Is it possible to learn about the health status of a population and potential side effect of medications by analyzing social media conversations? BIFOLD researchers tackled the challenge of making social media posts of medical laypersons concerning diseases and medications understandable for machines. At the BioCreative VII Challenge Evaluation Workshop 2021, they recently explored how a combination of background knowledge and a language transformer model can increase the precision of medical information extraction from Twitter texts.
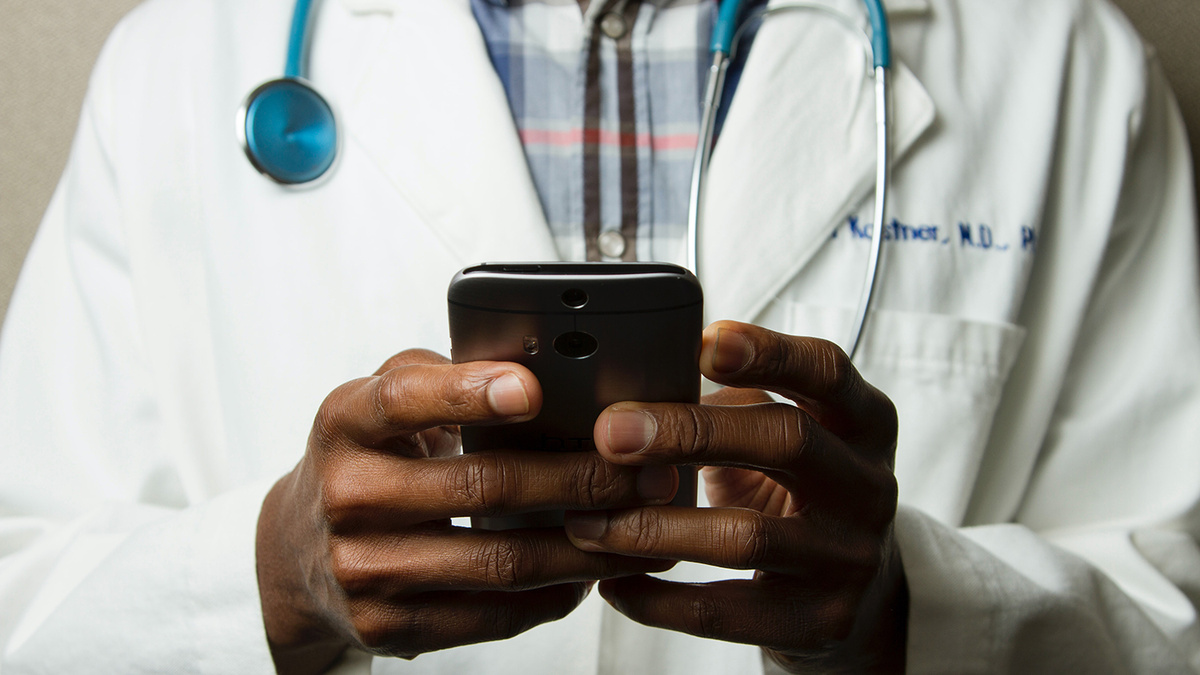
People share information about many aspects of their lives online – family, lifestyle, work, but also information about their health, medical drug intake and corresponding adverse drug reactions. Automatic extraction of health-related information on social media could potentially lead to valuable insights about population health and help to identify risk factors and unwanted side effects of commonly used medications.
The challenge for the automatic processing of social media texts lies in the casual language used. While machine learning algorithms can be very powerful, their accuracy relies on high quality data to be trained on. In a medical context these often are large annotated data sets including well-written sentences in medical expert’s expressions – often in latin. “The medical layman’s terms used on social media have to be either linked to or transformed into the corresponding precise medical expressions before we can gain insights from it with machine learning methods”, explains BIFOLD researcher Dr. Philippe Thomas.
Researchers in the group of BIFOLD Fellow Prof. Dr. Sebastian Möller, head of the Speech and Language Technology Research Department at the German Research Center for Artificial Intelligence (DFKI), do both. Dr. Philippe Thomas, Dr. Roland Roller and Lisa Raithel focus on the development of multilingual information extraction techniques for the detection of adverse drug reactions in social media and disease-specific forums. They create new annotated datasets as well as transformer models that translate natural language expressions into the corresponding medical terms.
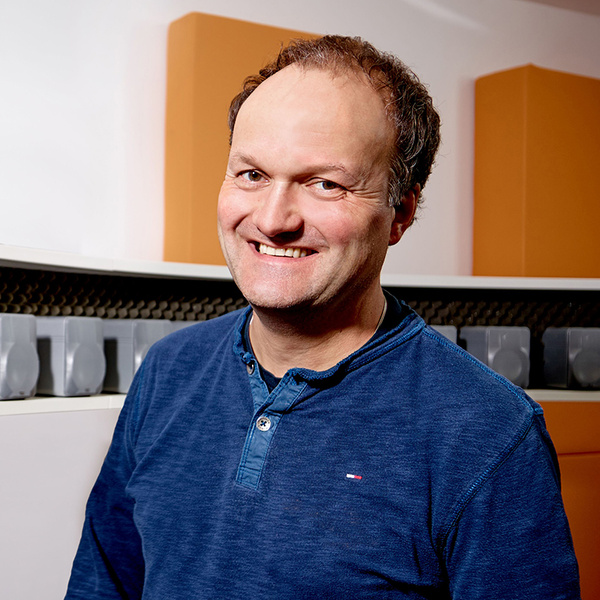
“Our multilingual learning approach enables Natural Lange Proccessing applications where large corpora or manually crafted language ressources are missing. Medical information extraction is just one of many applications made possible by our research at BIFOLD.”
More recently, BIFOLD researchers Roland Roller, Ammer Ayach, and Lisa Raithel tackled the “Automatic extraction of medication names in tweets” track challenge of the 2021 BioCreative VII Challenge Evaluation Workshop in their paper “Boosting Transformers using Background Knowledge, or how to detect Drug Mentions in Social Media using Limited Data”. The goal was to extract drug and medication mentions in Twitter posts by pregnant women. The provided data sets were especially challenging as the short nature of Tweets leads to very low context information and the data included very few actual mentions of medications. To handle these data limitations, the researchers boosted the performance of a pre-trained language transformer model by introducing background knowledge. They re-mapped medical annotations from the given data to unlabeled texts with string-matching. While string matching is very precise, it is limited to already existing labels. The transformer model on the other hand can detect new medical mentions by taking context information into account. The combination of both approaches significantly enhanced the information extraction performance. “You could compare this to a student who relies on a textbook for learning, but also on the advice of a tutor who has to explain complex application cases – both complement each other to gain a better understanding,” says Roland Roller.
The publication in detail:
Roland Roller, Ammer Ayach, Lisa Raithel: Boosting Transformers using Background Knowledge, or how to detect Drug Mentions in Social Media using Limited Data. BioCreative VII Challenge Evaluation Workshop 2021: 189
>
To process natural language and to extract information from text, transformers are currently the model of choice for many different tasks. Conversely, if the number of training examples is very limited, fine-tuning might not achieve the expected results, similarly as for other machine learning methods. In the past, a large range of different techniques have been presented to overcome this challenge, such as data augmentation or using distantly labelled data. In this work, we present our contribution to the drug mention detection of the BioCreative VII Challenge (Track 3), which includes a large number of negative, but only a small proportion of positive documents. In course of this, we explore different techniques to boost performance of a pre-trained transformer model. The combination of our transformer model and usage of background knowledge achieved the best results for our use case.
To process natural language and to extract information from text, transformers are currently the model of choice for many different tasks. Conversely, if the number of training examples is very limited, fine-tuning might not achieve the expected results, similarly as for other machine learning methods. In the past, a large range of different techniques have been presented to overcome this challenge, such as data augmentation or using distantly labelled data. In this work, we present our contribution to the drug mention detection of the BioCreative VII Challenge (Track 3), which includes a large number of negative, but only a small proportion of positive documents. In course of this, we explore different techniques to boost performance of a pre-trained transformer model. The combination of our transformer model and usage of background knowledge achieved the best results for our use case.
Contact:
Prof. Dr. Sebastian Möller
Deutsches Forschungszentrum für Künstliche Intelligenz (DFKI) GmbH
DFKI Project Office
Speech and Language Technology
Alt-Moabit 91c
D-10559 Berlin