The BIFOLD Data Systems Lab - Systems, Research, and Applications
Abstract:
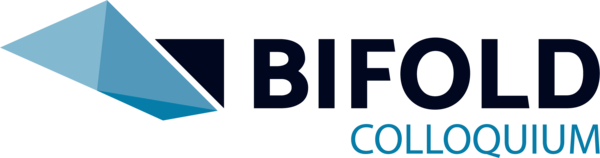
The BIFOLD Data Systems Lab unites all BIFOLD research groups related to data management and scalable systems, including the groups for database systems and information management (DIMA), big data engineering (DAMS), big data analytics for earth observation (RSiM), and distributed stream processing in heterogeneous environments. In this talk, we share an overview of joint activities and common research directions. In detail, we discuss federated data processing and learning, the acquisition and preprocessing of streaming and multi-modal data, as well as selected techniques for efficient and scalable processing. Finally, our primary application areas are earth observation and health-care/medical use cases. We describe technical challenges, domain-specific techniques, and our vision for an open citizen science platform.
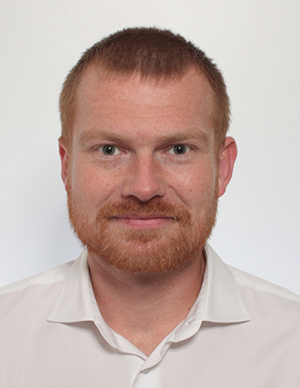
Speaker:
Matthias Boehm is a full professor for large-scale data engineering at Technische Universität Berlin and the BIFOLD center of excellence. His cross-organizational research group focuses on high-level, data science-centric abstractions as well as systems and tools to execute these tasks in an efficient and scalable manner. From 2018 through 2022, Matthias was a BMK-endowed professor for data management at Graz University of Technology, Austria, and a research area manager for data management at the co-located Know-Center GmbH. Prior to joining TU Graz in 2018, he was a research staff member at IBM Research - Almaden, CA, USA, with a major focus on compilation and runtime techniques for declarative, large-scale machine learning in Apache SystemML. Matthias received his Ph.D. from Dresden University of Technology, Germany in 2011 with a dissertation on cost-based optimization of integration flows. His previous research also includes systems support for time series forecasting as well as in-memory indexing and query processing.