Distribution-aware Data Integration
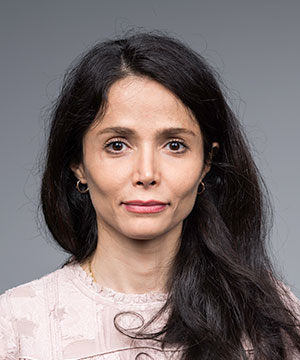
Abstract:
The rise of powerful and data-hungry models has shifted the focus from model-centric AI to data-centric AI, where the primary effort lies in collecting, cleaning, and improving the quality of data. Data integration is a core task in the data pre-processing step of ML pipelines. Despite decades of research in this field, the synergies of data bias and distribution with different dimensions of model quality are still understudied. This talk will explore the impact of data distribution on two integration tasks: data acquisition and entity matching. Data acquisition is the task of combining various data sources to construct a dataset with specific schema and distribution requirements. Entity matching seeks to match pairs of entity records from (the same or different) data sources that refer to the same real-world entity. In this talk, Fatemeh Nargesian will first describe how ideas from randomized algorithms can be used to tailor a dataset with desired distribution requirements from multiple sources to construct unbiased datasets. Next, she will present observations from an extensive experimental evaluation of the fairness of various entity-matching techniques. Finally, she will examine some preliminary results on the fair data selection problem.
Curriculum vitae:
Fatemeh Nargesian’s research in data management focuses on the discovery and integration of data in very large repositories of heterogeneous and raw data. Her research applies probabilistic and learning techniques to data management problems. Her previous work studies automated machine learning including feature engineering and model selection. Fatemeh received her PhD from the University of Toronto.