Whether in medicine, battery research, or materials science, researchers everywhere are seeking innovative substances. In the process, they can often predict the desired chemical and physical properties in great detail, right down to atomic level. However, the range of all potential chemical compounds alone is so vast that it would take years to find the appropriate substance. An interdisciplinary research group at the Berlin Institute for the Foundations of Learning and Data (BIFOLD) at Technische Universität Berlin has now developed an algorithm which uses AI to implement inverse chemical design and thus generates targeted molecules based on their desired properties. The research group’s publication titled “Inverse design of 3d molecular structures with conditional generative neural networks” has now been published in the renowned journal Nature Communications.
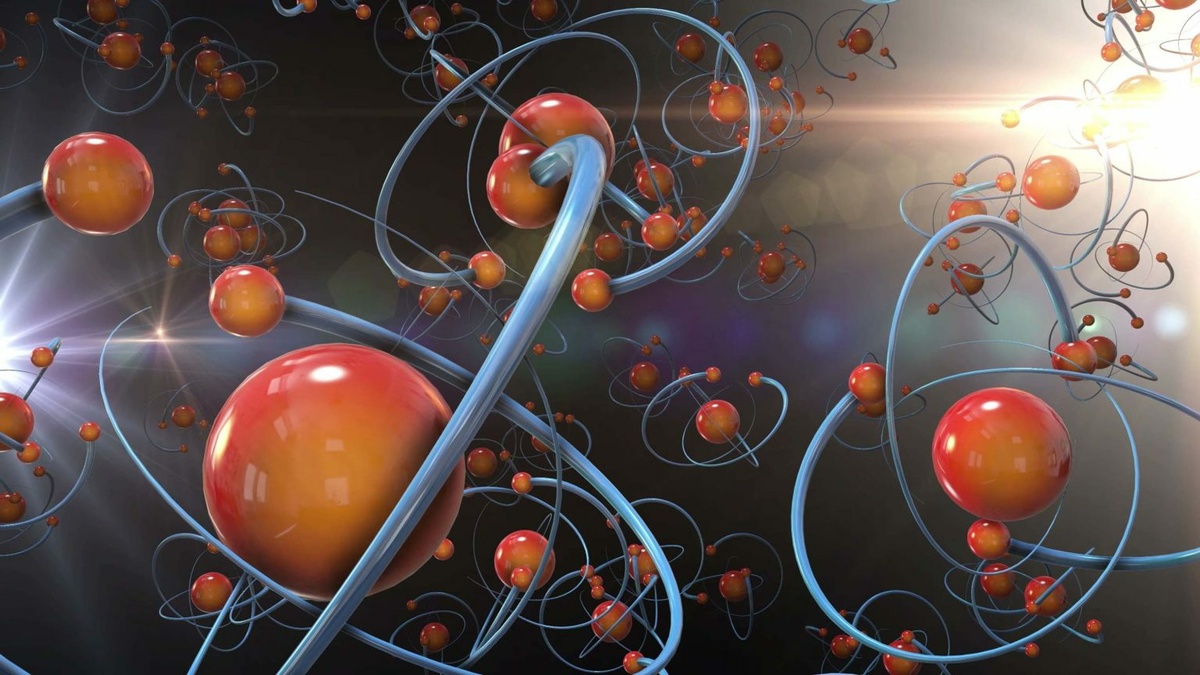
The search for suitable molecules for specific medical or industrial applications is an extremely complex and expensive process. “Hypothetically, there are an incredible number of possible structures. However, only a tiny fraction possesses the specific chemical or physical properties required for a particular application,” explains Dr. Kristof Schütt, BIFOLD Junior Fellow at TU Berlin. A wealth of methods has been developed in recent years capable of predicting the chemical properties and energetic states of given substances using AI. But even using these efficient methods, the search for molecules with specific properties has proven difficult in practice, as it is still necessary to search through an overwhelming number of candidates.
Reversing the Structure-Property Relationship
Consequently, the research group at BIFOLD is concentrating on what is known as inverse molecular design, where the structure-property relationship is reversed. Instead of the structure defining the properties, it is the properties that define the structure. The challenge consists in directly constructing molecular structures that correspond to a given set of properties. The AI algorithm is based on a deep generative neural network, incorporating prior knowledge of basic, physical conditions. The network only uses a few thousand sample molecules to learn the complex relationships between chemical structures and their properties. “The user can then specify various property values, and the generative neural network suggests a manageable number of suitable molecules and compounds. Only these candidates have to be investigated by the chemists,” explains Schütt. The researchers have been able to show that inverse chemical design also functions when the desired property values are only partly covered by the known sample of molecules.
The interdisciplinary research team expects that such algorithms, used in concert with other AI-driven approaches and quantum chemical methods, can greatly accelerate the search for new molecules and materials in many practical areas. Klaus-Robert Müller, BIFOLD co-director and professor of machine learning at TU Berlin, adds: “I see enormous potential here if both the design of the molecules and their analysis and simulation are supported by artificial intelligence methods. This could help in the development of drugs, for example, or accelerate the search for novel materials for batteries and solar cells.”
The publication in detail:
Niklas W. A. Gebauer, Michael Gastegger, Stefan S. P. Hessmann, Klaus-Robert Müller and Kristof T. Schütt: “Inverse design of 3d molecular structures with conditional generative neural networks”, Nat Commun 13, 973 (2022)
>
The rational design of molecules with desired properties is a long-standing challenge in chemistry. Generative neural networks have emerged as a powerful approach to sample novel molecules from a learned distribution. Here, we propose a conditional generative neural network for 3d molecular structures with specified chemical and structural properties. This approach is agnostic to chemical bonding and enables targeted sampling of novel molecules from conditional distributions, even in domains where reference calculations are sparse. We demonstrate the utility of our method for inverse design by generating molecules with specified motifs or composition, discovering particularly stable molecules, and jointly targeting multiple electronic properties beyond the training regime.
The rational design of molecules with desired properties is a long-standing challenge in chemistry. Generative neural networks have emerged as a powerful approach to sample novel molecules from a learned distribution. Here, we propose a conditional generative neural network for 3d molecular structures with specified chemical and structural properties. This approach is agnostic to chemical bonding and enables targeted sampling of novel molecules from conditional distributions, even in domains where reference calculations are sparse. We demonstrate the utility of our method for inverse design by generating molecules with specified motifs or composition, discovering particularly stable molecules, and jointly targeting multiple electronic properties beyond the training regime.