This project studies the Wasserstein distance in the broad context of deep learning, with a focus on cases where the data is affected by quality issues. The project investigates how the Wasserstein distance can be used to build more robust deep learning models with more homogeneous prediction performance across data subgroups. The project also addresses the question of how to meaningfully derive transport maps from the Wasserstein distance when the available data is unrepresentative of the true data distribution or contains artifacts.
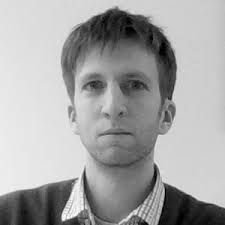
Research Junior Group Lead
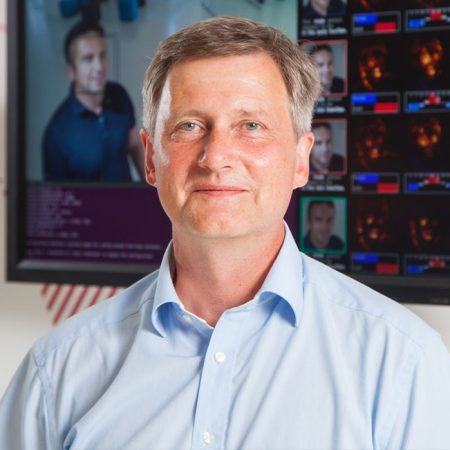
Director
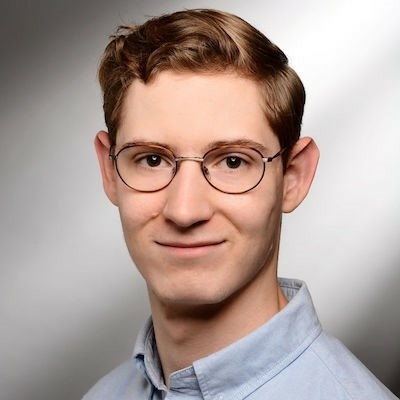
Doctoral Researcher