The Berlin Institute for the Foundations of Learning and Data (BIFOLD) set up two new Research Training Groups, led by Dr. Stefan Chmiela and Dr. Steffen Zeuch. The goal of these new research units at BIFOLD is to enable a junior researcher to conduct independent research and prepare him for a leadership position. Initial funding includes their own position as well as two PhD students and/or research associates for three years.
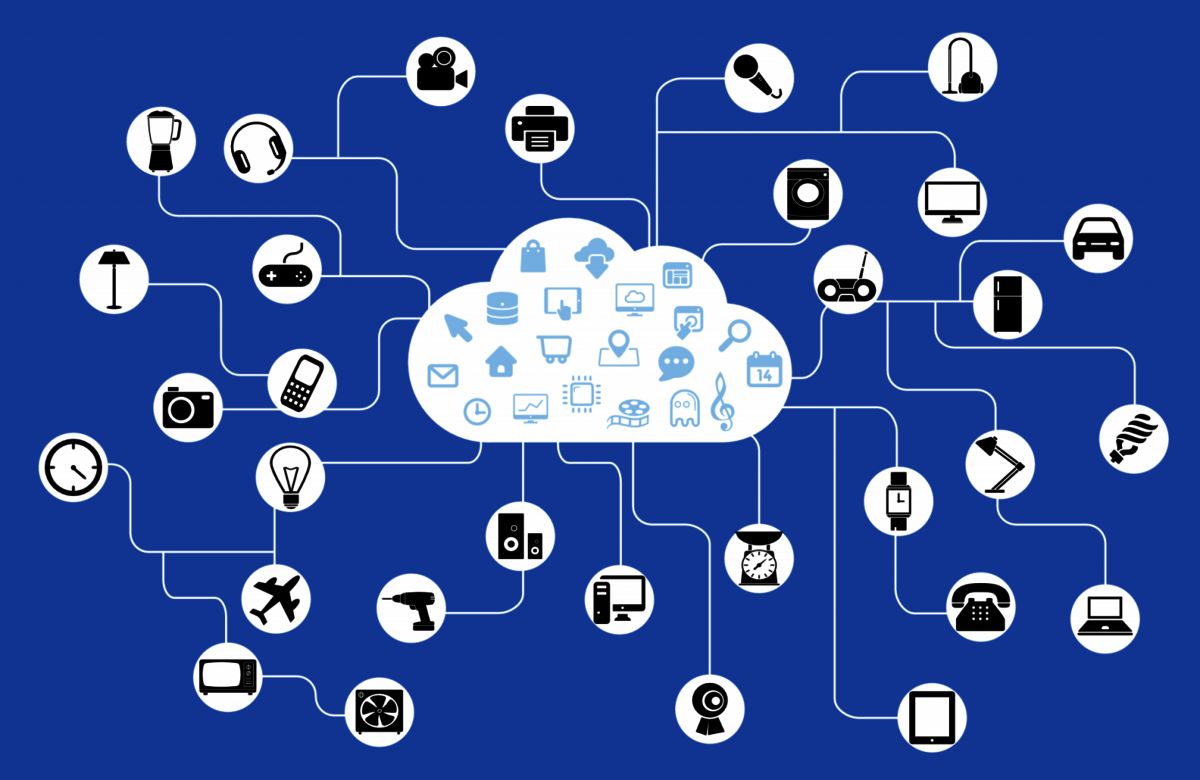
Steffen Zeuch is interested in how to overcome the data management challenges that the growing number of Internet of Things (IoT) devices bring: “Over the last decade, the amount of produced data has reached unseen magnitudes. Recently, the International Data Corporation estimated that by 2025, the global amount of data will reach 175 Zettabyte (ZB) and that 30 percent of this data will be gathered in real-time. In particular, the number of IoT devices increases exponentially such that the IoT is expected to grow as large as 20 billion connected devices in 2025.” The explosion in the number of devices will create novel data-driven applications in the near future. These applications require low-latency, location awareness, wide-spread geographical distribution, and real-time data processing on potentially millions of distributed data sources.
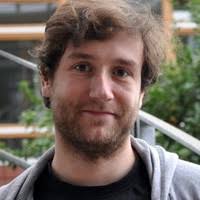
“To enable these applications, a data management system needs to leverage the capabilities of IoT devices outside the cloud. However, today’s classical data management systems are not ready yet for these applications as they are designed for the cloud,” explains Steffen Zeuch. “The focus of my research lies in introducing the NebulaStream Platform – a general purpose, end-to-end data management system for the IoT.”
Stefan Chmiela concentrates on so-called many-body problems. This broad category of physical problems deals with systems of interacting particles, with the goal to accurately characterize their dynamic behavior. These types of problems arise in many disciplines, including quantum mechanics, structural analysis and fluid dynamics and generally require solving high-dimensional partial differential equations. “In my research group we will particularly focus on problems from quantum chemistry and condensed matter physics, as these fields of science rank among the most computationally intensive”, explains Stefan Chmiela. In these systems, highly complex collective behavior emerges from relatively simple physical laws for the motion of each individual particle. Because of this, the simulation of high-dimensional many-body problems requires extremely challenging computation capacities. There is a limit to how much computational efficiency can be gained through rigorous mathematical and physical approximations, yet fully empirical solutions are often too simplistic to be sufficiently predictive.
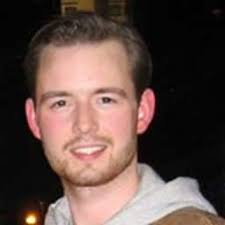
The lack of simultaneously accurate and efficient approaches makes many phenomena hard to model reliably. “Reconciling these two contradicting aspects of accuracy and computational speed is our goal” states Stefan Chmiela. “Our idea is to incorporate readily available fundamental prior knowledge into modern learning machines. We leverage conservation laws – which are derivable for many symmetries of physical systems, in order to increase the models ability to be accurate with less data.”