The paper “Beyond Smoothness: Incorporating Low-Rank Analysis into Nonparametric Density Estimation” by BIFOLD researcher Dr. Robert A. Vandermeulen and his colleague Dr. Antoine Ledent, Technical University Kaiserslautern, was presented at the Conference on Neural Information Processing Systems (NeurIPS 2021). Their paper provides the first solid theoretical foundations for applying low-rank methods to nonparametric density estimation.
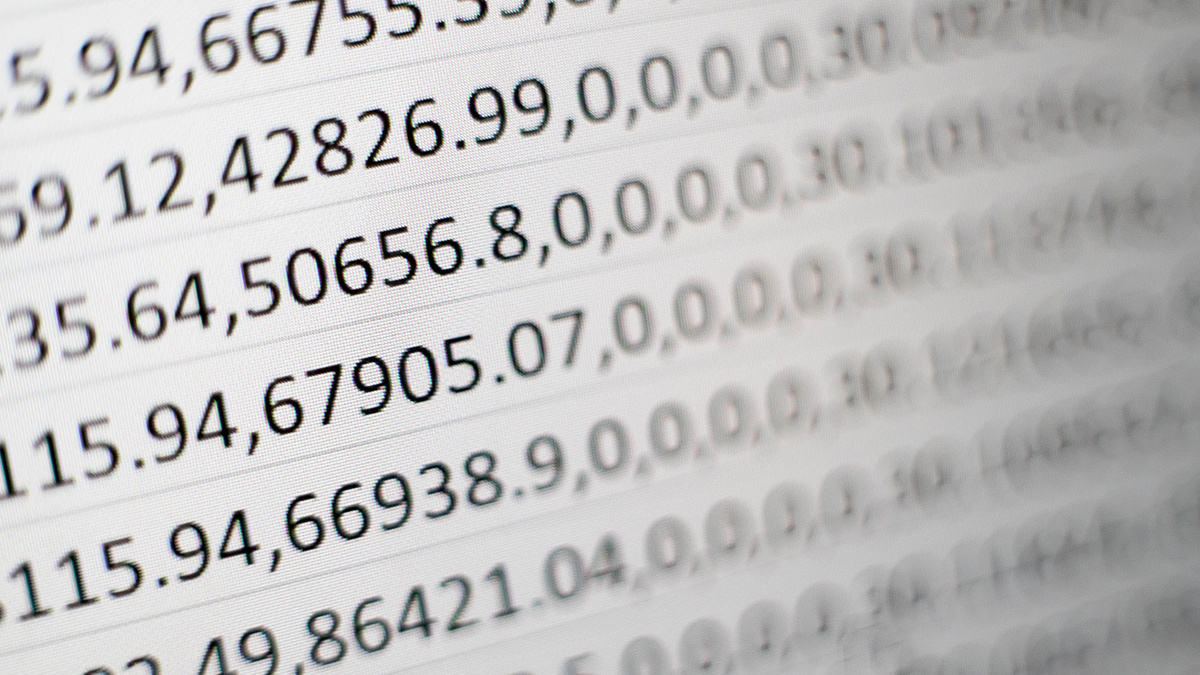
It is well-known that problems in statistics and machine learning become more difficult as the dimensionality, or number of features in the data grows larger. For example, when using collected data to make a predictor for the likelihood of a given type of cancer, patient information about their age, gender, weight and alcohol consumption would be fairly useful (four dimensions). If in addition 50.000 pieces of genetic data would be included, the information this data contains is potentially far more effective for predicting cancer than the four health markers. However, an enormous amount of patient data would be required to discern which variations of these 50.000 markers are relevant for predicting cancer. This kind of problem occurs in virtually all machine learning and statistics with a large number of dimensions and is termed ”the curse of dimensionality.” One reason for the current interest in neural networks is their ability to circumvent this problem. However researchers still have essentially no mathematical understanding as to why this is the case.
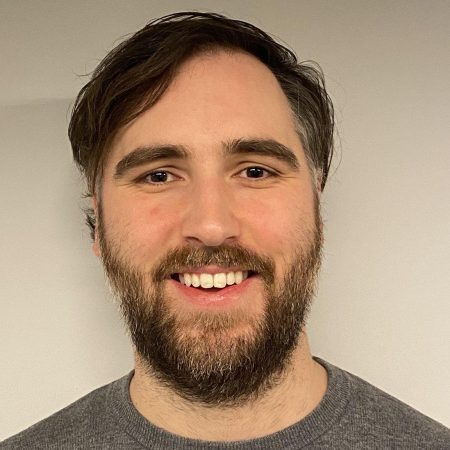
“Lifting the curse of dimensionality for nonparametric density estimations is a breakthrough. Being able to combine a high number of features with flexible statistical methods opens up many possibilities for new machine learning applications.”
Outside of neural networks in deep learning, there are methods for obviating the curse of dimensionality that are well-understood mathematically. A very popular method for this is known as ”compressed sensing.” This method was invented partially by Terrence Tao, a Fields Medalist and potentially the most famous living mathematician. The method has been applied with great success to linear methods however its underlying principles have yet to be extended to the highly flexible ”nonparametric” statistical methods, which are capable of utilizing or estimating complex dependencies between features. In this paper the researchers were able to prove that applying low-rank methods to nonparametric density estimation can completely eliminate the curse of dimensionality. This is a result that may be valid for other areas of nonparametric statistics as well.
The Publication in detail:
Robert A. Vandermeulen, Antoine Ledent: Beyond Smoothness: Incorporating Low-Rank Analysis into Nonparametric Density Estimation. NeurIPS 2021
>
The construction and theoretical analysis of the most popular universally consistent nonparametric density estimators hinge on one functional property: smoothness. In this paper we investigate the theoretical implications of incorporating a multi-view latent variable model, a type of low-rank model, into nonparametric density estimation. To do this we perform extensive analysis on histogram style estimators that integrate a multi-view model. Our analysis culminates in showing that there exists a universally consistent histogram style estimator that converges to any multi-view model with a finite number of Lipschitz continuous components at a rate of ˜O(1/3√n) in L1 error, compared to the standard histogram estimator which can converge at a rate slower than 1/d√n on the same class of densities. Beyond this we also introduce a new type of nonparametric latent variable model based on the Tucker decomposition. A very rudimentary experimental implementation of the ideas in our paper demonstrates considerable practical improvements over the standard histogram estimator. We also provide a thorough analysis of the sample complexity of our Tucker decomposition based model. Thus, our paper provides solid first theoretical foundations for extending low-rank techniques to the nonparametric setting.
The construction and theoretical analysis of the most popular universally consistent nonparametric density estimators hinge on one functional property: smoothness. In this paper we investigate the theoretical implications of incorporating a multi-view latent variable model, a type of low-rank model, into nonparametric density estimation. To do this we perform extensive analysis on histogram style estimators that integrate a multi-view model. Our analysis culminates in showing that there exists a universally consistent histogram style estimator that converges to any multi-view model with a finite number of Lipschitz continuous components at a rate of ˜O(1/3√n) in L1 error, compared to the standard histogram estimator which can converge at a rate slower than 1/d√n on the same class of densities. Beyond this we also introduce a new type of nonparametric latent variable model based on the Tucker decomposition. A very rudimentary experimental implementation of the ideas in our paper demonstrates considerable practical improvements over the standard histogram estimator. We also provide a thorough analysis of the sample complexity of our Tucker decomposition based model. Thus, our paper provides solid first theoretical foundations for extending low-rank techniques to the nonparametric setting.