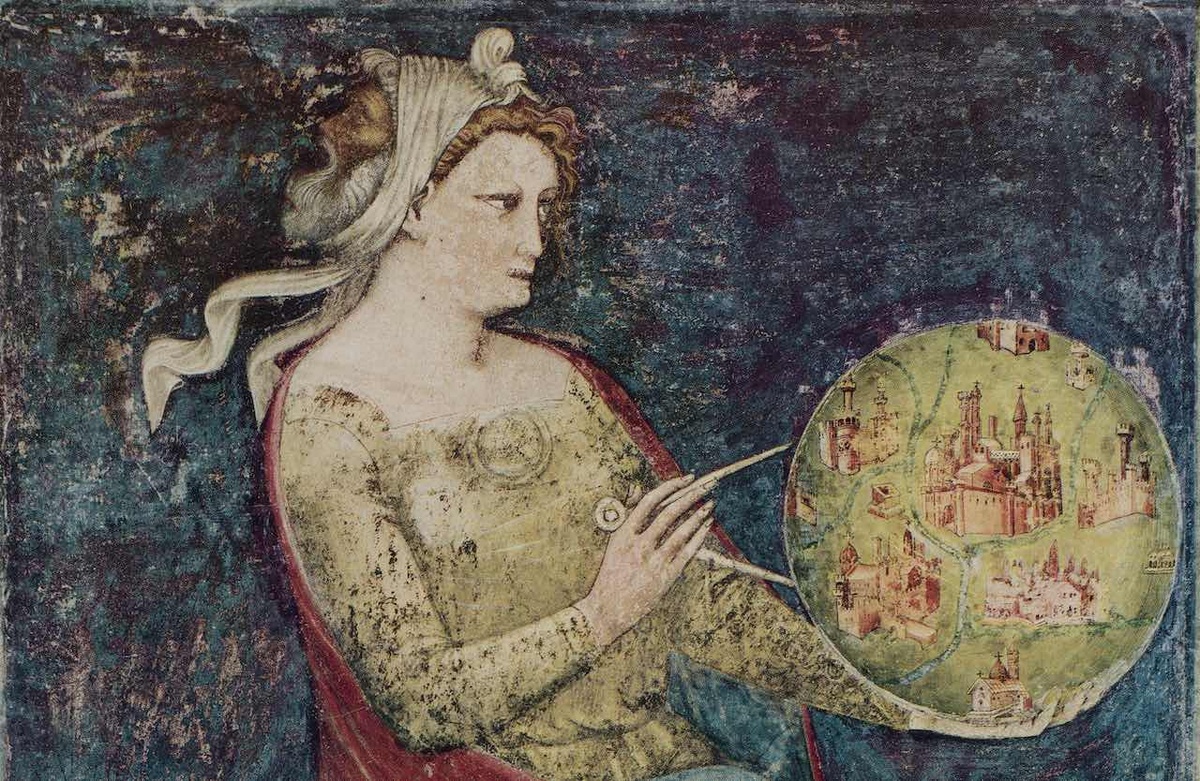
The project is co-financed by BIFOLD and researches the evolutionary path of the European scientific system as well as the establishment of a common scientific identity in Europe between the 13th and 17th centuries. Dr. Valleriani is working with fellow researchers from the Max Planck Institute for the Physics of Complex Systems to develop and implement empirical, multilayer networks to enable the analysis of huge quantities of data.
In Paris in the first half of the 13th century, Johannes de Sacrobosco compiled an elementary text on geocentric cosmology entitled Tractatus de sphaera. This manuscript is a simple, late medieval description of the geocentric cosmos based on a synthesis of Aristotelian and Ptolemaic worldviews.
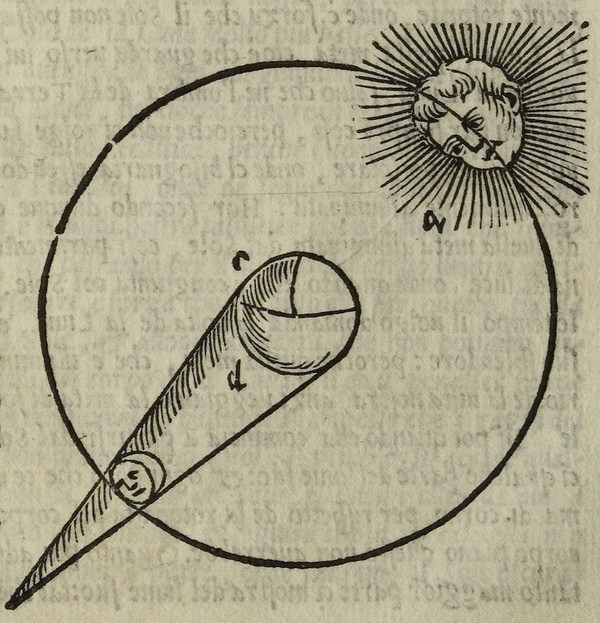
“This compilation of the knowledge of its time is the result of an emerging intellectual interest in Europe. In the 13th century, a need arose for a knowledge of astronomy and cosmology on a qualitative and descriptive basis – parallel to and driven by the emergence of a network of new universities,” explains Valleriani. Over the following decades, the Tractatus de sphaera was commented on, extended, and revised many times, but continued to be a mandatory text at all European universities until the 17th century. Digitized copies of 359 printed textbooks featuring modified forms of the Tractatus de sphaera from the period 1472 until 1650 are now available to researchers. During this period of about 180 years, some 30 new universities were founded in Europe.
The universal language of scholars at that time was Latin, which contributed significantly to the high mobility of knowledge even in this period. “An introductory course in astronomy was mandatory for students in Europe at that time,” explains Valleriani. “As a committed European, I am mainly interested in how this led to the emergence of a shared scientific knowledge in Europe.”
Taken together, these 359 books contain some 74,000 pages – a quantity of text and images that it is not possible for any individual person to examine and analyze. Working with machine learning experts from BIFOLD, the research team first had to clean, sort, and standardize this colossal data corpus drawn from a wide range of digital sources to make it accessible for algorithms. The first step was to sort the data into texts, images, and tables. The texts were then broken down into recurring textual parts and organized according to a specific semantic taxonomy reflecting early modern modes of production of scientific knowledge.
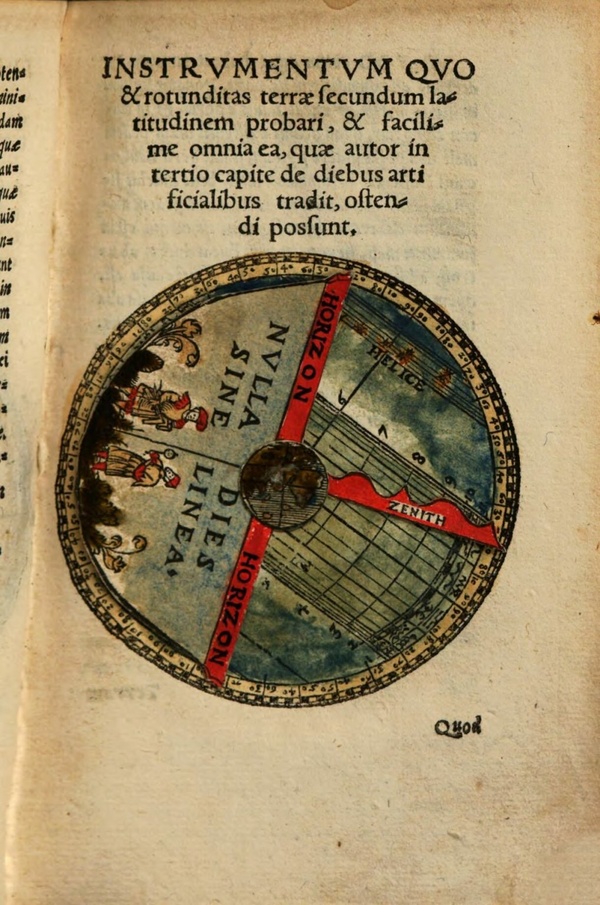
Each of the more than 20,000 scientific illustrations had to be linked to the extensive metadata of the editions and their textual parts. In addition, more than 11,000 tables were identified in the Sphaera corpus. “To analyze the tables, we developed an algorithm to divide them into several groups with similar characteristics. This allows us to now use further analyses to compare these groups with each other,” explains Valleriani. This process may sound simple, but in fact involves countless technical difficulties: “Developing suitable algorithms is made more difficult by four error sources. The books from this period contain many printer errors. This and the fact that the conditions of the books vary greatly makes them at times hard to digitize. Then there is the problem of the differing quality of the electronic copies. We also have to remember that at that time every printer used their own typeface, meaning that our algorithms have to be effectively trained for each printer to be able to even recognize the data.” In order to track the transformation process of the original text in the 359 books dating from this 180-year period and formalize this process of knowledge, the researchers need to understand precisely how knowledge changed, ultimately becoming more and more homogenous.
“To achieve an understanding based upon data requires an intelligent synthesis of machine learning and the working practices of historians. The algorithms which we will now publish are the first capable of analyzing such data. We are also looking forward to develop further algorithms as part of our continuing cooperation with BIFOLD,” Valleriani explains.
>
Authors:
Oliver Eberle, Jochen Büttner, Florian Kräutli, Klaus-Robert Müller, Matteo Valleriani, Grégoire Montavon
Abstract:
Many learning algorithms such as kernel machines, nearest neighbors, clustering, or anomaly detection, are based ondistances or similarities. Before similarities are used for training an actual machine learning model, we would like to verify that they arebound to meaningful patterns in the data. In this paper, we propose to make similarities interpretable by augmenting them with anexplanation. We develop BiLRP, a scalable and theoretically founded method to systematically decompose the output of an alreadytrained deep similarity model on pairs of input features. Our method can be expressed as a composition of LRP explanations, whichwere shown in previous works to scale to highly nonlinear models. Through an extensive set of experiments, we demonstrate thatBiLRP robustly explains complex similarity models, e.g. built on VGG-16 deep neural network features. Additionally, we apply ourmethod to an open problem in digital humanities: detailed assessment of similarity between historical documents such as astronomicaltables. Here again, BiLRP provides insight and brings verifiability into a highly engineered and problem-specific similarity model.
Publication:
IEEE Transactions on Pattern Analysis and Machine Intelligence
Authors:
Oliver Eberle, Jochen Büttner, Florian Kräutli, Klaus-Robert Müller, Matteo Valleriani, Grégoire Montavon
Abstract:
Many learning algorithms such as kernel machines, nearest neighbors, clustering, or anomaly detection, are based ondistances or similarities. Before similarities are used for training an actual machine learning model, we would like to verify that they arebound to meaningful patterns in the data. In this paper, we propose to make similarities interpretable by augmenting them with anexplanation. We develop BiLRP, a scalable and theoretically founded method to systematically decompose the output of an alreadytrained deep similarity model on pairs of input features. Our method can be expressed as a composition of LRP explanations, whichwere shown in previous works to scale to highly nonlinear models. Through an extensive set of experiments, we demonstrate thatBiLRP robustly explains complex similarity models, e.g. built on VGG-16 deep neural network features. Additionally, we apply ourmethod to an open problem in digital humanities: detailed assessment of similarity between historical documents such as astronomicaltables. Here again, BiLRP provides insight and brings verifiability into a highly engineered and problem-specific similarity model.
Publication:
IEEE Transactions on Pattern Analysis and Machine Intelligence
>
Authors:
Maryam Zamani, Alejandro Tejedor, Malte Vogl, Florian Kräutli, Matteo Valleriani, Holger Kantz
Abstract:
We investigated the evolution and transformation of scientific knowledge in the early modern period, analyzing more than 350 different editions of textbooks used for teaching astronomy in European universities from the late fifteenth century to mid-seventeenth century. These historical sources constitute the Sphaera Corpus. By examining different semantic relations among individual parts of each edition on record, we built a multiplex network consisting of six layers, as well as the aggregated network built from the superposition of all the layers. The network analysis reveals the emergence of five different communities. The contribution of each layer in shaping the communities and the properties of each community are studied. The most influential books in the corpus are found by calculating the average age of all the out-going and in-coming links for each book. A small group of editions is identified as a transmitter of knowledge as they bridge past knowledge to the future through a long temporal interval. Our analysis, moreover, identifies the most impactful editions. These books introduce new knowledge that is then adopted by almost all the books published afterwards until the end of the whole period of study. The historical research on the content of the identified books, as an empirical test, finally corroborates the results of all our analyses.
Publication:
Scientific Reports – Nature
Authors:
Maryam Zamani, Alejandro Tejedor, Malte Vogl, Florian Kräutli, Matteo Valleriani, Holger Kantz
Abstract:
We investigated the evolution and transformation of scientific knowledge in the early modern period, analyzing more than 350 different editions of textbooks used for teaching astronomy in European universities from the late fifteenth century to mid-seventeenth century. These historical sources constitute the Sphaera Corpus. By examining different semantic relations among individual parts of each edition on record, we built a multiplex network consisting of six layers, as well as the aggregated network built from the superposition of all the layers. The network analysis reveals the emergence of five different communities. The contribution of each layer in shaping the communities and the properties of each community are studied. The most influential books in the corpus are found by calculating the average age of all the out-going and in-coming links for each book. A small group of editions is identified as a transmitter of knowledge as they bridge past knowledge to the future through a long temporal interval. Our analysis, moreover, identifies the most impactful editions. These books introduce new knowledge that is then adopted by almost all the books published afterwards until the end of the whole period of study. The historical research on the content of the identified books, as an empirical test, finally corroborates the results of all our analyses.
Publication:
Scientific Reports – Nature