NebulaStream, the novel, general-purpose, end-to-end data management system for the IoT and the Cloud, recently announced the release of NebulaStream 0.2.0., the closed-beta release. The System is developed and explored by a team of BIFOLD researchers led by Prof. Dr. Volker Markl. It addresses the unique challenges of the “Internet of Things” (IoT). “Currently, NebulaStream is undergoing a heavy development process and we would love to cooperate with external users and developers, who may sign up for code access and collaborate with us and/or use NebulaStream for their own research”, explains Dr. Steffen Zeuch, group lead of the NebulaStream team.
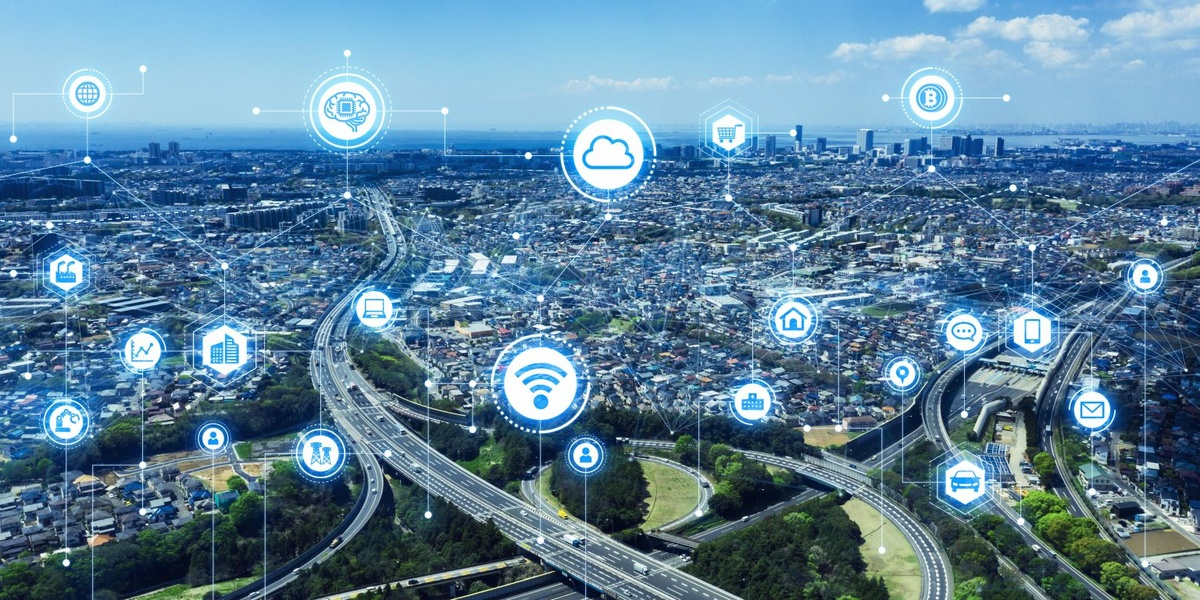
Recently, the International Data Corporation estimated that by 2025 the global amount of data will reach 175 zettabyte (ZB) and that 30 percent of this data will be gathered in real-time. In particular, more and more data will be generated by an exponentially increasing number of IoT devices (up to 20 billion connected devices in 2025), that continuously improve their processing capabilities. At the same time, the data processing landscape rapidly evolved towards a cloud-centric environment. Service providers leverage virtually unlimited resources to build novel data processing systems which offer high scalability, elasticity, and fault tolerance. As a result, end users can choose from a wide range of services depending on their requirements. However, the strong focus on cloud-based services fundamentally neglects that the majority of interesting data is produced outside the cloud. Thus, the main question for future system designs is how to enable analytics on zettabytes of data produced outside the cloud from millions of geo-distributed, heterogeneous devices in realtime.
To this end, NebulaStream aims to unify data management approaches that until now are realized in different systems: cloud-based streaming systems, fog-based data processing systems, and sensor networks. Cloud-based streaming systems support virtually unlimited processing capabilities and resource elasticity in the cloud but require that all data is shipped from sensors to the data center. Fog or edge-based data processing systems move the data processing to the sensor to reduce data transmission costs and can handle unreliable network connections but do not make use of cloud-based resources. Sensor networks optimize for battery lifetime and energy efficiency but do not support the execution of general queries. “As soon as the majority of interesting data will be produced outside the cloud, in the Smart-X universe, e.g., smart city, smart grid, smart home, we need to envision a unified data management system like NebulaStream that holistically manages the cloud, the edge, and the sensors”, says Steffen Zeuch.
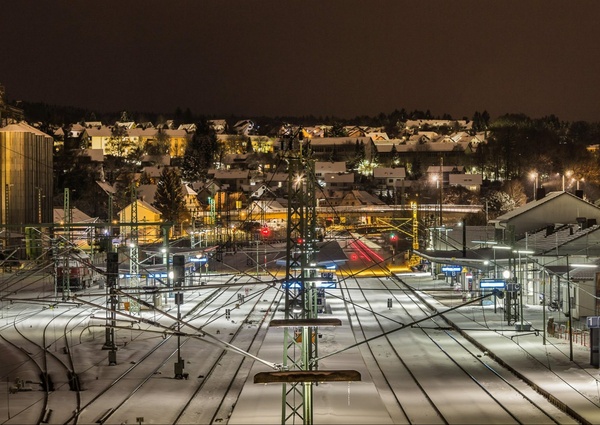
“A good everyday example is a public transportation provider in the city: Buses, trains, taxis, and so on are equipped with sensors that measure location, speed, occupancy, and many more metrics. All of this data is picked up by stationary base stations in the city and by using NebulaStream, either processed locally or forwarded through a network to the cloud. The data can be analyzed in real time and lead to actions that change the physical nature of the network. For example, if there is more demand on a station (higher occupancy) or traffic (longer wait times), then the transport provider can dispatch more vehicles”, explains Viktor Rosenfeld, member of the NebulaStream team. NebulaStream addresses these challenges by enabling heterogeneity and distribution of compute and data, supports diverse data and programming models going beyond relational algebra, deals with potentially unreliable communication, and enables constant evolution under continuous operation.
“NebulaStream is part of two joint international projects with a number of industrial and academic members”, adds Steffen Zeuch. ExDRa is a collaboration between Siemens, TU Graz, DFKI, and TU Berlin. The use case is the production process in paper mills. The project aims to predict the final paper quality during the production process. Various data are collected during the production from multiple production sites and fed into a federated learning process. NebulaStream is used to acquire sensor data and feed it into SystemDS which implements the federated learning pipeline.
ELEGANT is a Horizon 2020 project by the EU commission. It consists of six industry partners which provide use cases and expertise, and four academic partners, including TU Berlin. The goals of ELEGANT are similar to the goals of NebulaStream, i.e., to unify IoT processing and cloud processing, with a special focus on supporting hardware acceleration. The industry partners provide different use cases like video surveillance to extract a summary of interesting events from audio/video streams, a smart metering use case to monitor water usage and profile device usage, or a smart riding use case to classify skill of motorbike riders.
The publication in detail:
Sebastian Baunsgaard, Matthias Boehm, Ankit Chaudhary, Behrouz Derakhshan, Stefan Geißelsöder, Philipp M. Grulich, Michael Hildebrand, Kevin Innerebner, Volker Markl, Claus Neubauer, Sarah Osterburg, Olga Ovcharenko, Sergey Redyuk, Tobias Rieger, Alireza Rezaei Mahdiraji, Sebastian Benjamin Wrede, Steffen Zeuch: ExDRa: Exploratory Data Science on Federated Raw Data, SIGMOD/PODS ’21: June 2021, p. 2450–2463
>
Data science workflows are largely exploratory, dealing with under-specified objectives, open-ended problems, and unknown business value. Therefore, little investment is made in systematic acquisition, integration, and pre-processing of data. This lack of infrastructure results in redundant manual effort and computation. Furthermore, central data consolidation is not always technically or economically desirable or even feasible (e.g., due to privacy, and/or data ownership). The ExDRa system aims to provide system infrastructure for this exploratory data science process on federated and heterogeneous, raw data sources. Technical focus areas include (1) ad-hoc and federated data integration on raw data, (2) data organization and reuse of intermediates, and (3) optimization of the data science lifecycle, under awareness of partially accessible data. In this paper, we describe use cases, the overall system architecture, selected features of SystemDS’ new federated backend (for federated linear algebra programs, federated parameter servers, and federated data preparation), as well as promising initial results. Beyond existing work on federated learning, ExDRa focuses on enterprise federated ML and related data pre-processing challenges. In this context, federated ML has the potential to create a more fine-grained spectrum of data ownership and thus, even new markets.
Data science workflows are largely exploratory, dealing with under-specified objectives, open-ended problems, and unknown business value. Therefore, little investment is made in systematic acquisition, integration, and pre-processing of data. This lack of infrastructure results in redundant manual effort and computation. Furthermore, central data consolidation is not always technically or economically desirable or even feasible (e.g., due to privacy, and/or data ownership). The ExDRa system aims to provide system infrastructure for this exploratory data science process on federated and heterogeneous, raw data sources. Technical focus areas include (1) ad-hoc and federated data integration on raw data, (2) data organization and reuse of intermediates, and (3) optimization of the data science lifecycle, under awareness of partially accessible data. In this paper, we describe use cases, the overall system architecture, selected features of SystemDS’ new federated backend (for federated linear algebra programs, federated parameter servers, and federated data preparation), as well as promising initial results. Beyond existing work on federated learning, ExDRa focuses on enterprise federated ML and related data pre-processing challenges. In this context, federated ML has the potential to create a more fine-grained spectrum of data ownership and thus, even new markets.