Congratulations to BIFOLD Fellow Prof. Dr. Manfred Hauswirth and BIFOLD Junior Fellow Dr. Danh Le-Phuoc: At the International Semantic Web Conference 2021 (ISWC-2021), their paper “A Native and Adaptive Approach for Unified Processing of Linked Streams and Linked Data” achieved 2nd place for the “SWSA Ten-Year Award” with an honourable mention.
The paper by Danh Le-Phuoc, Minh Dao-Tran, Josiane Xavier Parreira, and Manfred Hauswirth presents one of the first systems for efficient, highly scalable processing of structured, semantic data streams (dynamic data) in combination with background knowledge (static data). The performance of the system was the best among the systems available at the time of the publication in 2011, it has significantly shaped and influenced the research field of semantic data stream processing, and continues to have an impact to the present day. The system makes it possible to easily and efficiently build applications in many fields, e.g. traffic monitoring, environment, Internet-of-things, edge computing, and the smart city.
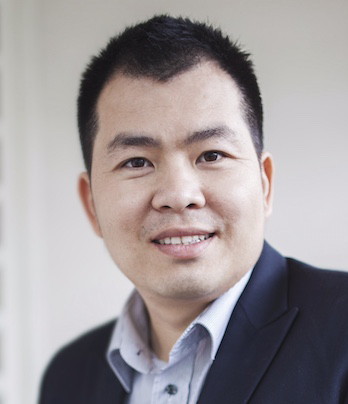
“We are very proud that our paper is recognized by one of the leading conferences on semantic web as one of the most impactful papers. At BIFOLD we continue to work on the CQELS Framework, a semantic stream processing and reasoning framework for developing semantic-driven stream and event processing engines on both edge devices and cloud.”
The International Semantic Web Conference is one of the most important conferences in this research area. Every year at the event, the most influential contributions from the conference proceedings of the last ten years are honored by the Semantic Web Science Association with the “SWSA Ten-Year Award”. The decision is based primarily, but not exclusively, on the number of citations received by the papers from the conference proceedings during the past decade.
The publication in detail:
Danh Le Phuoc, Minh Dao-Tran, Josiane Xavier Parreira, Manfred Hauswirth: A Native and Adaptive Approach for Unified Processing of Linked Streams and Linked Data. ISWC (1) 2011: 370-388
[PDF]
>
In this paper we address the problem of scalable, native and adaptive query processing over Linked Stream Data integrated with Linked Data. Linked Stream Data consists of data generated by stream sources, e.g., sensors, enriched with semantic descriptions, following the standards proposed for Linked Data. This enables the integration of stream data with Linked Data collections and facilitates a wide range of novel applications. Currently available systems use a “black box” approach which delegates the processing to other engines such as stream/event processing engines and SPARQL query processors by translating to their provided languages. As the experimental results described in this paper show, the need for query translation and data transformation, as well as the lack of full control over the query execution, pose major drawbacks in terms of efficiency. To remedy these drawbacks, we present CQELS (Continuous Query Evaluation over Linked Streams), a native and adaptive query processor for unified query processing over Linked Stream Data and Linked Data. In contrast to the existing systems, CQELS uses a “white box” approach and implements the required query operators natively to avoid the overhead and limitations of closed system regimes. CQELS provides a flexible query execution framework with the query processor dynamically adapting to the changes in the input data. During query execution, it continuously reorders operators according to some heuristics to achieve improved query execution in terms of delay and complexity. Moreover, external disk access on large Linked Data collections is reduced with the use of data encoding and caching of intermediate query results. To demonstrate the efficiency of our approach, we present extensive experimental performance evaluations in terms of query execution time, under varied query types, dataset sizes, and number of parallel queries. These results show that CQELS outperforms related approaches by orders of magnitude.
In this paper we address the problem of scalable, native and adaptive query processing over Linked Stream Data integrated with Linked Data. Linked Stream Data consists of data generated by stream sources, e.g., sensors, enriched with semantic descriptions, following the standards proposed for Linked Data. This enables the integration of stream data with Linked Data collections and facilitates a wide range of novel applications. Currently available systems use a “black box” approach which delegates the processing to other engines such as stream/event processing engines and SPARQL query processors by translating to their provided languages. As the experimental results described in this paper show, the need for query translation and data transformation, as well as the lack of full control over the query execution, pose major drawbacks in terms of efficiency. To remedy these drawbacks, we present CQELS (Continuous Query Evaluation over Linked Streams), a native and adaptive query processor for unified query processing over Linked Stream Data and Linked Data. In contrast to the existing systems, CQELS uses a “white box” approach and implements the required query operators natively to avoid the overhead and limitations of closed system regimes. CQELS provides a flexible query execution framework with the query processor dynamically adapting to the changes in the input data. During query execution, it continuously reorders operators according to some heuristics to achieve improved query execution in terms of delay and complexity. Moreover, external disk access on large Linked Data collections is reduced with the use of data encoding and caching of intermediate query results. To demonstrate the efficiency of our approach, we present extensive experimental performance evaluations in terms of query execution time, under varied query types, dataset sizes, and number of parallel queries. These results show that CQELS outperforms related approaches by orders of magnitude.