Learned Cost Models for Stream Processing in Heterogeneous Environments
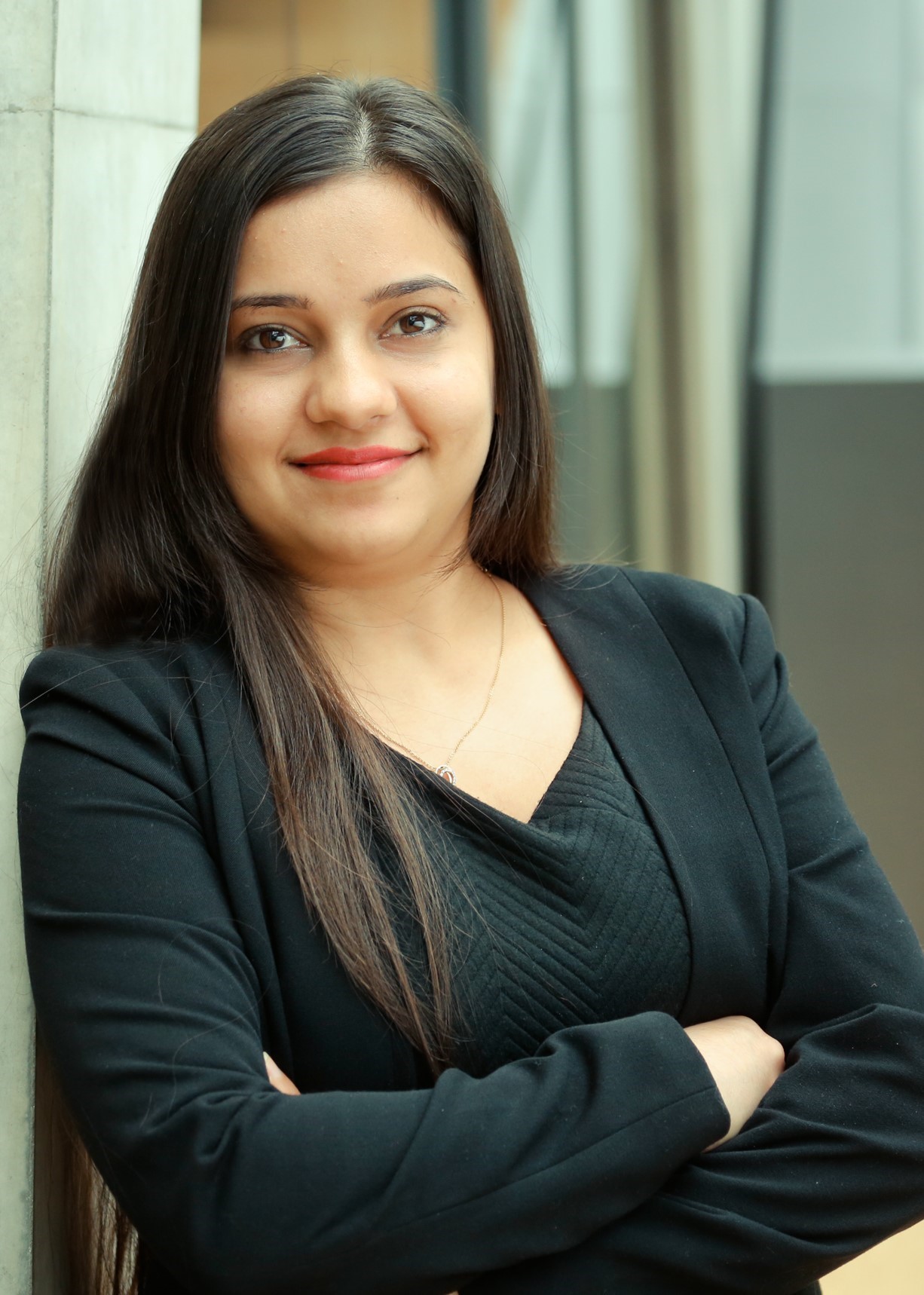
Abstract:
In this talk, Manisha Luthra Agnihotri will present a novel learned cost model designed to optimize the initial execution costs of stream processing queries on heterogeneous hardware, particularly crucial for IoT environments. This work paves the way for cost-based optimizations in streaming systems, such as determining operator placement and parallelism. Unlike existing approaches that lack generalisability, her work proposes a novel approach based on graph neural networks to predict the costs of streaming queries before execution that can generalise to unseen hardware placements and queries out-of-the-box. This enables accurate and effective placement and parallelism decisions, addressing the challenges posed by diverse hardware and network conditions of IoT environments. Her results demonstrate a speed-up of up to 21x compared to existing placement strategies using our learned cost model. Additionally, the model maintains accuracy in cost predictions even when extrapolating to unseen hardware and query configurations.
Short Bio:
Manisha Luthra Agnihotri is the Deputy Head at the German Research Center for Artificial Intelligence (DFKI) in Darmstadt and a Postdoctoral Researcher at TU Darmstadt. At DFKI, she co-leads the Systems AI for Decision Support group, focusing on topics ranging from learned system optimizations to multimodal data management. Her research interests lie at the intersection of machine learning, data systems and hardware, including work on learned cost-based optimizations and GPU- and RDMA-based acceleration of query workloads. Her doctoral thesis was honored with a prestigious German national award for the Best Ph.D. thesis by the GI/ITG special interest group on Communication and Distributed Systems (KuVS).
She has received several notable awards during her academic journey, including the Anita.Borg faculty scholarship, Zeiss scholarship for top dissertation and the DFG MAKI mentoring and networking awards. She has been invited to speak at numerous universities, including the University of Toronto, TU Delft, and the University of Groningen. Manisha has presented her work at major conferences and workshops on data management, including SIGMOD, VLDB, ICDE, and EDBT. She currently serves as a PC member for several international conferences like VLDB, EuroSys, DEBS, ICN and workshops at SIGMOD, ICDE, VLDB and NeurIPS. Additionally, she has peer-reviewed for international journals such as the VLDB Journal, TPDS, ACM Computing Surveys, and the IEEE Internet of Things Journal.