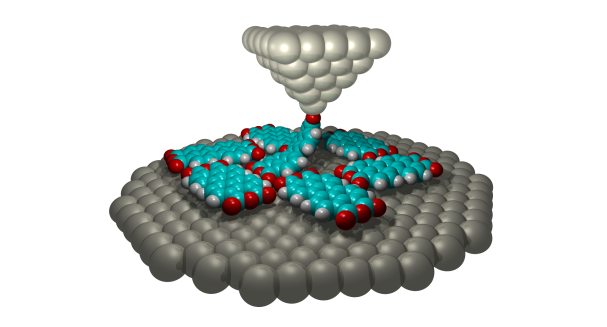
In a cooperation between the Machine Learning group at TU Berlin, led by BIFOLD Co-Director Prof. Dr. Klaus-Robert Müller and Jülich’s Quantum Nanoscience institute, led by Prof. Dr. Stefan Tautz, researchers enabled a robot to selectively grip and move single molecules from a layer, by applying reinforced learning. To achieve this, they let the machine learn in its real environment and a model in parallel. The corresponding paper was published in “Science Advances”.
“This is the first time ever that we have succeeded in bringing together artificial intelligence and nanotechnology,” emphasizes Klaus-Robert Müller. “Up until now, this has only been a ‘proof of principle’,” Stefan Tautz adds. “However, we are confident that our work will pave the way for the robot-assisted automated construction of functional supramolecular structures, such as molecular transistors, memory cells, or qubits – with a speed, precision, and reliability far in excess of what is currently possible.”
The Falling Walls Foundations honored this work as a scientific breakthrough by announcing Prof. Dr. Stefan Tautz as a winner in the category “Engineering and Technology” during the Berlin Science Week / Falling Walls event in November 2020!
Watch Prof. Tautz’ talk at the Berlin Science Week Falling Walls event on YouTube.
To learn more please visit the official press release of TU Berlin.
THE PAPER IN DETAIL:
>
Authors: Philipp Leinen, Malte Esders, Kristof T. Schütt, Christian Wagner, Klaus-Robert Müller, F. Stefan Tautz
Abstract:
The ability to handle single molecules as effectively as macroscopic building blocks would enable the construction of complex supramolecular structures inaccessible to self-assembly. The fundamental challenges obstructing this goal are the uncontrolled variability and poor observability of atomic-scale conformations. Here, we present a strategy to work around both obstacles and demonstrate autonomous robotic nanofabrication by manipulating single molecules. Our approach uses reinforcement learning (RL), which finds solution strategies even in the face of large uncertainty and sparse feedback. We demonstrate the potential of our RL approach by removing molecules autonomously with a scanning probe microscope from a supramolecular structure. Our RL agent reaches an excellent performance, enabling us to automate a task that previously had to be performed by a human. We anticipate that our work opens the way toward autonomous agents for the robotic construction of functional supramolecular structures with speed, precision, and perseverance beyond our current capabilities.
Published in: Science Advances 02 Sep 2020: Vol. 6, no. 36, eabb6987
Authors: Philipp Leinen, Malte Esders, Kristof T. Schütt, Christian Wagner, Klaus-Robert Müller, F. Stefan Tautz
Abstract:
The ability to handle single molecules as effectively as macroscopic building blocks would enable the construction of complex supramolecular structures inaccessible to self-assembly. The fundamental challenges obstructing this goal are the uncontrolled variability and poor observability of atomic-scale conformations. Here, we present a strategy to work around both obstacles and demonstrate autonomous robotic nanofabrication by manipulating single molecules. Our approach uses reinforcement learning (RL), which finds solution strategies even in the face of large uncertainty and sparse feedback. We demonstrate the potential of our RL approach by removing molecules autonomously with a scanning probe microscope from a supramolecular structure. Our RL agent reaches an excellent performance, enabling us to automate a task that previously had to be performed by a human. We anticipate that our work opens the way toward autonomous agents for the robotic construction of functional supramolecular structures with speed, precision, and perseverance beyond our current capabilities.
Published in: Science Advances 02 Sep 2020: Vol. 6, no. 36, eabb6987