The Berlin Institute for the Foundations of Learning and Data is very pleased to announce the first six BIFOLD Junior Fellows. They were selected for the excellence of their research and are already well-established researchers in the computer sciences. In addition, their research interests show exceptional potential for BIFOLD’s research goals, either by combining machine learning and data management or by bridging the two disciplines and other research areas. The first six Junior Fellows will cover a broad range of research topics during their collaboration with BIFOLD.
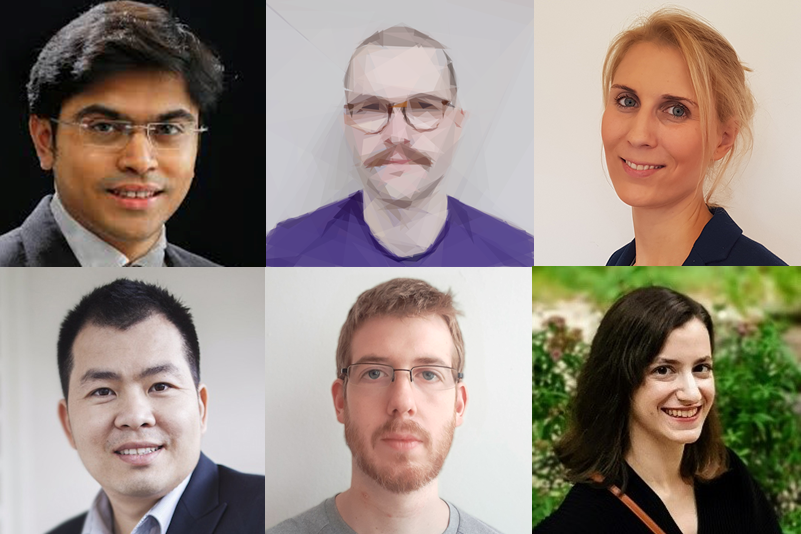
These excellent researchers will receive mentoring from a BIFOLD Research Group Leader or Fellow, as well as opportunities to mentor a graduate school student and additional funding to support their research activities.Their research in collaboration with BIFOLD will advance the sciences in the following areas:
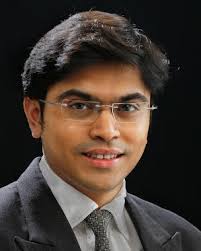
Dr. Kaustubh Beedkar:
My research interest lies in exploring efficient and effective tools and methods for compliant geo-distributed data analytics. In contrast to traditional means, my work focuses on building data processing frameworks that enable decentralized data analytics. In particular, I research how to integrate legal constraints arising from regulatory bodies concerning data sovereignty and data movement, as well as disparate compute infrastructures at multiple sites into data processing frameworks. For example, processing data generated by autonomous cars in three different geographies, such as Europe, North America, and Asia may face different regulations: there may be legal requirements that only aggregated, anonymized data may be shipped out of Europe and no data whatsoever may be shipped out of Asia. In my research, I explore how to specify and enforce compliance to these types of constraints while generating efficient query execution plans.
“The BIFOLD junior fellowship offers excellent opportunities in the form of seminars, training, and mentorship from world-renowned researchers, to hone my research skills in order to pursue a successful career in academia.”
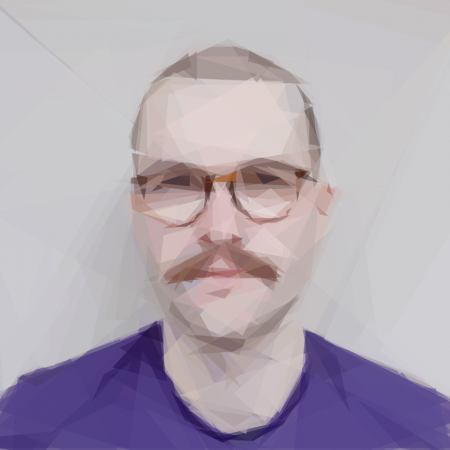
Dr. Jan Hermann:
Many modern technologies stand on our ability to design novel molecules and materials. This design can be greatly assisted by computer simulations of the chemical and physical properties of these novel compounds. Typical realistic simulations require a whole hierarchy of different computational methods. One central component of this hierarchy are methods that can directly model the behavior of electrons in molecules and materials. Currently, such methods are limited either by computational efficiency or by accuracy. Recently, machine learning techniques have been successfully used across physical sciences, but not to target the direct modeling of electrons. My research attempts to fill this gap through tight integration of machine learning into existing methods for simulation of electrons in molecules and materials. The goal is to lift the restrictions on the use of these methods by broadening the scope of materials for which they can be effectively used.
“BIFOLD offers the opportunity to cooperate with other experts at the intersection of computer sciences and the physical sciences. Through mentoring opportunities in the BIFOLD junior fellowship program, I also hope to inspire the next generation of scientists to engage in machine-learning-based research in the physical sciences.”
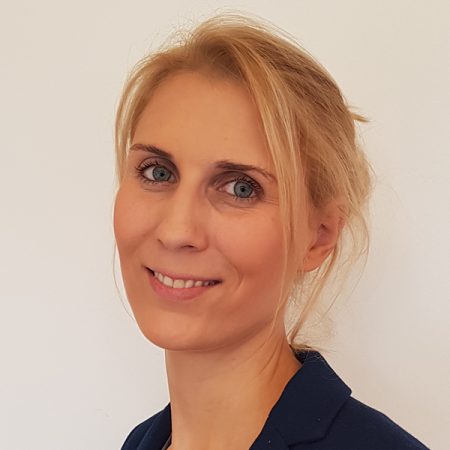
Dr. Marina Marie-Claire Höhne:
In order to bring the positive potential of artificial intelligence (AI) into real applications, for example in cancer diagnostics, where AI machines detect cancer cells within milliseconds, we need to understand the machine’s decision. My research together with my junior research group UMI lab (Understandable Machine Intelligence) aims to understand the highly complex learning machines and contributes to the field of explainable AI. In particular, I develop methods that enable a holistic understanding of AI models and, with the help of Bayesian networks, additionally transfer the prediction uncertainties from the AI model to the explanation.
“For me, BIFOLD is one of the crucial building blocks that contribute to research at the level of excellence. In my opinion, the accumulation of expert knowledge from different areas of AI is the decisive criterion for attaining a higher level of knowledge, which can lead to a holistic understanding of AI and is important in order to exploit the enormous potential of AI innovations.“
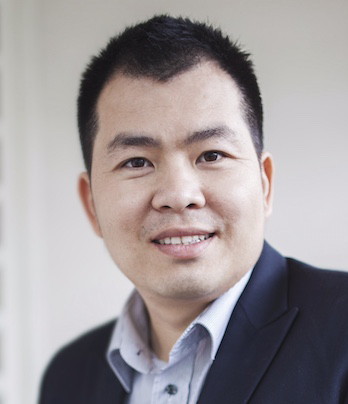
Dr. Danh Le Phuoc:
The core of my research interest is centered around the research problem of how to autonomously fuse different types of sensory streams to build high-level perception, planning and control components for robots, drones and autonomous vehicles. My approach for this problem is leveraging common-sense and domain knowledge to guide the learning and reasoning tasks of such data fusion operations. The common-sense and domain knowledge are used to emulate the reasoning ability of a human. To this end, my research mission in BIFOLD is to develop scalable reasoning and learning algorithms and systems which bring the best both worlds, neural networks and symbolic reasoning, called neural-symbolic fusion frameworks.
“The BIFOLD network will facilitate my collaboration with BIFOLD experts in machine learning, database and large-scale processing to push the frontiers of the neural-symbolic research which is among the emerging key foundations of data processing and learning.”
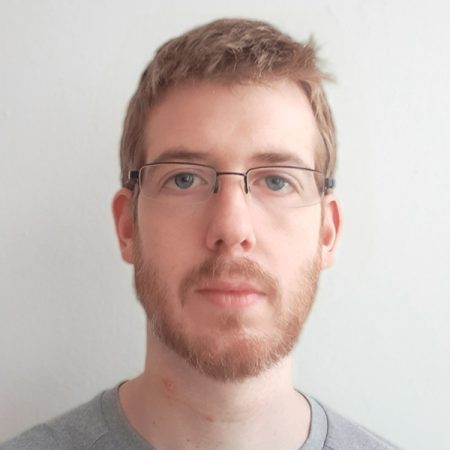
Dr. Kristof Schütt:
Finding molecules and materials with specific chemical properties is important for progress in many technological applications, such as drug design or the development of efficient batteries and solar cells. In my research, I aim to accelerate this process by using machine learning for the discovery, simulation and understanding of these chemical systems. To achieve this, I study neural network that predict the chemical properties of molecules, or even directly generate molecules that possess a desired property.
“BIFOLD brings researchers from many fields together. Working interdisciplinary at the intersection of machine learning and quantum chemistry, I enjoy the exchange with other disciplines as it always results in new ideas and perspectives. Machine learning methods can often be applied to similar problems in quite different applications. Therefore, you can both learn from and contribute to many fields as a machine learning researcher – for which BIFOLD provides the ideal environment.“
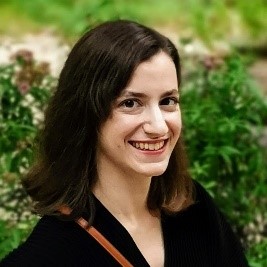
Dr. Eleni Tzirita Zacharatou:
Spatial data is at the heart of any human activity. Billions of mobile devices, cars, social networks, satellites, sensors, scientific simulations, and many other sources produce spatial data constantly. My research aims to provide efficient tools to store, process, and manage the wealth of spatial data available today, thereby enabling discoveries, better services, and new products. To that end, I apply a broad portfolio of techniques, from efficient use of modern hardware to approximation algorithms and workload-driven adaptation. My goal within BIFOLD is to create an efficient data ecosystem that brings the benefits of spatial big data analytics to a broad community, helps to boost data literacy, and contributes to citizen science.
“BIFOLD offers great collaboration opportunities in data management, machine learning, and earth observation that can help me advance my research. In addition, the BIFOLD junior fellowship program allows me to evolve my mentoring skills and provides further training opportunities, thereby contributing to the successful development of my academic career.”