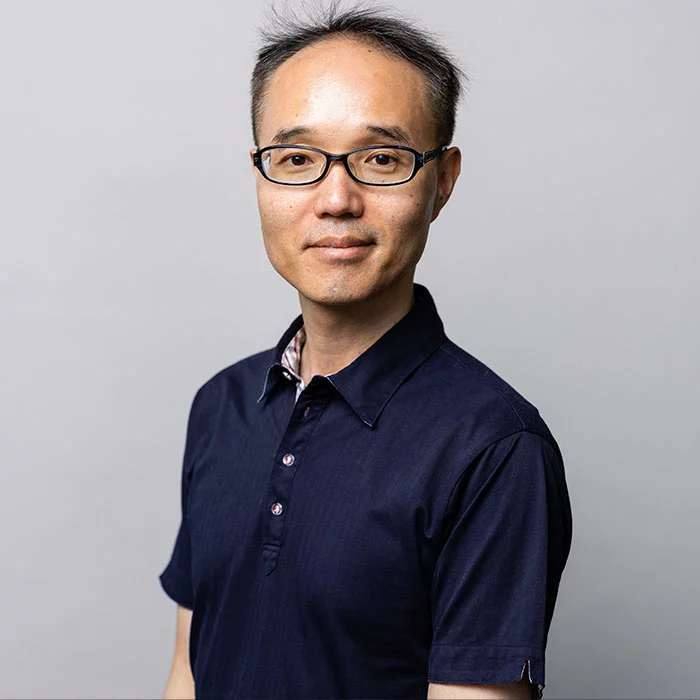
PhD Shinichi Nakajima
Research Group Lead
Dr. Shinichi Nakajima is a member of Machine Learning Group in Technische Universität Berlin. He received the master degree on physics in 1995 from Kobe university, and worked with Nikon Corporation until September 2014 on statistical analysis, image processing, and machine learning. He received the doctoral degree on computer science in 2006 from Tokyo Institute of Technology. His research interest is in theory and applications of machine learning, in particular, Bayesian inference, generative modeling, uncertainty estimation, explainable artificial intelligence, and their applications for computer vision, natural language processing, science and quantum computing. At the BIFOLD he leads the research group "Probabilistic Modeling and Inference".
Khaled Kahouli, Stefaan Simon Pierre Hessmann, Klaus-Robert Müller, Shinichi Nakajima, Stefan Gugler, Niklas Wolf Andreas Gebauer
Molecular relaxation by reverse diffusion with time step prediction
Dennis Grinwald, Philipp Wiesner, Shinichi Nakajima
Solution Simplex Clustering for Heterogeneous Federated Learning
Kim Andrea Nicoli, Christopher Anders, Lena Funcke, Karl Jansen, Shinichi Nakajima
NeuLat: a toolbox for neural samplers in lattice field theories
Kim A. Nicoli, Christopher J. Anders, Tobias Hartung, Karl Jansen, Pan Kessel, Shinichi Nakajima
Detecting and Mitigating Mode-Collapse for Flow-based Sampling of Lattice Field Theories
Kirill Bykov, Laura Kopf, Shinichi Nakajima, Marius Kloft, Marina M.-C. Höhne