Outline
Security researchers have found significant vulnerabilities in learning algorithms used for text analysis: With the help of a novel attack, they were able to show that topic recognition algorithms can be fooled by even small changes in words, sentences and references. While human readers hardly notice a difference due to the attack, learning algorithms like topic models are misled and identify wrong topics. Using the example of peer review of scientific texts, the researchers show the danger posed by this attack and that better security precautions are necessary.
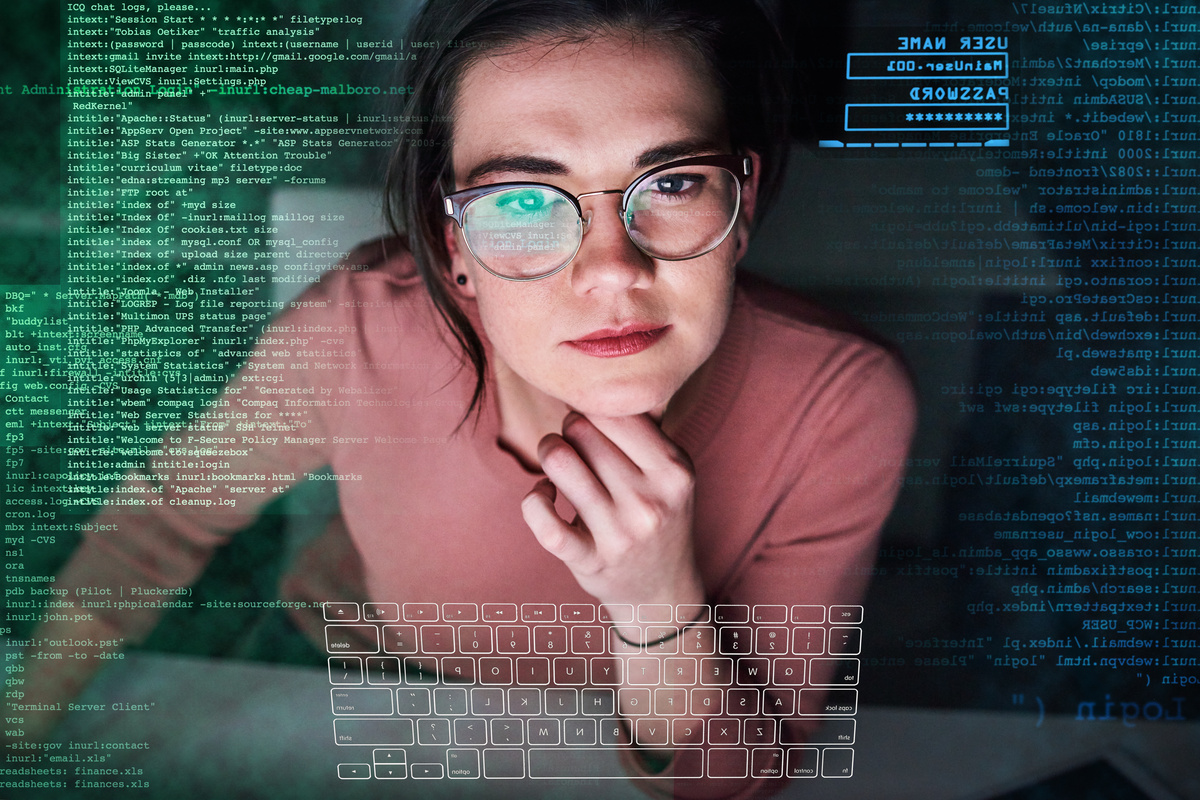
Details
Machine Learning and Security
Machine learning algorithms have become an important tool in the analysis of texts, not just since ChatGPT. In particular, the automatic recognition of topics with so-called topic models is used in many places to quickly sort texts. For example, these models are used by the organizers of scientific conferences to automatically distribute submitted articles to reviewers.
To investigate the security of this AI-powered text analysis, a research team has unveiled a new attack against topic models. This attack manipulates texts by making small changes to words, phrases, and references. The researchers call these manipulated documents Adversarial Papers. They ensure that targeted false topics are detected by the learning algorithms of the topic models. The automatic assignment of reviewers at scientific conferences can thus be influenced. It becomes possible to exclude disagreeable reviewers and include well-meaning individuals. "It is not exactly in the spirit of science if manipulated conference papers select their own reviewers," says Prof. Dr. Konrad Rieck, who heads the research team at the Berlin Institute for the Foundations of Learning and Data (BIFOLD).
While human readers hardly notice a difference due to the attack, the targeted omission and addition of terms quickly leads the learning algorithms astray. In order to make as few of these changes as possible, the research team has developed a new method that gradually performs small manipulations, measures their effect, and thus successively adjusts a text. Here, the method goes back and forth again and again to achieve the most effective but unobtrusive manipulation possible. In the end, not only can the topics be changed, but in the case of scientific papers, the reviewers can be selected. For this purpose, the researchers simulated the submission process of two major conferences and successfully manipulated the assignment of reviewers with their attack.
The research team proposes various measures to prevent manipulation in texts. It has also informed the operators of systems for reviewing conference papers about the attack and possible countermeasures. However, the fundamental problem of automatically falsified texts cannot currently be solved and further research is needed. "Just as learning algorithms perceive images differently from humans, they also perceive texts differently from us. This can quickly lead to confusion, which can be exploited by attackers. We still have a lot of work ahead of us," explains Rieck.
*The paper was presented on August 11, 2023, at the 32nd USENIX Security Symposium by Thorsten Eisenhofer.
Topic related tweet by Prof. Dr. Konrad Rieck, August 11, 2023: https://twitter.com/mlsec/status/1690042108239917067 (13.187 Views, 2023/08/11, 9:00 am)
Update September 13, 2023: Press information published on IDW https://idw-online.de/en/news820371