SPEAKERS
This page provides basic information about the speakers of the BIFOLD Weizenbaum Summer School 2023.
Dr. Sebastian Buschjäger
Towards Energy-Efficient Model Application
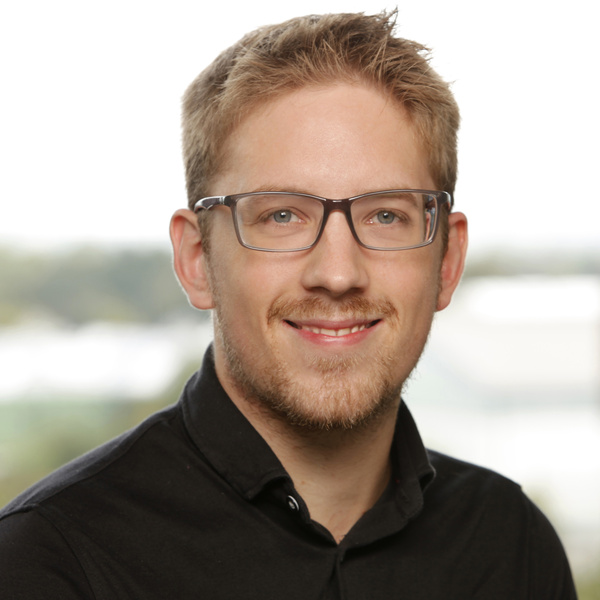
ABSTRACT: Data scientists often train multiple models over and over to find the best hyperparameter combination for the best possible performance. However, once this initial exploration phase is complete, the continuous application of the trained model across different users and/or devices often requires much more energy than its initial training. In this talk, we will discuss how to make already ML models more resource-efficient once they have been trained.
BIOGRAPHY: Sebastian Buschjäger is a postdoctoral researcher and the Coordinator of Resource-Aware Machine Learning at the Lamarr Institute for Machine Learning and Artificial Intelligence in Dortmund since 2023. Before that, he was a PhD student at the Artificial Intelligence Unit of the TU Dortmund University in Germany where he finished his dissertation (Dr. rer. nat.) in 2022 with distinction. His research focuses on machine learning methods combined with small devices and specialized hardware.
WEBSITE: www.buschjaeger.it
Prof. Andrea Cominola
Are roofs in Berlin becoming greener? Geodata and deep learning for sustainable urban development
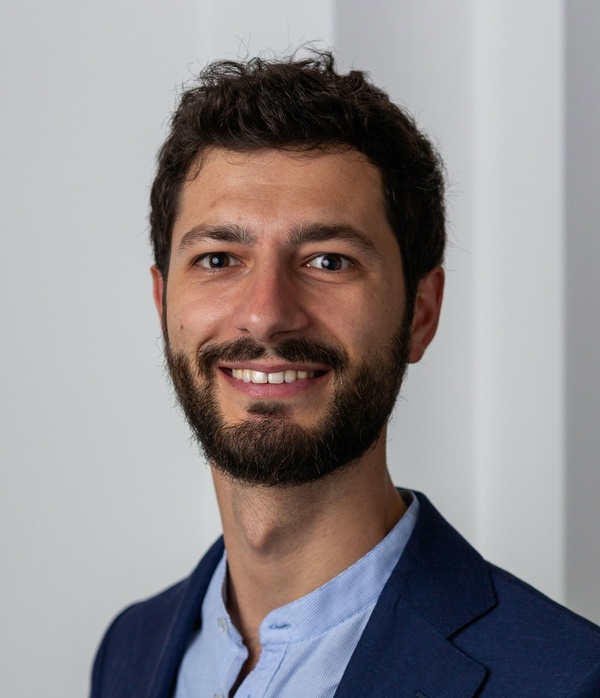
ABSTRACT: The dynamic interplay between population growth and climate change poses significant challenges to our cities. The pursuit of sustainable urban development becomes paramount to reduce the vulnerability of European critical infrastructure, mitigate the impact of hydroclimatic extremes, and ultimately improve the resilience of European populations to water-related hazards and support the achievement of climate neutrality in Europe by 2050. Blue-Green Infrastructure (BGI) such as green roofs are fundamental to achieve resilience against climate-induced hazards and conquer the above-mentioned challenges. Their large-scale adoption is critical in enhancing resilience against urban hazards, such as urban flooding, urban heat island effects, and biodiversity loss. Currently, the most popular policy format to encourage their roll-out is subsidy programs. In this talk, we will first introduce the concept of BGI and provide an overview of artificial intelligence methods to support BGI planning and management. We will than take the case study of Berlin to showcase how geodata and deep learning can be harnessed for automated monitoring of green roof development and assessment of BGI development plans across temporal scales. We validate the state-of-the-art tool open-source deep learning algorithm for green roof mapping and urban sustainability “Roofpedia” using satellite imagery for Berlin and showcase how it can be used to assess the efficacy of recent Berlin’s green roofing subsidy programs, which received 2.7 Million Euros since 2019. Broad focus will be devoted to unpacking current challenges in training and validating such approaches for automated BGI evolution monitoring and to foster transferability to different cities moving towards a transferrable policy evaluation framework.
BIOGRAPHY: Andrea Cominola is an Assistant Professor and head of the Smart Water Networks group at the Technische Universität Berlin and Einstein Center Digital Future. In early 2017 he was awarded a PhD in Information Technology at Politecnico di Milano for his thesis on modelling residential water consumption behavior with smart meter data. In 2013 he obtained his MSc in Environmental and Land Planning Engineering at Politecnico di Milano and Politecnico di Torino (double-degree). In early 2016, he was a visiting PhD candidate at the Watershed Sciences Center and Center for Water-Energy Efficiency of UC Davis (CA - USA). He was formerly a visiting researcher at Korea University (Seoul, Korea), and visiting student at PennState University (PA - USA) and Norwegian University of Science and Technology (Trondheim, Norway). Prof. Dr. Cominola’s research focuses on urban water systems modelling and management, leakage and anomaly detection, behavioral modelling, data mining, and machine learning for water and coupled human-environment systems analysis. Since May 2021 he is the Chair of the HS5 subdivision on Water policy, management and control at the European Geosciences Union (EGU). Since 2020 he is also a Principal Investigator in ide3a, the DAAD-funded international alliance for digital e-learning, e-mobility and e-research in academia (https://ide3a.net). Since 2019 he serves as Associate Editor for the Journal of Water Resources Planning and Management (American Society of Civil Engineers). He is author of 20 scientific publications in peer-reviewed international journals, 2 book chapters, more than 70 publications/presentations in international conferences, and reviewer for several international journals, organizations, and institutions. He also co-authored a technical report on “Climate change, infrastructure and mobility. Solutions and strategies for infrastructure invest- ments in a context adaptation to climate change and mitigation of greenhouse gas emissions”, prepared for the Italian Ministry of Sustainable Infrastructures and Mobility.
WEBSITE: https://www.tu.berlin/swn
PROF. Begüm Demir
Information Discovery from Big Earth Observation Data Archives
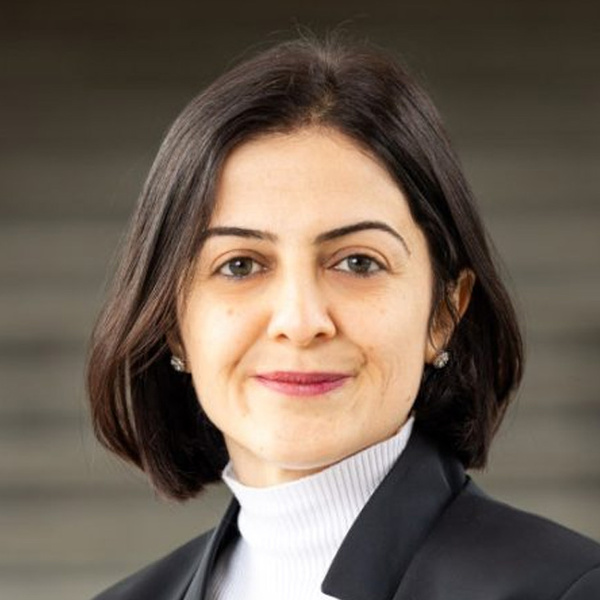
ABSTRACT: Earth observation (EO) data archives are explosively growing as a result of advances in satellite systems. As an example, remote sensing (RS) images acquired by ESA’s Sentinel satellites (which are a part of EU’s Copernicus program) reach the scale of more than 10 TB per day. The “big EO data” is a great source for information discovery and extraction for monitoring Earth from space. Thus, accurate and scalable techniques for RS image understanding, search and retrieval have recently emerged.
This lecture will start by introducing the characteristics of the images acquired by sensors mounted on satellite platforms. Then, a general overview on scientific and practical problems related to RS image characterization, indexing and search from massive archives will be initially discussed, and the state-of-the-art methods and approaches that can overcome these problems will be presented. Particular attention will be given to deep hashing networks that learn a semantic-based metric space, while simultaneously producing binary hash codes for scalable and accurate content-based indexing and retrieval of satellite images. Practical applications will be provided throughout the lecture.
BIOGRAPHY: Begüm Demir is currently a Full Professor and the founder head of the Remote Sensing Image Analysis (RSiM) group at the Faculty of Electrical Engineering and Computer Science, TU Berlin and the head of the Big Data Analytics for Earth Observation research group at the Berlin Institute for the Foundations of Learning and Data (BIFOLD). Her research activities lie at the intersection of machine learning, remote sensing and signal processing. Specifically, she performs research in the field of processing and analysis of large-scale Earth observation data acquired by airborne and satellite-borne systems. She was awarded by the prestigious '2018 Early Career Award' by the IEEE Geoscience and Remote Sensing Society for her research contributions in machine learning for information retrieval in remote sensing. In 2018, she received a Starting Grant from the European Research Council (ERC) for her project "BigEarth: Accurate and Scalable Processing of Big Data in Earth Observation". She is an IEEE Senior Member and Fellow of European Lab for Learning and Intelligent Systems (ELLIS).
Niklas Gebauer
Generative models for molecules: Challenges and promises
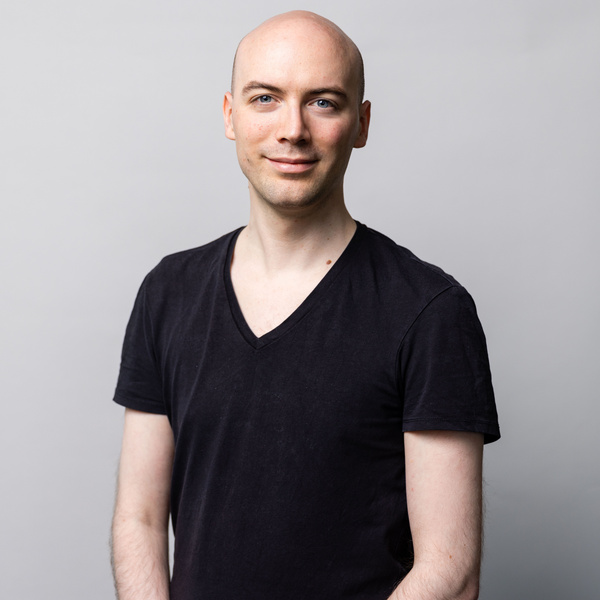
ABSTRACT: A lot of hype has evolved around generative machine learning models lately. Examples such as Stable Diffusion, Midjourney, DALL-E, and ChatGPT have gone viral and are used by many people in their everyday life. Less prominently known but similarly fascinating is the application of these powerful kind of models to the natural sciences. In this talk, we discuss how they can aid in the exploration of novel molecules and materials. To this end, we present the technical and conceptual challenges of molecule generation as well as the promises of advancements in this field, e.g. for renewable energies.
BIOGRAPHY: Niklas Gebauer is a doctoral researcher at the Machine Learning group of Prof. Dr. Klaus-Robert Müller at Technische Universität Berlin. He is a member of the BIFOLD graduate school and part of BASLEARN, the Berlin based joint lab of BASF and TU Berlin for machine learning. His work focuses on generative deep neural networks for molecular structures. He recieved his Master's and Bachelor's degrees in Computer Science from TU Berlin.
Dennis Grinwald
Carbon-aware Machine Learning
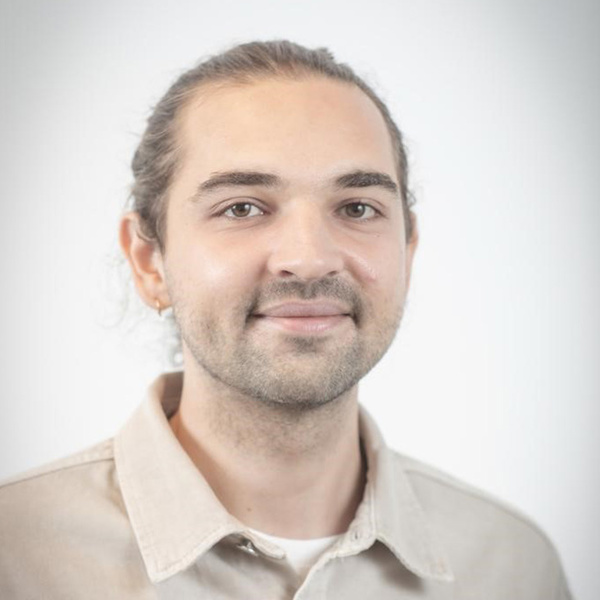
ABSTRACT: Modern machine learning models have revolutionized various fields such as image recognition, natural language processing, and speech recognition. However, training these models requires significant amounts of computational resources and energy, with the latter often coming from fossil fuel sources. The resulting carbon footprint raises growing concerns about the environmental impact of these models. As demand for machine learning models continues to grow, carbon-aware ML training will be critical to the sustainability of this field. In our talk, we will present recent research on carbon-aware and efficient machine learning that aims to mitigate these issues.
BIOGRAPHY: Dennis Grinwald is a PhD student at the Machine Learning Group at TU Berlin and member of the Graduate School at the Berlin Institute of the Foundations of Learning and Data (BIFOLD). His research focuses on efficient and robust ensemble learning on centralised and distributed data.
Dr. Jan Hermann
Machine learning in quantum chemistry
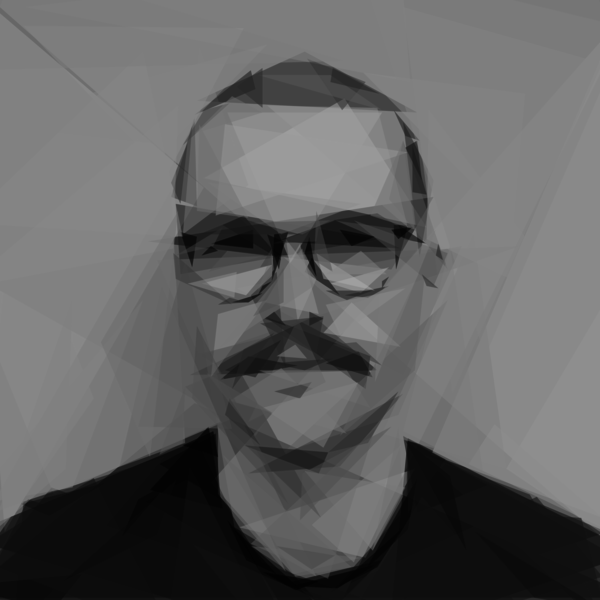
ABSTRACT: Quantum chemistry aims to predict chemical properties of molecules and materials through their simulations according to the laws of quantum mechanics. In the past decade, quantum chemistry has been successfully accelerated in various ways through combining electronic structure calculations with machine learning. There are three main classes of such approaches: Entirely avoiding the electronic-structure problem and learning molecular properties directly from atomic structure, learning to construct effective model Hamiltonians (such as density-functional theory) that make the electronic-structure problem easier to solve, and finally using machine learning to solve the ab-initio electronic Hamiltonian directly. In this introductory lecture, I will review recent efforts in all these three classes of approaches.
BIOGRAPHY: Computational chemist and physicist by training️, who got later hooked on machine learning, and now enjoys integrating deep learning into quantum chemistry. Before joining Microsoft Research AI4Science in Berlin, I was a postdoc and then a junior group leader at FU Berlin, where I worked on deep learning for quantum Monte Carlo and density functional theory. I got my Phd in physics in 2017 while working at the Fritz Haber Institute on modeling of van der Waals interactions in molecules and materials.
WEBSITE: https://jan.hermann.name/
Prof. Marina Höhne
How much can I trust you? Towards Understanding Neural Networks
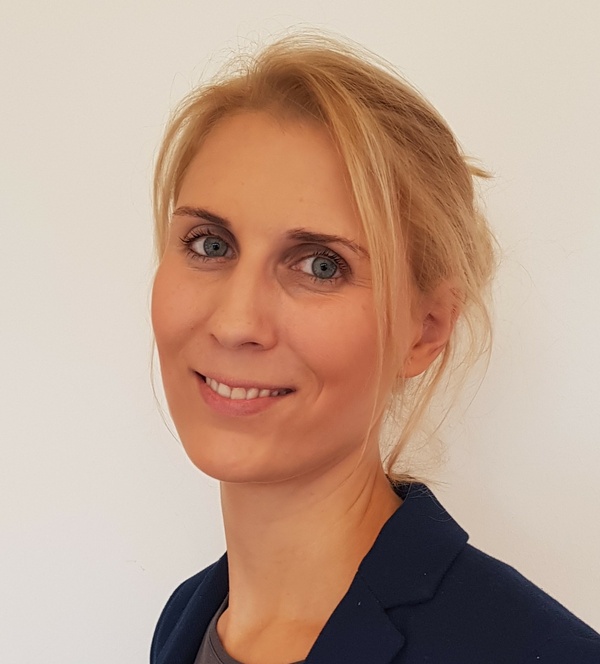
ABSTRACT: In my presentation I will talk about the need to understand the AI models, which are often referred to as black box models. Due to correlations in the training data, the models might have learned artifacts, which lead to undesired behavior and might pose a risk especially in safety-critical applications. I will give a general overview of XAI and present afterwards DORA, a framework to automatically detect neurons that have learned spurious concepts, such as Clever Hans artifacts. Furthermore, I will show how we can improve local and global explanation methods by incorporating the uncertainty knowledge from Bayesian neural networks (BNN) into the explanations and how to quantitatively evaluate your explanations by using Quantus.
BIOGRAPHY: Marina Marie-Claire Höhne is a full professor of the University of Potsdam in the Computer Science faculty and she is the head of the data science department of the Leibniz-Institution for Agriculture and Bioeconomy, including the research group Understandable Machine Intelligence (UMI lab). She received her masters degree in Technomathematics from the Technical University of Berlin in 2012. Afterwards she worked as a researcher at Ottobock in Vienna, Austria, on time series data and domain adaptation for controlling prosthetic devices. In 2014 she started her PhD on explainable AI and received the Dr. rer. nat. degree from the Technical University of Berlin in 2017. After one year maternal leave, she continued working at the machine learning chair at TU Berlin as a postdoctoral researcher. In 2020 she started her own research group UMI Lab dealing with explainable artificial intelligence at the Technical University of Berlin. From 2021-2023 she had a secondary employment as Associate Professor at the Arctic University of Norway. Furthermore, she is a member of the Berlin Institute for Foundations of Learning and Data (BIFOLD), and of the European Laboratory for Learning and Intelligent Systems (ELLIS) Society.
WEBSITE: https://www.atb-potsdam.de/en/about-us/team/staff-members/person/marina-hohne
Prof. Melanie Jaeger-Erben
AI as techno fix? Technological solutionism - and its alternatives
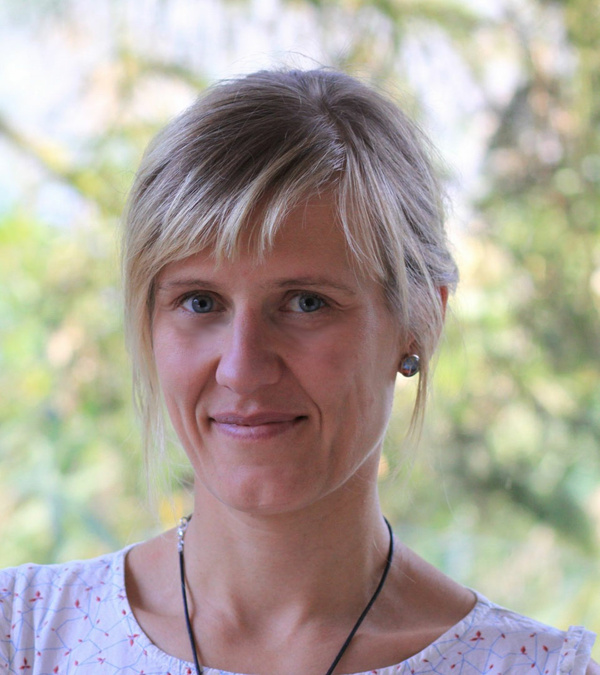
ABSTRACT: There is a paradoxical relationship between technology, particularly Artificial Intelligence (AI), and ecological sustainability. Across political parties with differing ideologies we see a political faith in technology as the ultimate solution to environmental degradation and climate change. This perspective is a holdover from the "technocratic modernity" that started with the industrial revolution and instilled an unwavering belief in technology as the solution to all issues, social or otherwise.
Hence, we want to make everything from production to transportation and energy generation "smarter" and more efficient, ignoring the substantial resource and energy consumption. The damaging effects appear, when technological thinking synergizes with economic interests to suppress non-technological solutions that aim for simplification or even doing without. Technologies like AI are not inherently problematic but become so when designed by entities interested in continuous need amplification.
BIOGRAPHY: Melanie Jaeger-Erben is Professor of Sociology of Technology and the Environment at Brandenburg University of Technology (BTU).
Before 2021, she headed the department of Transdisciplinary Sustainability Research in Electronics at the Technical University Berlin. Her areas of expertise include Social Science Technology Research, Sustainable Consumption and Production Systems, Social Innovation and Social Change, and Qualitative Research Methods, Mixed-Methods, and Participatory Approaches. She earned her Ph.D. in Sociology from the Technical University of Berlin, with a dissertation titled "Between Routine, Reflection, and Transformation – The Change in Everyday Consumption Through Life Events and the Role of Sustainability.". Her academic journey has included working at the Center for Technology and Society at the Technical University of Berlin from 2008 to 2013 and again from 2016 to early 2019.
Between 2013 and 2016, she served as an external consultant for the German Federal Government's Scientific Advisory Board on Global Environmental Change (WBGU).
Jonas Köhler
Getting to Know Generative Models: VAEs, Normalizing Flows, Diffusion Models, and Flow-Matching
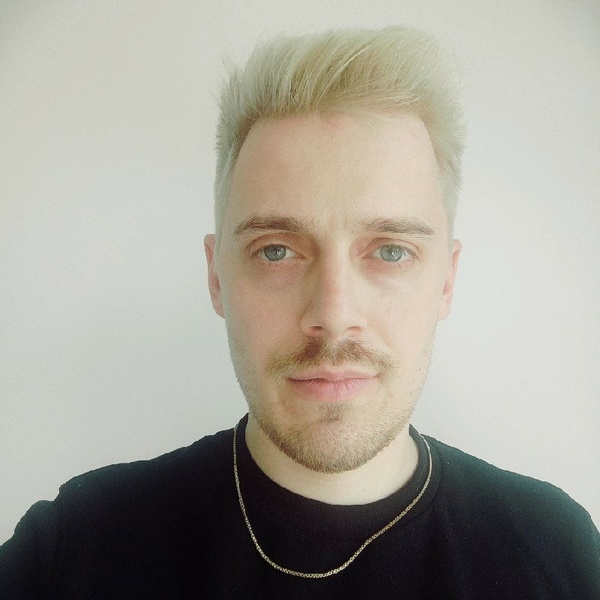
ABSTRACT: In this lecture, we'll take a close look at four types of generative models used in machine learning: Variational Autoencoders (VAEs), Normalizing Flows, Diffusion Models, and Flow-Matching. We'll talk about what each model is good at, and where it might have some downsides. Instead of treating these models as separate things, we'll learn how they're all connected. This will help us get a bigger picture of the world of generative models, making it easier to use the right model for the right job in our future work.
BIOGRAPHY: I'm a senior researcher at Microsoft Research AI4Science Berlin and soon-to-be PhD graduate from Free University of Berlin. My current research focuses on deep learning for electronic structure. Before I worked a lot on generative models for molecular modeling and coarse-graining (during my PhD), differential private generative models as well as equivariant neural networks (during my master). My undergraduate studies combined pure math, applied computer science and sound art at universities of Bonn and Weimar followed by a master in AI at University of Amsterdam. During my studies I interned at Max-Planck-Institute Tuebingen (during my master's) as well as at DeepMind (during my PhD). I am generally interested in generative models, deep learning with hard physical constraints, efficient numerics on scale and using all that to tackle hard pure science problems. I also like any sort of hacky coding especially if it serves aesthetic purposes.
WEBSITE: http://argmin.xyz
Simon Letzgus
XAI for sustainable energy systems
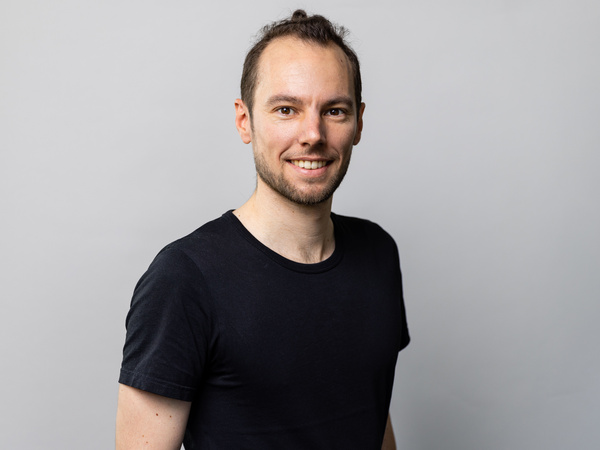
ABSTRACT: Decarbonizing global energy systems represents one of the most challenging task of our generation, playing a crucial role in mitigating the exhaustion of greenhouse gases on a global scale. The complexity of the task is amplified by the utility-scale implementation of sustainable generation units like wind turbines or photovoltaic power plants, which exhibit intermittent generation patterns. Additionally, the electrification of entire sectors such as heat supply and transportation further adds to the complexity of the problem. While machine learning (ML) methods can contribute to addressing these challenges, they often suffer from the perception of being opaque "black-boxes," leading to distrust and hindering their practical application. In this session, we will provide a concise overview of the domain of explainable AI (XAI) and explore how it can foster the development of more sustainable energy systems. Specifically, we will present application cases from the energy sector and highlight the advantages of employing XAI methods in this context.
BIOGRAPHY: Simon Letzgus is a doctoral researcher at the Machine Learning group of Prof. Dr. Klaus-Robert Müller at Technische Universität Berlin. After completing his master's degree in power engineering at Stuttgart University in 2015, he joined Siemens Energy to develop technical solutions related to the challenges of renewable energy systems. Since his return to academia, his research has focused on ML solutions for optimizing, monitoring and understanding complex technical systems with a specific emphasis on XAI for regression models.
WEBSITE: www.linkedin.com/in/simon-letzgus/
Prof. Volker Markl
Welcome Speech
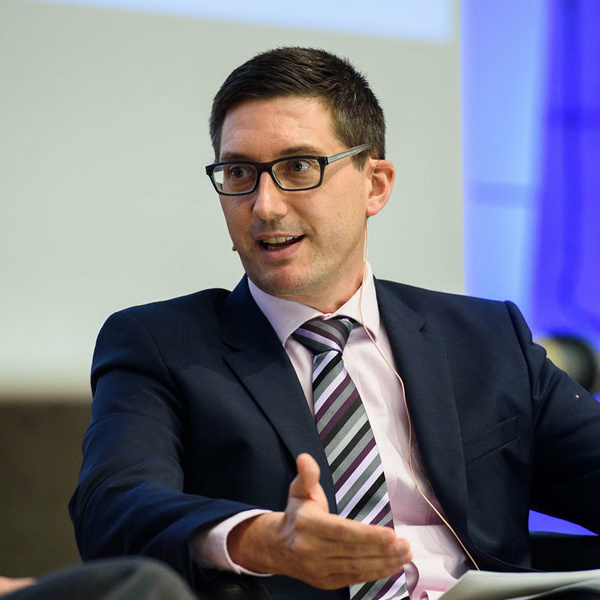
BIOGRAPHY: Volker Markl, Director of the BIFOLD Berlin Insitute, is a German computer scientist, database systems researcher, and a full professor. He leads the Chair of Database Systems and Information Management and is Director of the Berlin Institute for the Foundations of Learning and Data at the Technische Universität Berlin. He is also Chief Scientist and Head of the Intelligent Analytics for Massive Data Research Group at the German Research Center for Artificial Intelligence in Berlin. He has served as the President of the VLDB Endowment and has held an adjunct professorship at the University of Toronto. He currenty serves on the academic advisory council to the Alexander von Humboldt Institute for Internet and Society as well as the scientific advisory board to Software AG. Additionally, he co-chairs the Technological Enablers and Data Science Interdisciplinary Working Group of the ‘Plattform Lernende Systeme,‘ a platform of leading experts who are developing a roadmap for the responsible use of self-learning systems and AI, sponsored by BMBF, the German Federal Ministry of Education and Research. He is a strong proponent of data literacy, systems-oriented research, and computer science education in general.
Prof. Rainer Mühlhoff
Keynote: AI and society: A sociotechnical perspective / Workshop: AI Capitalism: Critique and Regulation
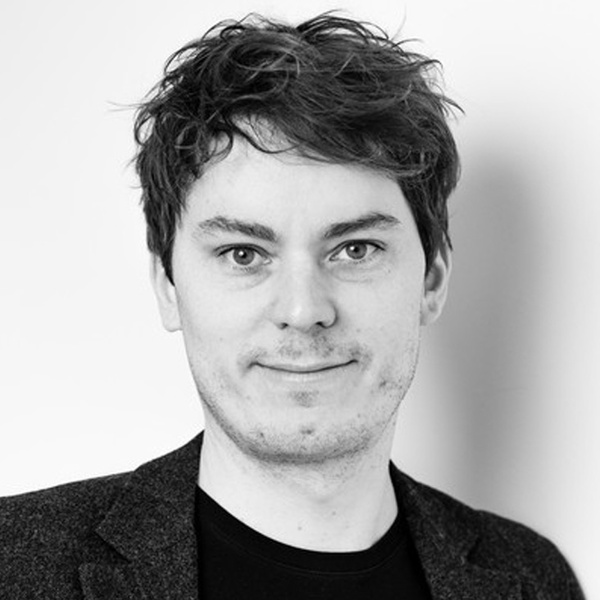
ABSTRACT Keynote: In this keynote, I will tell the story of contemporary AI in a slightly different way: Enabled by new media techniques that capture humans worldwide as data producers, the blossoming of machine learning exemplifies what one could call "Human-Aided AI". Based on this sociotechnical, rather than algorithmic, characterisation of machine learning, I will be able to ask questions of power asymmetries, exploitation and social inequality in relation to contemporary AI - and thus shed some light on the social dimensions of sustainability.
ABSTRACT Workshop: In this two-part workshop, we delve into the intricate relationship between AI and capitalism, viewing AI as a distinctive manifestation of global economic structures. Through a critical lens, we equip participants with analytical tools to identify and dissect the mechanisms of expropriation and power imbalances inherent in AI technology and its implications for sustainability.
In the first part, we will explore the systemic dimensions of AI capitalism, illuminating the dynamics that drive wealth accumulation and social disparities. In the second part, our focus shifts to the regulatory aspect, with an emphasis on data protection as a potential approach to address the widening power asymmetries between data-driven companies and societies.
BIOGRAPHY: Rainer Mühlhoff is leading the Ethics and critical theories of artificial intelligence research group at the Institute of Cognitive Science at the University of Osnabrück. He works on contemporary critical philosophy of the digital world; projects cover the ethics of AI, data protection and Big Data, digital technology and power, digital enlightenment, empowerment and participation.
WEBSITE: rainermuehlhoff.de/en/
Prof. Klaus-Robert Müller
Welcome Speech
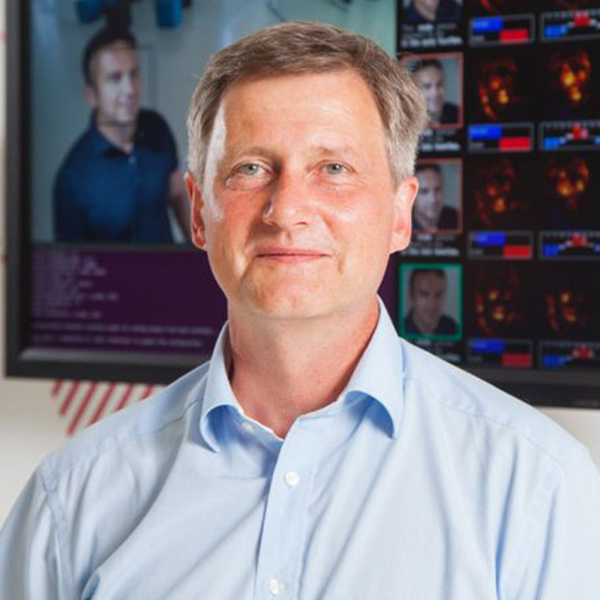
BIOGRAPHY: Klaus-Robert Müller, Director of the BIFOLD Berlin Institute, received the Diploma degree in mathematical physics in 1989 and the Ph.D. in theoretical computer science in 1992, both from University of Karlsruhe, Germany. From 1992 to 1994 he worked as a Postdoctoral fellow at GMD FIRST, in Berlin where he started to built up the intelligent data analysis (IDA) group. From 1994 to 1995 he was a European Community STP Research Fellow at University of Tokyo in Prof. Amari’s Lab. From 1995 until 2008 he was head of department of the IDA group at GMD FIRST (since 2001 Fraunhofer FIRST) in Berlin and since 1999 he holds a joint associate Professor position of GMD and University of Potsdam. In 2003 he became a full professor at University of Potsdam, in 2006 he became chair of the machine learning department at TU Berlin. He has been lecturing at Humboldt University, Technical University Berlin and University of Potsdam. In 1999 he received the annual national prize for pattern recognition (Olympus Prize) awarded by the German pattern recognition society DAGM, in 2006 the SEL Alcatel communication award and in 2014 he was granted the Science Prize of Berlin awarded by the Governing Mayor of Berlin and in 2017 he received the Vodafone Innovations Award. Since 2012 he is Member of the German National Academy of Sciences Leopoldina and he holds a distinguished professorship at Korea University in Seoul. In 2017 he was elected member of the Berlin Brandenburg Academy of Sciences and also external scientific member of the Max Planck Society. For 5 years he was director of the Bernstein Center for Neurotechnology, from 2014 he became co-director of the Berlin Big Data Center (BBDC) and from 2018 simultaneously director of the Berlin Center for Machine Learning (BZML).
MORE: www.bifold.berlin/people/prof-dr-klaus-robert-mueller.html
Tabea Rößner, MdB
A political perspecitve on Artificial Intelligence and Sustainability
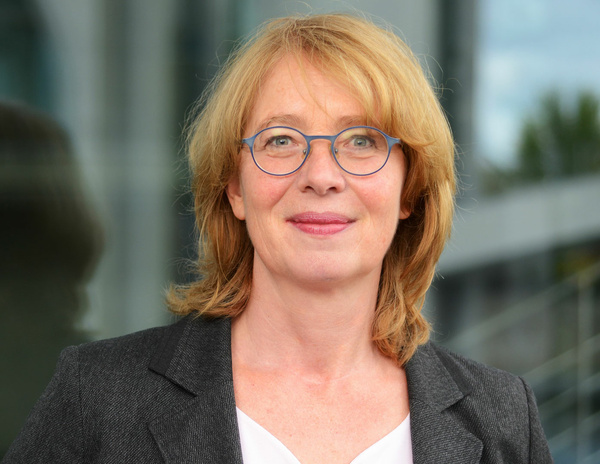
ABSTRACT: The topic of Artificial Intelligence and Sustainability has reached the political sphere long since. Discussions about benefits, risks and the need for regulation take place regularly. However, AI capabilities are evolving and new use cases are coming up, but ecological impacts are also becoming clearer. In this talk, Tabea Rößner will give an insight into how the AI & sustainability discourse takes shape in the German and European political process, what is being planned politically, what are political obstacles and what she considers as open questions for researchers to tackle.
BIOGRAPHY: Tabea Rößner is a member of the the German Bundestag (Green Party). She is Chairwoman of the Committee for Digital Affairs and a member of the Committee for the Environment, Nature Conservation, Nuclear Safety and Consumer Protection.
Friederike Rohde
Sustainable AI and the impacts of socio-technical AI systems
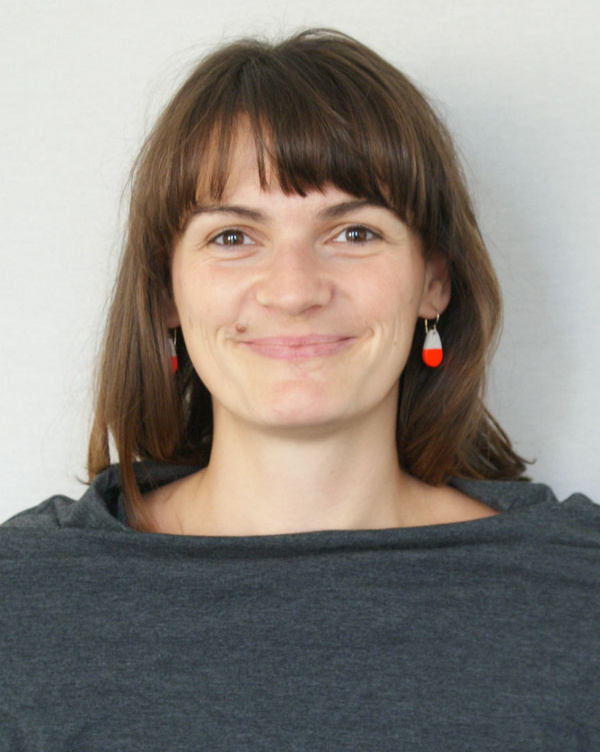
ABSTRACT: The expanded use of AI systems is associated with multi-faceted societal, environmental, and economic consequences.
These include non-transparent decision-making processes, discrimination, increasing inequalities, rising energy consumption and greenhouse gas emissions in AI model development and application, and an increasing concentration of economic power. By considering the multi-dimensionality of sustainability, the presented interdisciplinary approach contributes a holistic perspective on sustainable AI and suggests a set of sustainability criteria and indicators to support the conscious development and application of AI systems.
BIOGRAPHY: Friederike Rohde (Dipl. Soz. tec.) is a researcher in Science & Technology Studies, focusing on the dynamics of socio-technical change processes. She is concerned with making digital change sustainable and is interested in transformative social innovation approaches. Currently, she is a Researcher at the Institute for Ecological Economy Research (IÖW), where she works on narratives and future visions on digitalisation, such as Smart Grids and Smart Cities. In the SustAIn project, she critically examines the interrelations between AI and sustainability, and analyses the expectations and promises of AI concerning sustainability. As a spokesperson for the Bündnis digitale Stadt (Digital City Alliance), she is engaged in promoting a democratic, inclusive digital policy for Berlin that serves public interests.
Prof. Wojciech Samek
Concept-Level Explainable AI
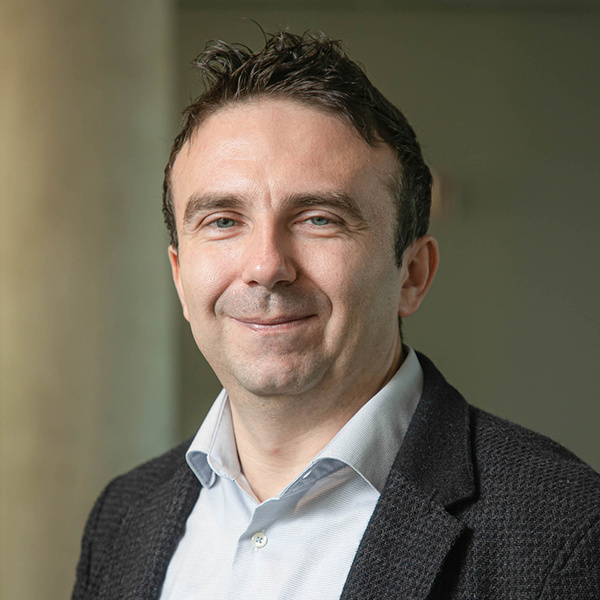
ABSTRACT: The emerging field of Explainable AI (XAI) aims to bring transparency to today's powerful but opaque deep learning models. This talk will present Concept Relevance Propagation (CRP), a next-generation XAI technique which explains individual predictions in terms of localized and human-understandable concepts. Other than the related state-of-the-art, CRP not only identifies the relevant input dimensions (e.g., pixels in an image) but also provides deep insights into the model’s representation and the reasoning process. This makes CRP a perfect tool for AI-supported knowledge discovery in the sciences. In the talk we will demonstrate on multiple datasets, model architectures and application domains, that CRP-based analyses allow one to (1) gain insights into the representation and composition of concepts in the model as well as quantitatively investigate their role in prediction, (2) identify and counteract Clever Hans filters focusing on spurious correlations in the data, and (3) analyze whole concept subspaces and their contributions to fine-grained decision making. By lifting XAI to the concept level, CRP opens up a new way to analyze, debug and interact with ML models, which is of particular interest in safety-critical applications and the sciences.
BIOGRAPHY: Wojciech Samek is a professor in the Department of Electrical Engineering and Computer Science at the Technical University of Berlin and is jointly heading the Department of Artificial Intelligence at Fraunhofer Heinrich Hertz Institute (HHI), Berlin, Germany. He studied computer science at Humboldt University of Berlin, Heriot-Watt University and University of Edinburgh and received the Dr. rer. nat. degree with distinction (summa cum laude) from the Technical University of Berlin in 2014. During his studies he was awarded scholarships from the German Academic Scholarship Foundation and the DFG Research Training Group GRK 1589/1, and was a visiting researcher at NASA Ames Research Center, Mountain View, USA. Dr. Samek is associated faculty at the BIFOLD - Berlin Institute for the Foundation of Learning and Data, the ELLIS Unit Berlin, the DFG Research Unit DeSBi, and the DFG Graduate School BIOQIC, and member of the scientific advisory board of IDEAS NCBR - Polish Centre of Innovation in the Field of Artificial Intelligence. Furthermore, he is a senior editor of IEEE TNNLS, an editorial board member of Pattern Recognition, and an elected member of the IEEE MLSP Technical Committee and the Germany's Platform for Artificial Intelligence. He is recipient of multiple best paper awards, including the 2020 Pattern Recognition Best Paper Award and the 2022 Digital Signal Processing Best Paper Prize, and part of the expert group developing the ISO/IEC MPEG-17 NNC standard. He is the leading editor of the Springer book "Explainable AI: Interpreting, Explaining and Visualizing Deep Learning" (2019), co-editor of the open access Springer book “xxAI – Beyond explainable AI” (2022), and organizer of various special sessions, workshops and tutorials on topics such as explainable AI, neural network compression, and federated learning. Dr. Samek has co-authored more than 150 peer-reviewed journal and conference papers; some of them listed as ESI Hot (top 0.1%) or Highly Cited Papers (top 1%).
WEBSITE: https://iphome.hhi.de/samek
Prof. Sebastian Schelter
Directions Towards Resource-Efficient Machine Learning Systems in e-Commerce
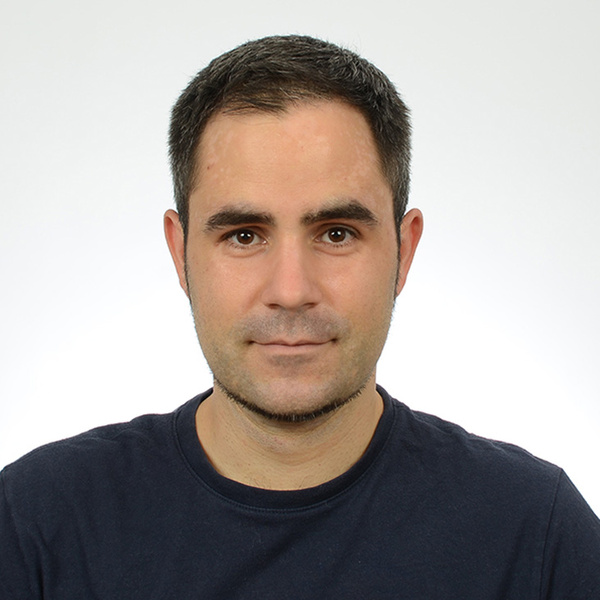
ABSTRACT: Real-world ML applications are still difficult to develop and extremely resource-intensive to operate, despite recent breath-taking scientific advances. In this talk, I will share some of our ongoing research on building resource-efficient ML applications for large-scale e-Commerce scenarios. In the first part of the talk, I will detail how we designed and implemented ''Serenade'', a kNN-based recommender system running in production at bol.com, a large e-commerce platform in the Netherlands with 13 million customers and a catalog containing several million products. Serenade is resource-efficent by design and cheap to operate: the system currently serves up to 2,000 requests per second with a p90 latency below ten milliseconds from two CPU cores only. In the second part of the talk, I will discuss some of our ongoing research on reducing the operational costs for deploying large language models (LLMs) for data wrangling and question answering tasks. In particular, we aim to enable the re-use small off-the-shelf models across a wide variety of tasks and datasets, while enhancing their performance with techniques like learned prompts, retrieval-augmentation or data pruning.
BIOGRAPHY: Sebastian Schelter is an Assistant Professor with the University of Amsterdam, where he conducts research at the intersection of data management and machine learning. His work addresses data-related problems that occur in the real world application of machine learning. Examples are scalable data quality validation, data debugging for machine learning pipelines, or enforcing the “right-to-be-forgotten” in deployed machine learning applications. Sebastian's research is accompanied by efficient and scalable open source implementations, many of which are applied in real world use cases, for example in the SageMaker Model Monitor service and the Glue Data Integration service of the Amazon Web Services cloud, the product recommendation system at bol.com and large-scale recommendation libraries in Microsoft Azure. His research contributions have been recognized with an ACM SIGMOD Systems Award, an ACM SIGMOD Best Demo Runner Up Award, a Best Paper Runner Up Award from the Table Representation Learning workshop at NeurIPS and an Amazon Education Research Grant Award. In the past, he has been a Faculty Fellow with the Center for Data Science at New York University and a Senior Applied Scientist at Amazon Research, after obtaining his PhD at the database group of TU Berlin with Volker Markl. He has been active in open source as an elected member of the Apache Software Foundation, and has extensive experience in building real world systems from his time at Amazon, Twitter, IBM Research and Zalando.
WEBSITE: https://ssc.io
Paul Schütze
AI Capitalism: Critique and Regulation
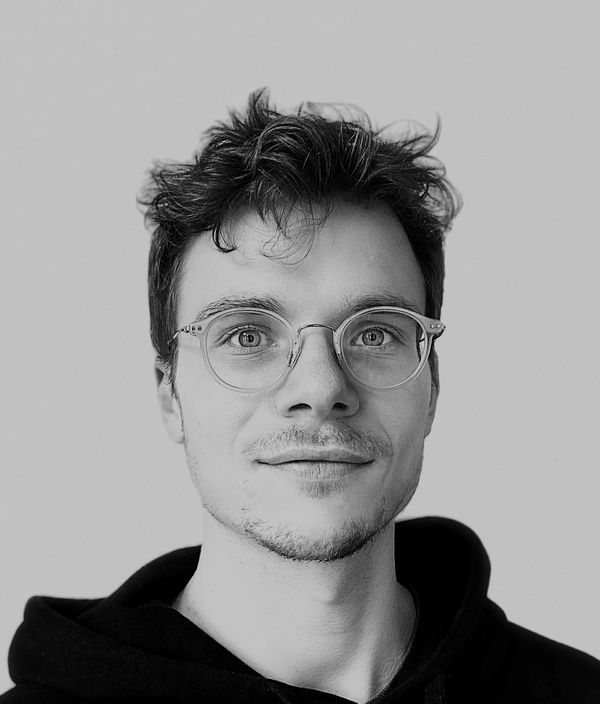
ABSTRACT: In this two-part workshop, we delve into the intricate relationship between AI and capitalism, viewing AI as a distinctive manifestation of global economic structures. Through a critical lens, we equip participants with analytical tools to identify and dissect the mechanisms of expropriation and power imbalances inherent in AI technology and its implications for sustainability.
In the first part, we will explore the systemic dimensions of AI capitalism, illuminating the dynamics that drive wealth accumulation and social disparities. In the second part, our focus shifts to the regulatory aspect, with an emphasis on data protection as a potential approach to address the widening power asymmetries between data-driven companies and societies.
BIOGRAPHY: Paul is a research assistant in the Ethics and Critical Theories of Artificial Intelligence research group at the University of Osnabrück. In his current research he focuses on the structural effects, power relations and underlying social and material conditions of AI. Especially, he works on the socio-economic dynamics that surround AI technologies and how these influence society’s relation to the climate crisis. He has a background in critical social philosophy, affect studies, a political philosophy of mind and cognitive science.
Prof. Pinar Tözün
The Different Scales of Resource-Aware ML & How to Tackle Them
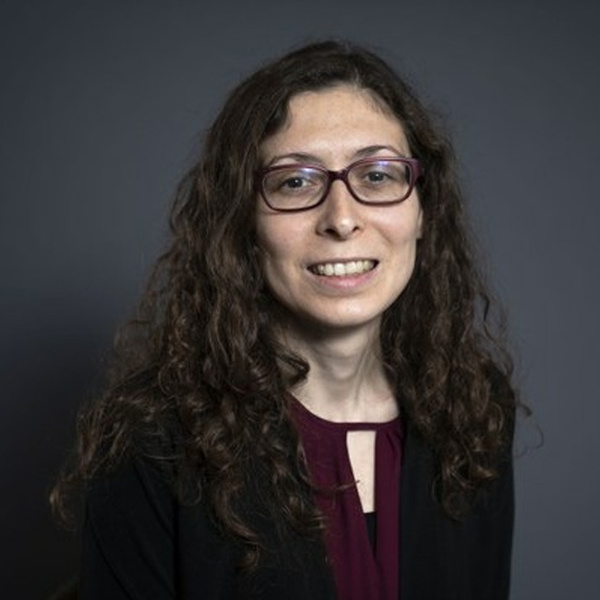
ABSTRACT: Today, machine learning (ML) runs at various scales of hardware resources from the cloud and high-performance computing (HPC) centers to edge and Internet-of-Things (IoT) devices. To achieve resource-aware machine learning, we must understand the needs and challenges of ML applications at these different scales. In this talk, we will first investigate ways of improving hardware utilization on modern and powerful CPU-GPU co-processors, which serve as the commodity hardware for ML in the cloud and HPC, using workload collocation. Then, we will investigate performance and power trade-offs for ML-based image analysis in space using resource-constrained edge/IoT devices.
BIOGRAPHY: Pınar Tözün is an Associate Professor at IT University of Copenhagen. Before ITU, she was a research staff member at IBM Almaden Research Center. Prior to joining IBM, she received her PhD from EPFL. Her thesis received ACM SIGMOD Jim Gray Doctoral Dissertation Award Honorable Mention in 2016. Her research focuses on hardware-conscious machine learning, performance characterization of data-intensive systems, and scalability and efficiency of data-intensive systems on modern hardware.
WEBSITE: www.pinartozun.com
Dr. Stefan Ullrich
Conscience Bytes - ethical case studies in computer science
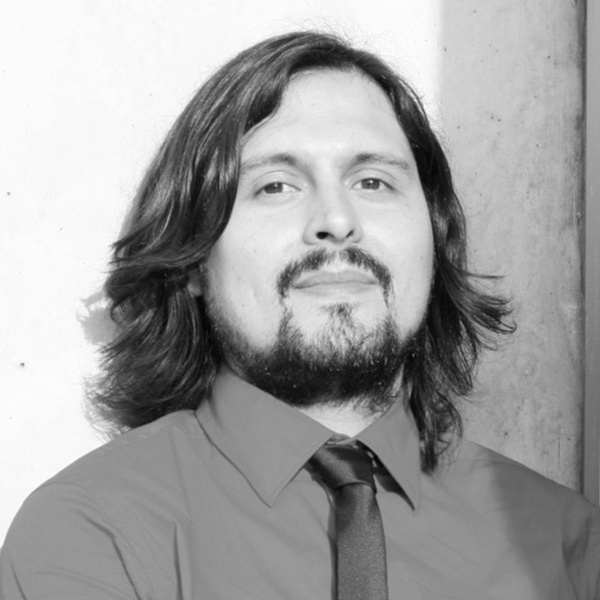
ABSTRACT: Critical thinking has become more important than ever in the age of large ML models and Big Data. Its socio-technical context must be understood in order to develop common good oriented IT systems. In the workshop, we want to make use of collaborative public reasoning on this topic.
BIOGRAPHY: Stefan Ullrich is an Informatician with a minor degree in Philosophy who is researching on the impact of ubiquitous information technology systems on society. He is co-Chair of the Commission for Ethics in Research at the Technical University Berlin.
Website: www.weizenbaum-institut.de/en/spezialseiten/persons-details/p/stefan-ullrich/
Marcus Voss
Artificial Intelligence and Planetary Boundaries - How can we use AI to improve environmental sustainability?
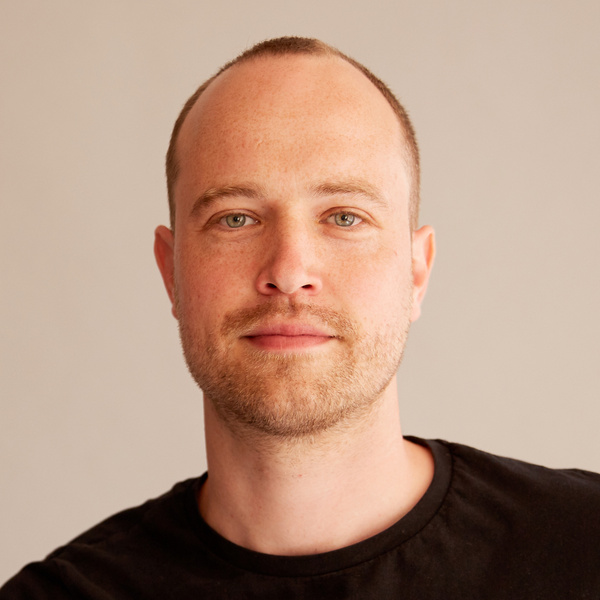
ABSTRACT: Artificial intelligence and machine learning provide powerful tools for effective climate action and improving environmental sustainability. In various applications, such as energy, buildings, or transportation, they can help mitigate climate change by reducing greenhouse gas emissions. They can help adapt to a changing environment, monitor and slow the loss of biodiversity, and support the efficient use of resources. However, AI and ML are not a silver bullet and can only be part of the solution. This talk will provide an overview of the strengths and weaknesses of ML, some examples of applications and recurring themes, as well as trends in research and application in industry. Examples will be given from the Quantified Trees project on the use of AI for street tree irrigation in Berlin and from the BiGEye project where AI is used as part of a digital vegetation management to support the phase-out of glyphosate.
BIOGRAPHY: Marcus Voss is an AI expert at Birds on Mars, where he is responsible for AI applications for environmental sustainability. He is on the Board of Directors of Climate Change AI, an international NPO catalyzing impactful work at the intersection of climate change and machine learning. Marcus has been an external lecturer on AI and data science at TU Berlin, Leuphana University Lüneburg, and CODE University. Previously, Marcus was a Research Associate at the TU Berlin, where he led the Smart Energy Systems research group of the DAI Lab, working on AI applications in the smart grid and the sustainable development of AI systems.
WEBSITE: www.marcusvoss.com
Philipp Wiesner
Carbon-aware Machine Learning
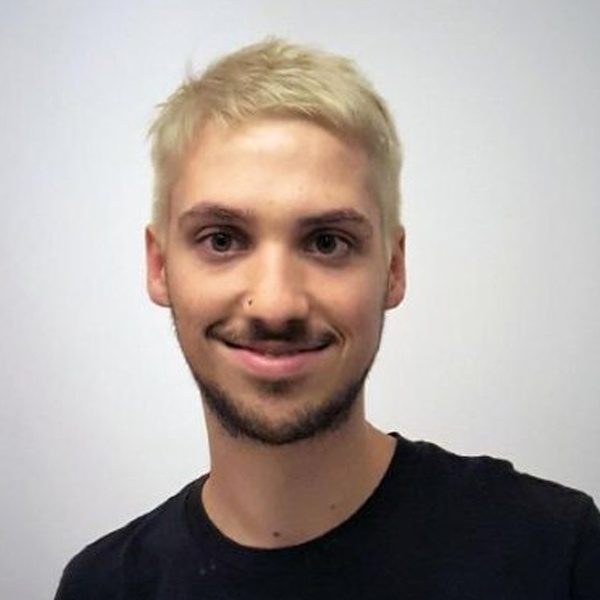
ABSTRACT: Modern machine learning models have revolutionized various fields such as image recognition, natural language processing, and speech recognition. However, training these models requires significant amounts of computational resources and energy, with the latter often coming from fossil fuel sources. The resulting carbon footprint raises growing concerns about the environmental impact of these models. As demand for machine learning models continues to grow, carbon-aware ML training will be critical to the sustainability of this field. In our talk, we will present recent research on carbon-aware and efficient machine learning that aims to mitigate these issues.
BIOGRAPHY: Philipp Wiesner is a research associate and third year PhD student at TU Berlin, working in the research group on Distributed and Operating Systems (DOS) by Prof. Odej Kao. Before that, I’ve worked as a Software and Data Engineer at different companies in Berlin. My research focus is on carbon-aware computing: Aligning the power consumption of computing systems with the availability of renewable energy. Besides that, I’m interested in research on systems for machine learning as well as the co-simulation and testing of computing and energy systems.
WEBSITE: https://philippwiesner.org/
Prof. Herbert Zech
Welcome Speech
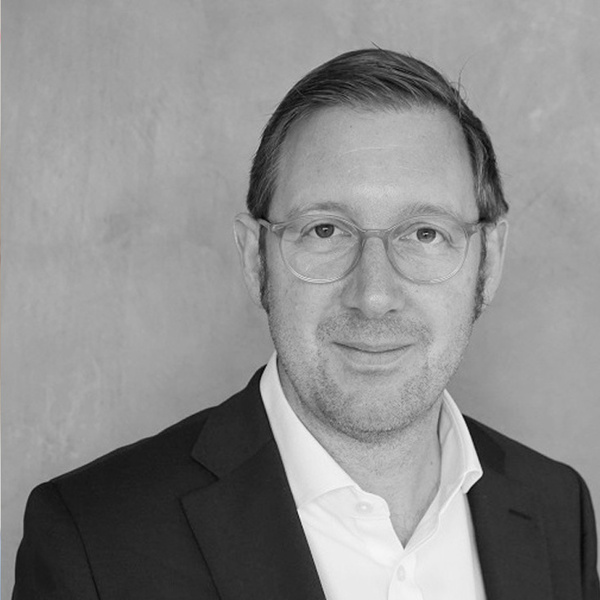
BIOGRAPHY: Herbert Zech, Director of the Weizenbaum Institute, habilitated in Bayreuth. From 2012, he worked at the University of Basel, initially as Extraordinarius for Private Law with a focus on Life Sciences Law, and since 2015 as Full Professor for Life Sciences Law and Intellectual Property Law. In April 2019, he moved to the Humboldt University of Berlin to the Chair of Civil Law, Technology and IT Law. The professorship is linked to the position of director at the Weizenbaum Institute for the Networked Society. Herbert Zech will be affiliated with the Institute for Computer Science of the Faculty of Mathematics and Natural Sciences in the form of a secondary membership. Herbert Zech also studied biology after his bar exam. Following on from this, he is particularly interested in the connection between the natural sciences and law. His main areas of expertise are technology law and intellectual property law. He is currently working on legal problems of Big Data and Artificial Intelligence, among others.
MORE: www.weizenbaum-institut.de/en/spezialseiten/persons-details/p/herbert-zech/
Dr. Steffen Zeuch
NebulaStream: a general purpose, end-to-end data management system for the IoT
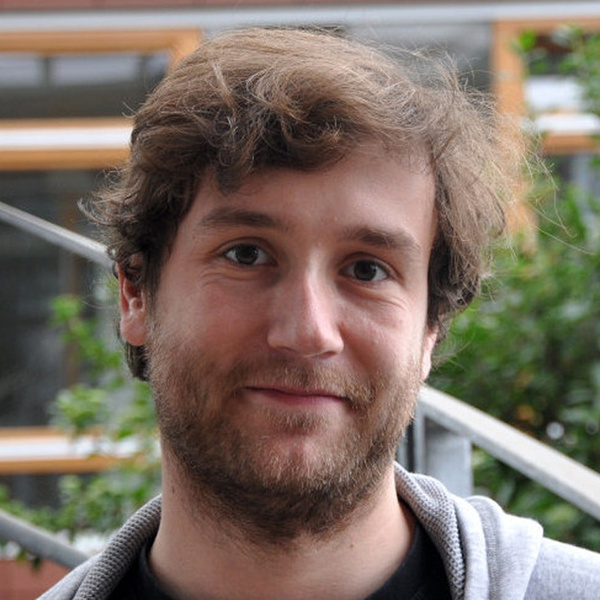
ABSTRACT: In this talk, Steffen Zeuch will first present his past research activities in the fields of modern hardware, stream processing, and IoT data management. After that, he will outline his research vision of a general-purpose data management system (NebulaStream) that is capable to cope with and exploit recent changes in data characteristics, workloads, hardware capabilities, as well as computing infrastructures. With NebulaStream, we envision a platform to enable researchers and practitioners to develop and test their algorithms and approaches in the context of future IoT environments. On top of this platform, researchers from different domains like Machine Learning, Signal Processing, Complex-Event Processing, or Spatial Processing could implement their approaches. One possible direction for future research is to combine different parts of data science, e.g., data analytics, data mining, or machine learning, in one system to maximize sharing potential, utilize new optimization potential, and provide users with a unified view of their data.
BIOGRAPHY: Steffen Zeuch is a Senior Researcher at the DIMA group (TU Berlin) and IAM group (DFKI). He received his Ph.D. in Computer Science at Humboldt University Berlin in the research group of Prof. Freytag. He is conducting research in data management, with an emphasis on topics related to modern hardware, distributed systems, and IoT environments. He has published research papers on query optimization and execution as well as on novel system architectures in many top-tier conferences. Currently, he is the project lead of the NebulaStream (www.nebula.stream) project that builds a novel open-source stream data management system, which combines the cloud, the fog, and the sensors in a single unified platform and provides a holistic view for processing distributed fast data. Within BIFOLD (https://bifold.berlin/de/), the Berlin Institute for the Foundations of Learning and Data, he is leading the IoT-Lab. The mission of this lab is to research stream data management for the Internet of Things, in order to enable users to execute data-driven analytics applications in a heavily distributed environment of sensors and processing devices.