"CorDeep" - a new web application for historians
The ever-increasing amount of digitized historical works means that historians are, more than ever, in need of digital tools to process and extract information from electronic copies of historical sources. The methods and approaches to extract text and images from scanned historical documents are numerous, however, they remain out of reach of the majority of the historical community due to their technical requirements and sophistication.
BIFOLD scientists in collaboration with the Max Planck Institute for the History of Science, developed and trained YOLO (You Only Look Once), an object detection model able to detect four different classes of visual elements within historical sources from the 15th to the 17th centuries. They relied on a ground truth dataset of almost 30.000 visual elements – printed on more than 76.000 pages – of collected data within the project “The Sphere: Knowledge System Evolution and the Shared Scientific Identity of Europe” (https://sphaera.mpiwg-berlin.mpg.de/).
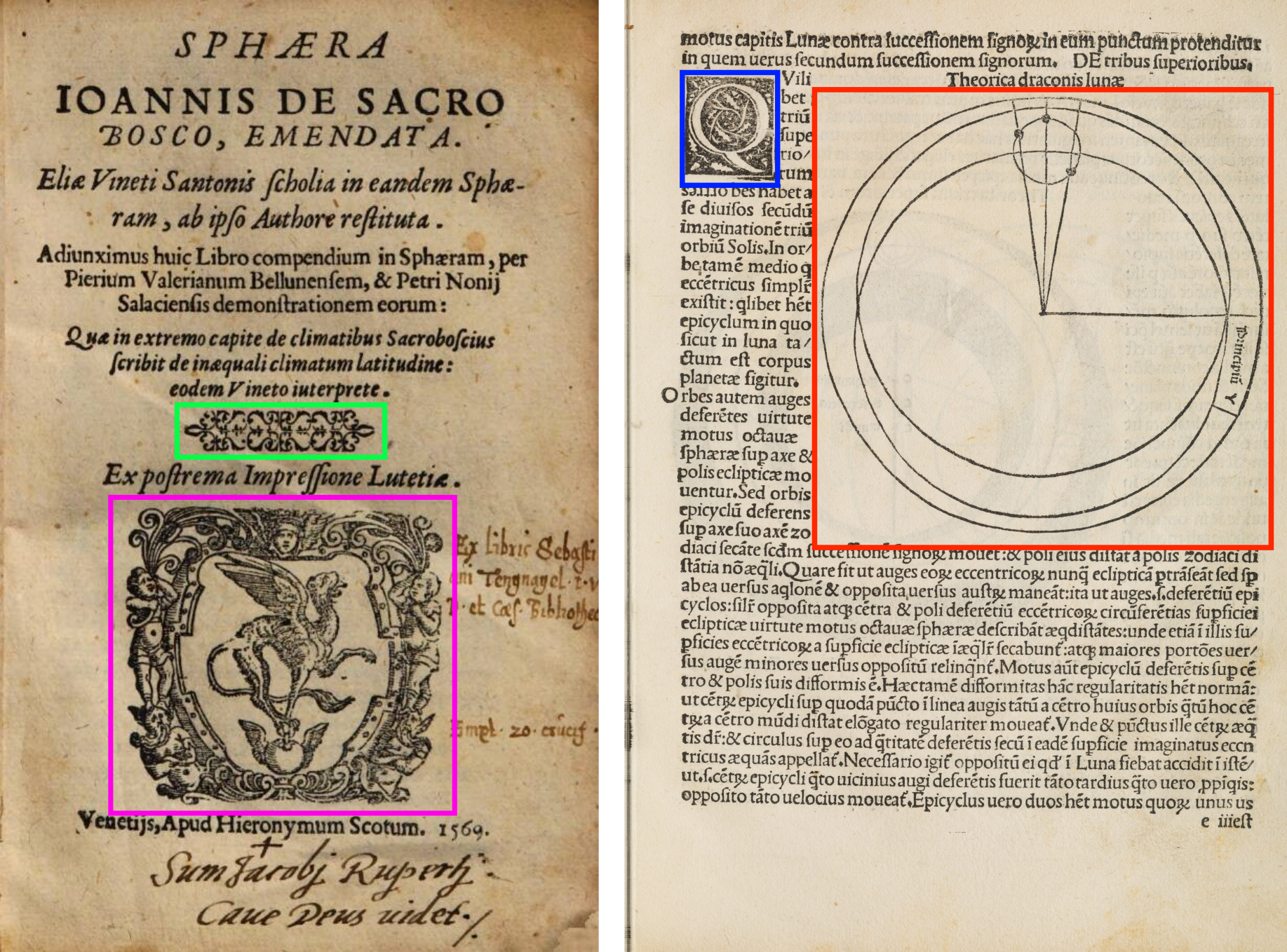
These four different classes represent the four main types of visual elements that one can encounter in a historical source of the above-mentioned period. The most abundant of these classes is the content illustration class, which is an element inserted in and around the text to explain, enrich, describe or criticize the textual content. The texts themselves could contains initials, which are small visual elements, often highly decorated, that represent a letter at the beginning of certain paragraphs, while decorations represent little decorative items often marking the beginning or end of a paragraph or chapter. Initials and Decorations are often indicative of the printers who produced the sources, when these are not identifiable from the sources themselves. Finally, printer’s marks represent emblems and insignia of the printers and are often placed at the beginning or end of the book. These four classes, detected by our model, capture the diverse nature of visual elements in these historical sources and can help researchers explore their content from a visual medium perspective.
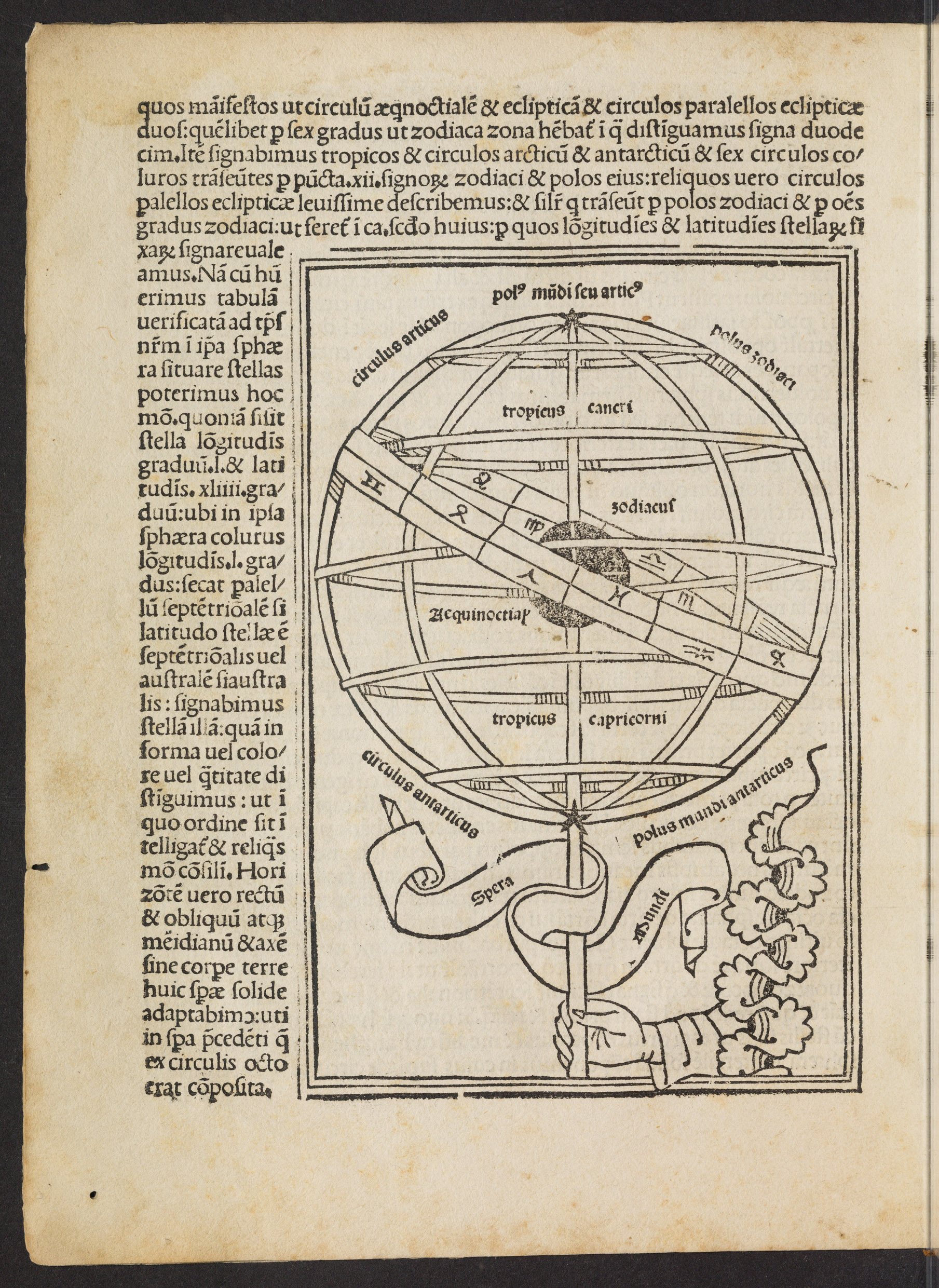
The YOLO model, trained on the hand curated dataset, outperforms competitors in the area of visual element detection in historical sources; which makes it a great tool for the modern historian. To help other researchers build on these results, the scientists share the code and the curated dataset here: ZENODO. Since computer skills are one of the major barriers towards the uptake of ML approaches within the humanities, they created a web-service called CorDeep, to emphasize its intended use case, namely corpus analysis, and the Deep Learning methods it is based on. Users of this service can, without writing any line of code, simply drag and drop the scanned version of their historical documents (JPG, PNG, IIIF Manifest) into the web-service interface and recieve the extracted and labelled images within a short period of time.
The use of Deep Learning algorithms comes with a relatively large carbon footprint. To ensure that CorDeep users are aware of their carbon footprint, the scientists implemented the CO2 emission estimation per uploaded document. In the long run, the web-service will be a sustainable endeavor by reducing the need for other researchers working in the same field to develop and train their own bespoke models.
Try out the digital CorDeep platform here.
The publication in detail:
Büttner, Jochen, Julius Martinetz, Hassan El-Hajj, and Matteo Valleriani. 2022. "CorDeep and the Sacrobosco Dataset: Detection of Visual Elements in Historical Documents" Journal of Imaging 8, no. 10: 285.